Collaborative and Incremental Learning for Modulation Classification With Heterogeneous Local Dataset in Cognitive IoT
user-61447a76e55422cecdaf7d19(2023)
摘要
Among 6G high-density connection applications, the cognitive Internet of Things (IoT) is considered to be an effective means to cope with the problem of spectrum resource shortage and reduce energy consumption. The modulation classification with the functions of primary user detection and signal recognition has become the core technology in the cognitive IoT. However, the remarkable surge in the generated data makes it intractable to store and analyze such a large amount of data in a centralized manner. Meanwhile, due to the heterogeneity of IoT devices, the collected data in distributed devices may be non-independent-identical distribution, resulting in heterogeneous local datasets problem. Therefore, we introduce the federated learning (FL) and propose federated incremental learning (FIL) method to address the problem of heterogeneous local datasets in modulation classification. Specifically, we regard the private classes of a particular local device as incremental classes and incremental learning is adopted to learn the classification knowledge of private classes. In order to avoid excessive dispersion between the global model parameters and local model parameters, we utilize knowledge distillation in the local model training stage for imposing similar constrain of parameters on different local models. Simulation results have proven the effectiveness of our proposed method.
更多查看译文
关键词
Cognitive IoT,modulation classification,federated learning,incremental learning,knowledge distillation
AI 理解论文
溯源树
样例
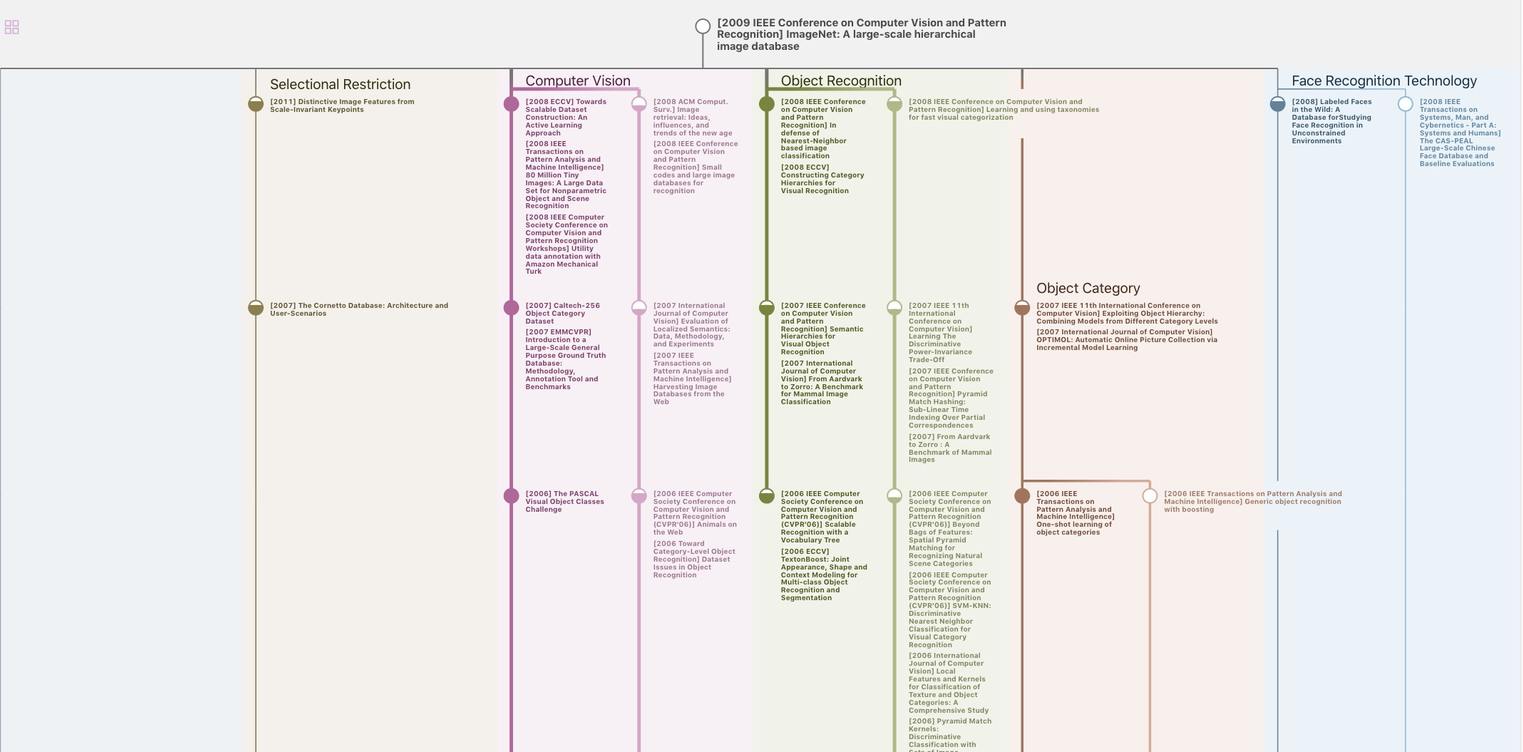
生成溯源树,研究论文发展脉络
Chat Paper
正在生成论文摘要