Accelerating super-resolution ultrasound localization microscopy using generative adversarial nete
Acta Physica Sinica(2022)
摘要
Ultrafast ultrasound localization microscopy (uULM) has broken through the fundamental acoustic diffraction limit by accumulating thousands of sub-wavelength microbubble localisation points and improved the spatial resolution by more than one order of magnitude, which is conducive to clinical diagnosis. By localizing individually injected microbubbles and tracking their movement with a subwavelength resolution, the vasculature microscopy can be achieved with micrometer scale. However, the reconstruction of a uULM image often requires tens or even hundreds of seconds of continuous long-range image acquisition, which limits its clinical application. In order to solve this problem, a generative adversarial network (GAN) based deep learning method is proposed to reconstruct the super-resolution ultrasound localization microscopy. In vivo uULM ultrasound datasets are used to train the network to reconstruct dense vascular networks via localized microbubbles. This approach is validated by using another in-vivo dataset obtained in a rat brain. Results show that GAN based ultrafast ultrasound localization microscopy (GAN-uULM) can resolve micro vessels smaller than 10 mu m. Besides, GAN-uULM is able to distinguish small vessels that cannot be continuously reconstructed by using a standard uULM reconstruction method. Saturation parameter based on counting the number of explored pixels is used to evaluate the reconstruction quality. The proposed reconstruction approach reduces the data requirement by half and thus significantly accelerates the uULM imaging. It is illustrasted that for a dataset of 292 s ultrafast acquisition, the saturation of standard uULM image is 33%, while that of GAN-uULM can reach 46%. Fourier ring correlation (FRC) method is utilized to measure the spatial resolution in uULM. Resolutions of the images obtained by standard uULM and GAN-ULM are 7.8 mu m and 8.9 mu m, respectively. In conclusion, the developed deep learning model is able to connect trajectories with less computational complexity and avoids manual tuning and trajectory screening, providing an effective solution for accelerating ultrasound localization microscopy.
更多查看译文
关键词
super-resolution, ultrasonic localization microscopy, convolutional neural network, generative, adversarial network
AI 理解论文
溯源树
样例
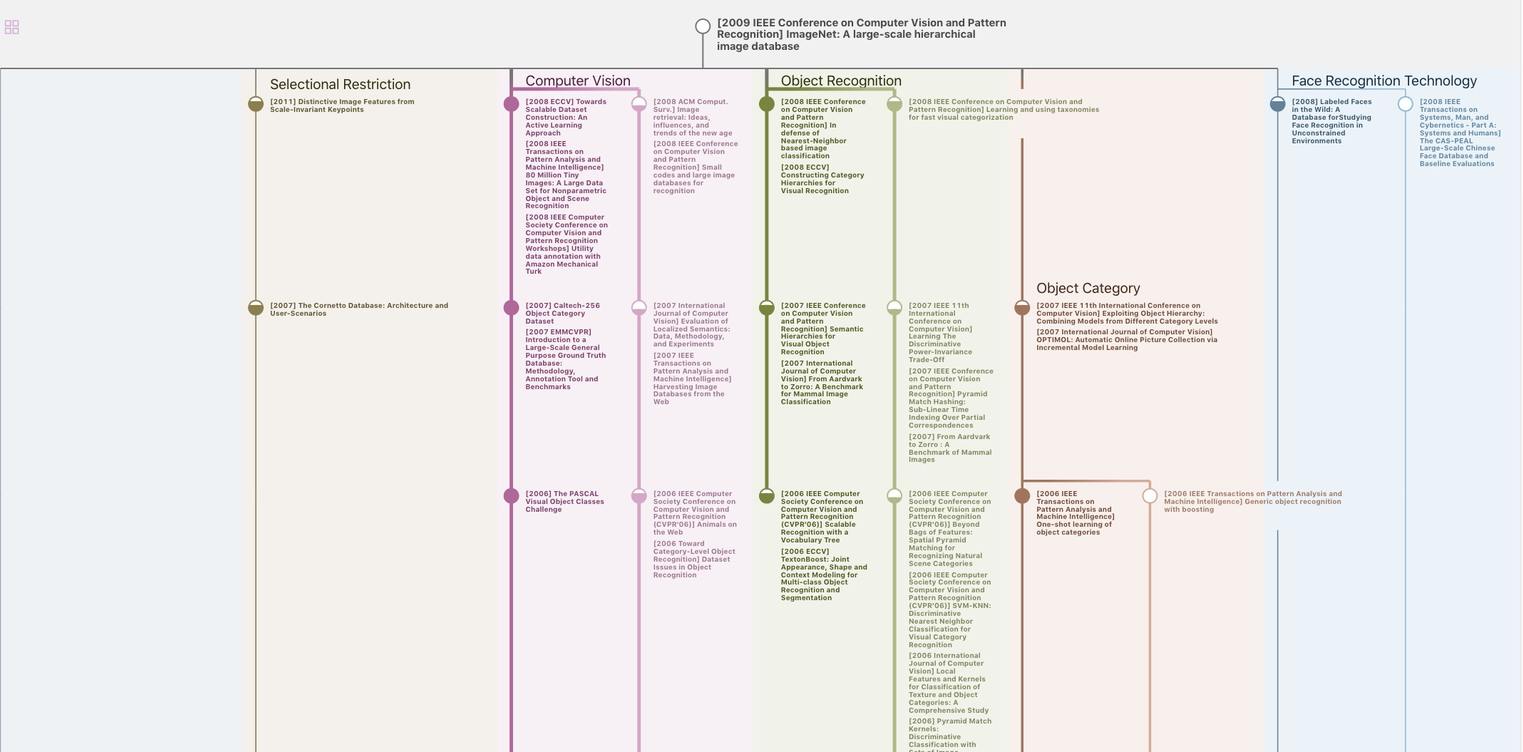
生成溯源树,研究论文发展脉络
Chat Paper
正在生成论文摘要