PolarMOT: How Far Can Geometric Relations Take us in 3D Multi-object Tracking?
European Conference on Computer Vision(2022)
摘要
Most (3D) multi-object tracking methods rely on appearance-based cues for data association. By contrast, we investigate how far we can get by only encoding geometric relationships between objects in 3D space as cues for data-driven data association. We encode 3D detections as nodes in a graph, where spatial and temporal pairwise relations among objects are encoded via localized polar coordinates on graph edges. This representation makes our geometric relations invariant to global transformations and smooth trajectory changes, especially under non-holonomic motion. This allows our graph neural network to learn to effectively encode temporal and spatial interactions and fully leverage contextual and motion cues to obtain final scene interpretation by posing data association as edge classification. We establish a new state-of-the-art on nuScenes dataset and, more importantly, show that our method, PolarMOT, generalizes remarkably well across different locations (Boston, Singapore, Karlsruhe) and datasets (nuScenes and KITTI).
更多查看译文
关键词
3D multi-object tracking, Graph neural networks, Lidar scene understanding
AI 理解论文
溯源树
样例
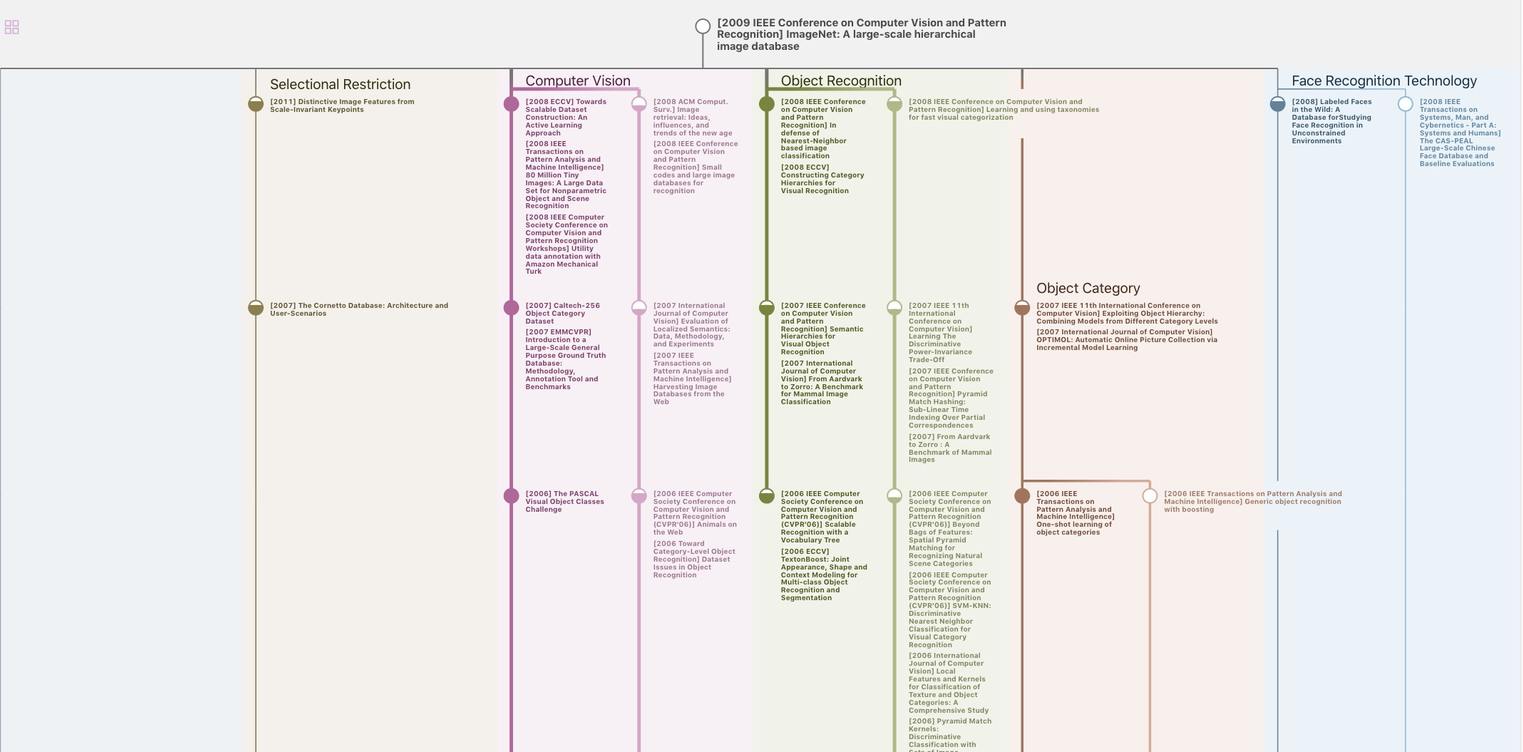
生成溯源树,研究论文发展脉络
Chat Paper
正在生成论文摘要