TransPillars: Coarse-to-Fine Aggregation for Multi-Frame 3D Object Detection
WACV(2023)
摘要
3D object detection using point clouds has attracted increasing attention due to its wide applications in autonomous driving and robotics. However, most existing studies focus on single point cloud frames without harnessing the temporal information in point cloud sequences. In this paper, we design TransPillars, a novel transformer-based feature aggregation technique that exploits temporal features of consecutive point cloud frames for multiframe 3D object detection. TransPillars aggregates spatial-temporal point cloud features from two perspectives. First, it fuses voxel-level features directly from multi-frame feature maps instead of pooled instance features to preserve instance details with contextual information that are essential to accurate object localization. Second, it introduces a hierarchical coarse-to-fine strategy to fuse multi-scale features progressively to effectively capture the motion of moving objects and guide the aggregation of fine features. Besides, a variant of deformable transformer is introduced to improve the effectiveness of cross-frame feature matching. Extensive experiments show that our proposed TransPillars achieves state-of-art performance as compared to existing multi-frame detection approaches.
更多查看译文
关键词
Algorithms: 3D computer vision
AI 理解论文
溯源树
样例
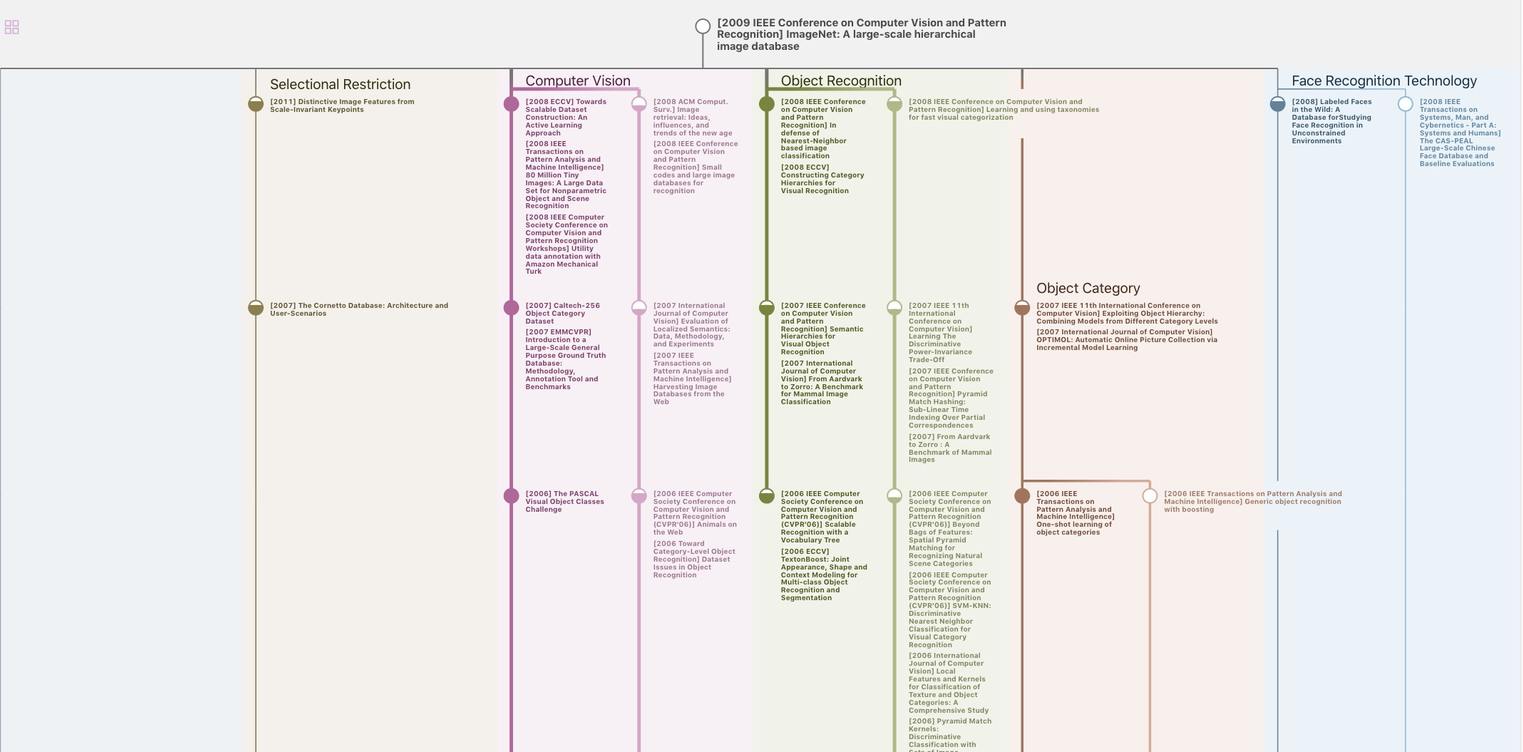
生成溯源树,研究论文发展脉络
Chat Paper
正在生成论文摘要