Robust muscle force prediction using NMFSEMD denoising and FOS identification
PLOS ONE(2022)
摘要
In this paper, an aliasing noise restraint technique and a system identification-based surface electromyography (sEMG)-force prediction model are proposed to realize a type of robust sEMG and muscle force prediction. For signal denoising, a novel non-negative matrix factorization screening empirical mode decomposition (NMFSEMD) and a fast orthogonal search (FOS)-based muscle force prediction model are developed. First, the NMFSEMD model is used to screen the empirical mode decomposition (EMD) results into the noisy intrinsic mode functions (IMF). Then, the noise matrix is computed using IMF translation and superposition, and the matrix is used as the input of NMF to obtain the denoised IMF. Furthermore, the reconstruction outcome of the NMFSEMD method can be used to estimate the denoised sEMG. Finally, a new sEMG muscle force prediction model, which considers a kind of candidate function in derivative form, is constructed, and a data-training-based linear weighted model is obtained. Extensive experimental results validate the suggested method's correction: after the NMFSEMD denoising of raw sEMG signal, the signal-noise ratio (SNR) can be improved by about 15.0 dB, and the energy percentage (EP) can be greater than 90.0%. Comparing with the muscle force prediction models using the traditional pretreatment and LSSVM, and the NMFSEMD plus LSSVM-based method, the mean square error (MSE) of our approach can be reduced by at least 1.2%.
更多查看译文
AI 理解论文
溯源树
样例
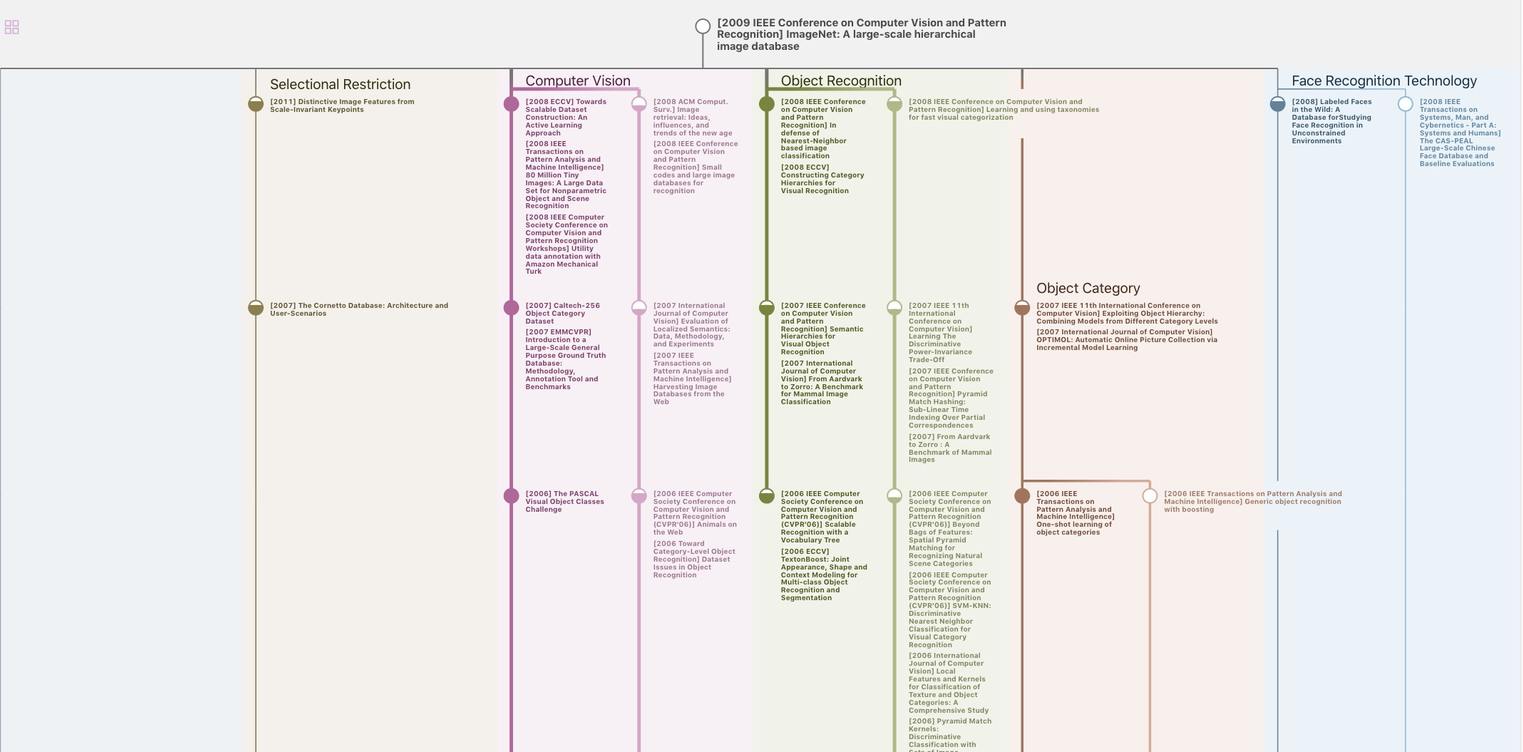
生成溯源树,研究论文发展脉络
Chat Paper
正在生成论文摘要