An electromagnetic signal classification method inspired by the visual characteristics of biological dual-channel
Wireless Networks(2024)
摘要
Automatic modulation classification of electromagnetic signals has an important role in the field of civil and military signal processing, its researches are mainly based on deep learning methods. However, existing deep learning models generally have the problems such as network redundancy and non-interpretability. Since the biological visual system has extraordinary visual signal recognition ability, this paper constructs the biological dual-channel visual neural network (BDVNN) to complete the task of signal classification. The input part of BDVNN simulates the dual-channel structure of lateral geniculate nucleus (LGN), building a dual-channel parallel module composed of convolution kernels of different scales to extract the overall coarse features and local fine features from the input signal. The middle part of BDVNN simulates the fusion of coarse and fine features by the primary visual cortex receptive fields, which facilitates the further extraction of advanced features in subsequent layers of the network. On the setting of network layers, BDVNN model refers to the biological visual pathway hierarchy to meet the lightweight characteristics so as to significantly reduce the training cost. For RadioML2016.10a dataset, the recognition accuracy of BDVNN has 2~17% improvement over the rest of mainstream algorithms. For ACARS dataset, the average recognition accuracy of BDVNN for 20 signals is 95.2%, which is also better than other mainstream algorithms. The experimental results show that the proposed model is lightweight and interpretable, while achieving high recognition accuracy, which provides a new idea for the optimization of the structure and function of deep convolutional neural networks.
更多查看译文
关键词
Automatic modulation classification,Dual-channel neural network,Neural network interpretability,Biological visual pathway model
AI 理解论文
溯源树
样例
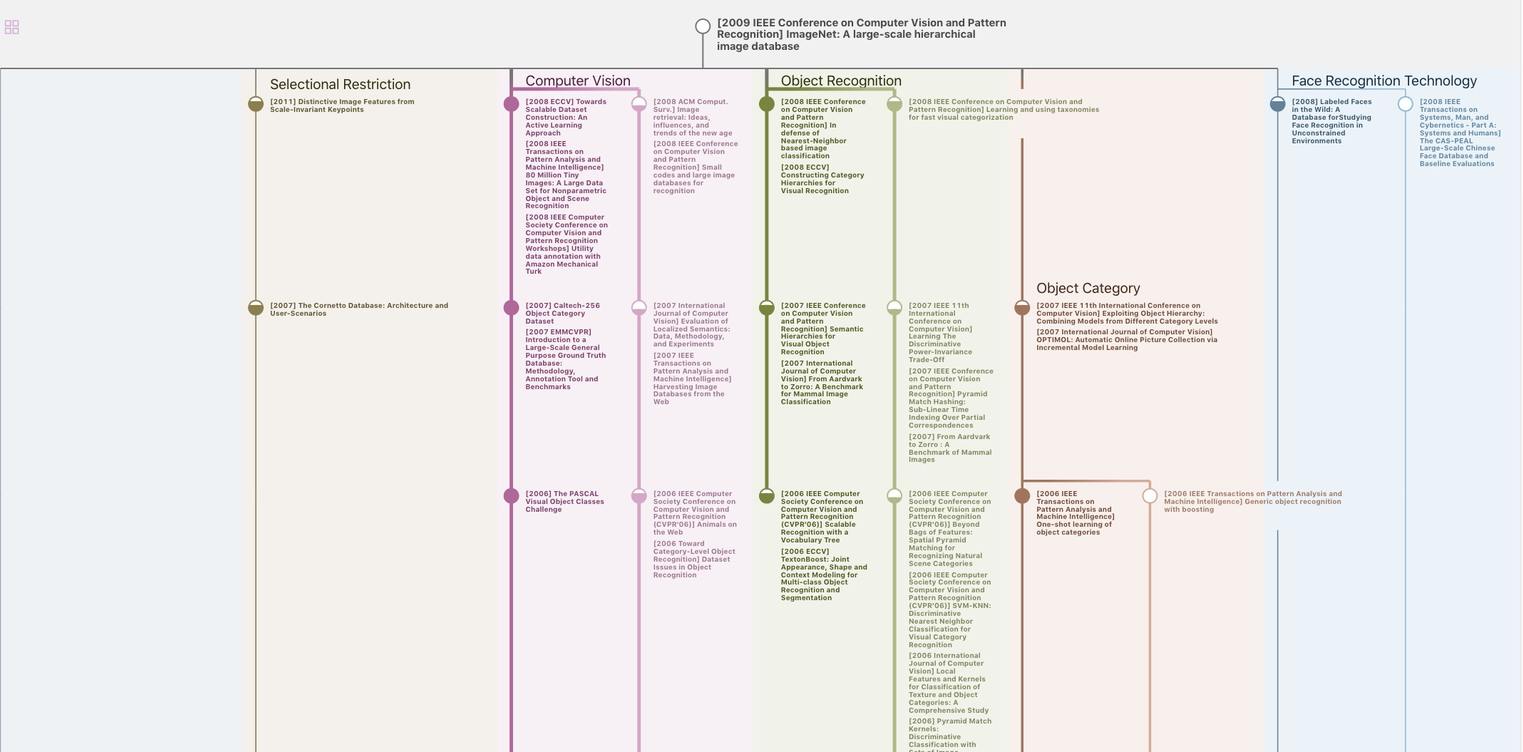
生成溯源树,研究论文发展脉络
Chat Paper
正在生成论文摘要