Brain Lesion Synthesis via Progressive Adversarial Variational Auto-Encoder
SIMULATION AND SYNTHESIS IN MEDICAL IMAGING, SASHIMI 2022(2022)
摘要
Laser interstitial thermal therapy (LITT) is a novel minimally invasive treatment that is used to ablate intracranial structures to treat mesial temporal lobe epilepsy (MTLE). Region of interest (ROI) segmentation before and after LITT would enable automated lesion quantification to objectively assess treatment efficacy. Deep learning techniques, such as convolutional neural networks (CNNs) are state-ofthe-art solutions for ROI segmentation, but require large amounts of annotated data during the training. However, collecting large datasets from emerging treatments such as LITT is impractical. In this paper, we propose a progressive brain lesion synthesis framework (PAVAE) to expand both the quantity and diversity of the training dataset. Concretely, our framework consists of two sequential networks: a mask synthesis network and a mask-guided lesion synthesis network. To better employ extrinsic information to provide additional supervision during network training, we design a condition embedding block (CEB) and a mask embedding block (MEB) to encode inherent conditions of masks to the feature space. Finally, a segmentation network is trained using raw and synthetic lesion images to evaluate the effectiveness of the proposed framework. Experimental results show that our method can achieve realistic synthetic results and boost the performance of down-stream segmentation tasks above traditional data augmentation techniques.
更多查看译文
关键词
Laser interstitial thermal therapy, Adversarial variational auto-encoder, Progressive lesion synthesis
AI 理解论文
溯源树
样例
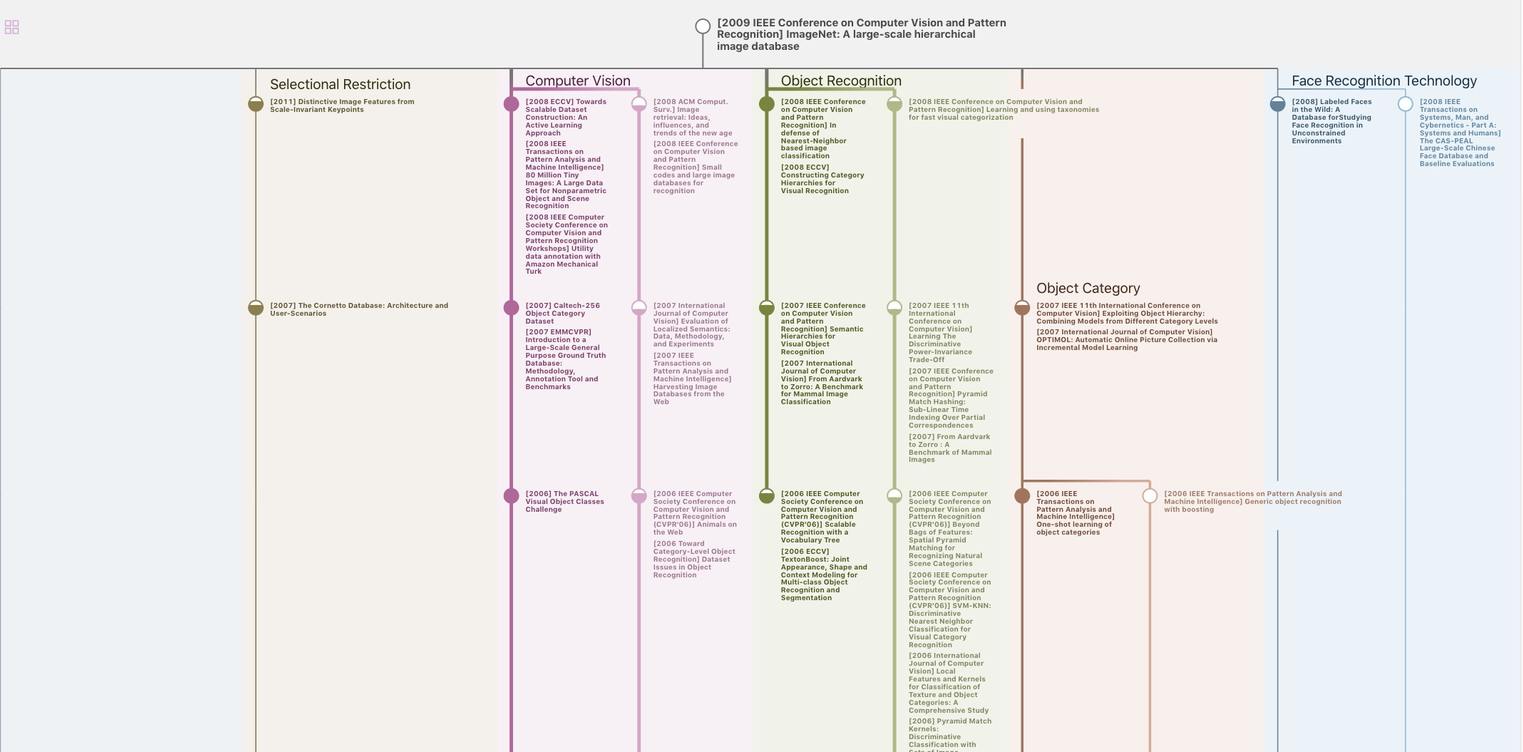
生成溯源树,研究论文发展脉络
Chat Paper
正在生成论文摘要