Development of a Frailty Detection Model Using Machine Learning with the Korean Frailty and Aging Cohort Study Data
HEALTHCARE INFORMATICS RESEARCH(2022)
摘要
Objectives: This paper aimed to use machine learning to identify a new group of factors predicting frailty in the elderly population by utilizing the existing frailty criteria as a basis, as well as to validate the obtained results. Methods: This study was conducted using data from the Korean Frailty and Aging Cohort Study (KFACS). The KFACS participants were classified as robust or frail based on Fried's frailty phenotype and excluded if they did not properly answer the questions, resulting in 1,066 robust and 165 frail participants. We then selected influential features through feature selection and trained the model using support vector machine, random forest, and gradient boosting algorithms with the prepared dataset. Due to the im-balanced distribution in the dataset with a low sample size, holdout was applied with stratified 10-fold and cross-validation for estimating the model performance. The reliability of the constructed model was validated using an unseen test set. The model was then trained with hyperparameter optimization. Results: During the feature selection process, 27 features were identified as meaningful factors for frailty. The model was trained based on the selected features, and the weighted average F1-score reached 95.30% with the random forest algorithm. Conclusions: The results of the study demonstrated the possibil-ity of adopting machine learning to strengthen existing frailty criteria. As the method analyzes questionnaire responses in a short time, it can support higher volumes of data on participants' health conditions and alert them regarding potential risks in advance.
更多查看译文
关键词
Frailty,Aged,Dyskinesias,Surveys and Questionnaire,Machine Learning
AI 理解论文
溯源树
样例
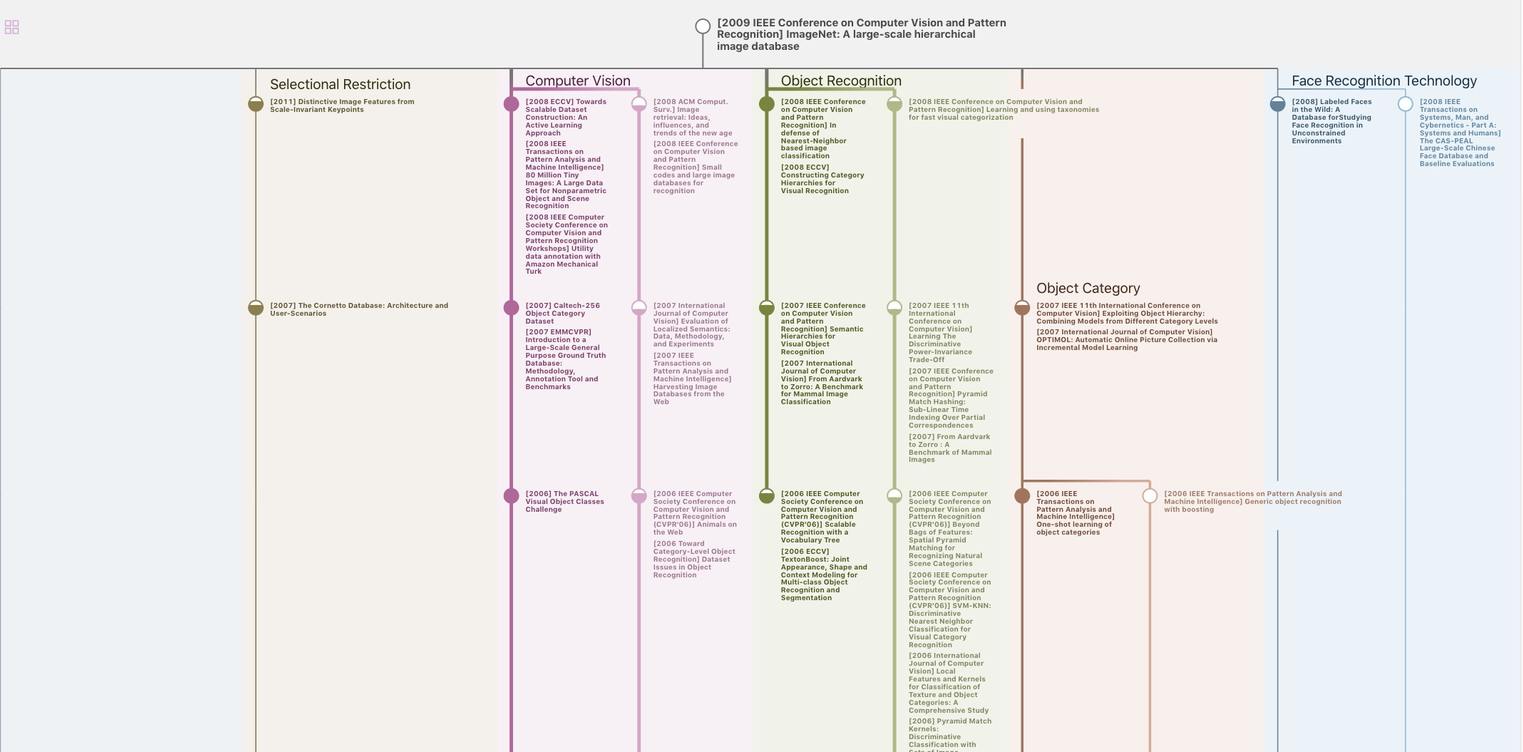
生成溯源树,研究论文发展脉络
Chat Paper
正在生成论文摘要