Low Complexity Binarized 2D-CNN Classifier for Wearable Edge AI Devices
IEEE Transactions on Biomedical Circuits and Systems(2022)
摘要
Wearable Artificial Intelligence-of-Things (AIoT) devices exhibit the need to be resource and energy-efficient. In this paper, we introduced a quantized multilayer perceptron (qMLP) for converting ECG signals to binary image, which can be combined with binary convolutional neural network (bCNN) for classification. We deploy our model into a low-power and low-resource field programmable gate array (FPGA) fabric. The model requires 5.8× lesser multiply and accumulate (MAC) operations than known wearable CNN models. Our model also achieves a classification accuracy of 98.5%, sensitivity of 85.4%, specificity of 99.5%, precision of 93.3%, and F1-score of 89.2%, along with dynamic power dissipation of 34.9 μW.
更多查看译文
关键词
Artificial intelligence-of-things,co-design,convolutional neural network,ECG,field programmable gate array,inference,low-power design,multi-layer perceptron,multi-tasking,reuse,state machine,wearable
AI 理解论文
溯源树
样例
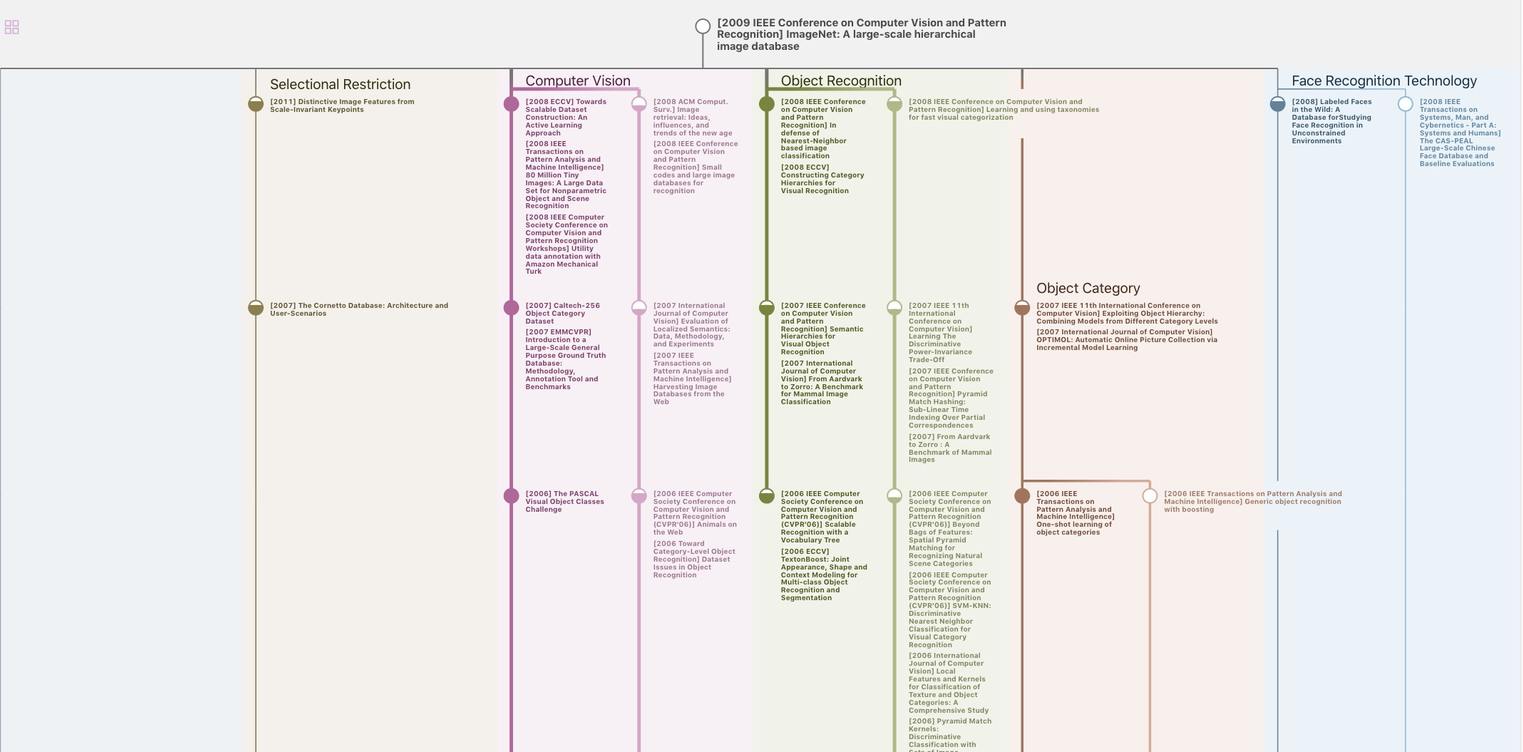
生成溯源树,研究论文发展脉络
Chat Paper
正在生成论文摘要