Cross-domain fault diagnosis through optimal transport for a CSTR process
IFAC-PapersOnLine(2022)
摘要
Fault diagnosis is a key task for developing safer control systems, especially in chemical plants. Nonetheless, acquiring good labeled fault data involves sampling from dangerous system conditions. A possible workaround to this limitation is to use simulation data for training data-driven fault diagnosis systems. However, due to modelling errors or unknown factors, simulation data may differ in distribution from real-world data. This setting is known as cross-domain fault diagnosis (CDFD). We use optimal transport for: (i) exploring how modelling errors relate to the distance between simulation (source) and real-world (target) data distributions, and (ii) matching source and target distributions through the framework of optimal transport for domain adaptation (OTDA), resulting in new training data that follows the target distribution. Comparisons show that OTDA outperforms other CDFD methods. Copyright (C) 2022 The Authors.
更多查看译文
关键词
Fault Diagnosis,Optimal Transport,Transfer Learning
AI 理解论文
溯源树
样例
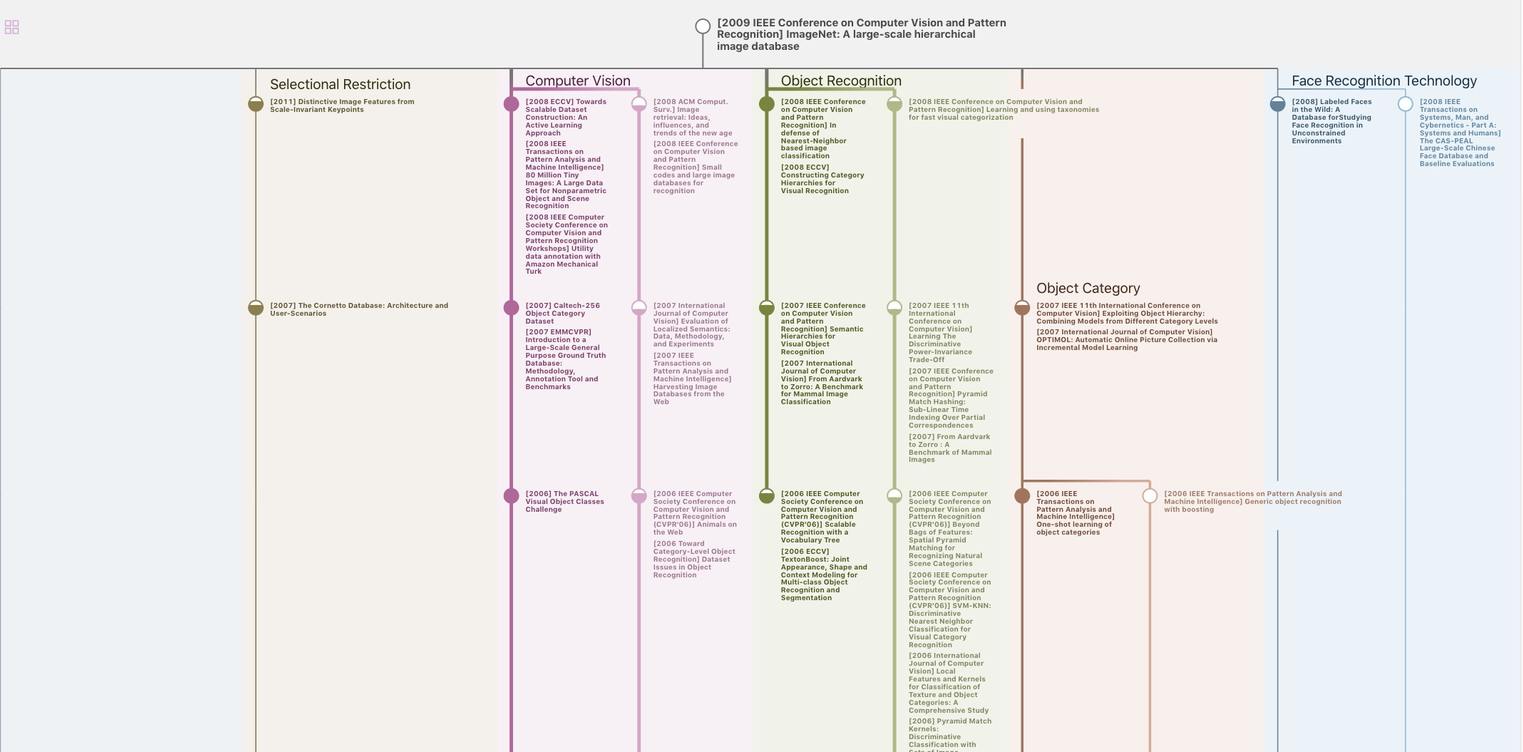
生成溯源树,研究论文发展脉络
Chat Paper
正在生成论文摘要