A Stochastic Maximum Principle Approach for Reinforcement Learning with Parameterized Environment.
Journal of Computational Physics(2023)
摘要
In this work, we introduce a stochastic maximum principle (SMP) approach for solving the reinforcement learning problem with the assumption that the unknowns in the environment can be parameterized based on physics knowledge. For the development of numerical algorithms, we apply an effective online parameter estimation method as our exploration technique to estimate the environment parameter during the training procedure, and the exploitation for the optimal policy is achieved by an efficient backward action learning method for policy improvement under the SMP framework. Numerical experiments are presented to demonstrate that the SMP approach for reinforcement learning can produce reliable control policy, and the gradient descent type optimization in the SMP solver requires less training episodes compared with the standard dynamic programming principle based methods.
更多查看译文
关键词
Reinforcement learning,Optimal control,Stochastic maximum principle,Parameter estimation,Optimal filtering
AI 理解论文
溯源树
样例
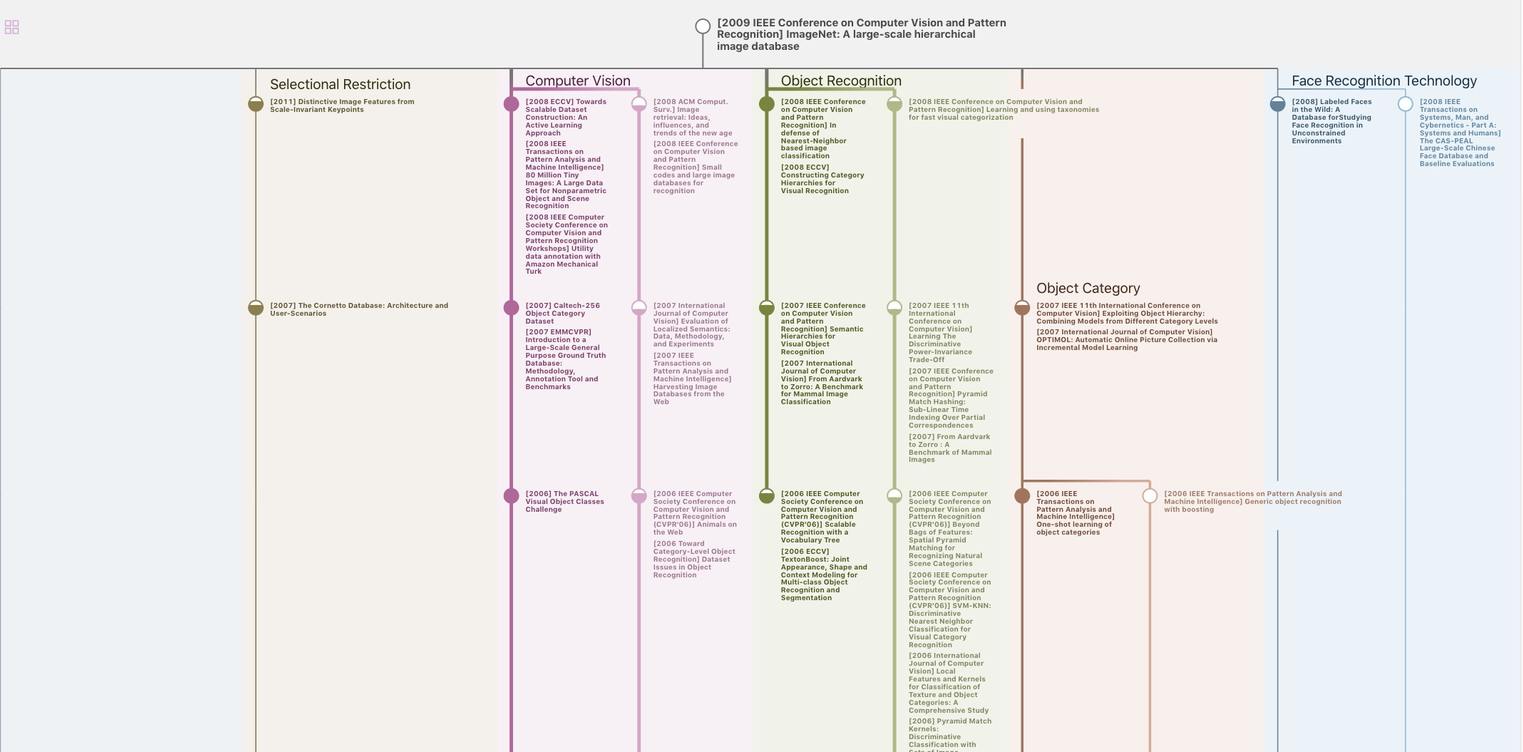
生成溯源树,研究论文发展脉络
Chat Paper
正在生成论文摘要