A hybrid dynamical approach for seasonal prediction of sea‐level anomalies: A pilot study for Charleston, South Carolina
Journal of Geophysical Research: Oceans(2022)
摘要
Using Earth system models for seasonal sea-level prediction remains challenging due to model biases and initialization shocks. Here we present a hybrid dynamical approach for seasonal sea-level prediction to alleviate some of these issues. The approach is based on convolving atmospheric forcings with sea-level sensitivities to these forcings. The sensitivities are pre-computed by the adjoint model of the Estimating Circulation and Climate of the Ocean (ECCO) system. The forcings are a concatenation of ECCO forcings before prediction initialization and a 10-member predicted atmospheric forcing ensemble from the Community Climate System Model version 4 (CCSM4) after initialization, with offline forcing bias corrections applied using the observationally-constrained ECCO seasonal forcing climatology. As a pilot study, we conducted 12-month hindcasts from 1995 to 2016 in Charleston (United States East Coast). Our approach avoids drifts in CCSM4 sea-level predictions and beats seasonal climatology and damped persistence as predictors up to a 6-month lead time. The prediction skill comes from two factors: (a) ECCO forcings prior to prediction initialization influence sea level after initialization through delayed oceanic adjustments (e.g., coastally-trapped waves, open-ocean Rossby waves, and advection of steric anomalies) leading to skillful predictions beyond 2 months after initialization, and (b) the 10-member CCSM4 ensemble forcing predictions have relatively good skill at 1-2 months lead times. Our method is computationally efficient for operational sea-level prediction at specific locations and can attribute sea-level prediction skill and uncertainty to specific forcings or forcing from particular regions, thereby providing useful information to seasonal prediction centers for improving their prediction systems.
更多查看译文
关键词
sea-level prediction, adjoint models, ocean dynamics, climate predictability, coastal hazards
AI 理解论文
溯源树
样例
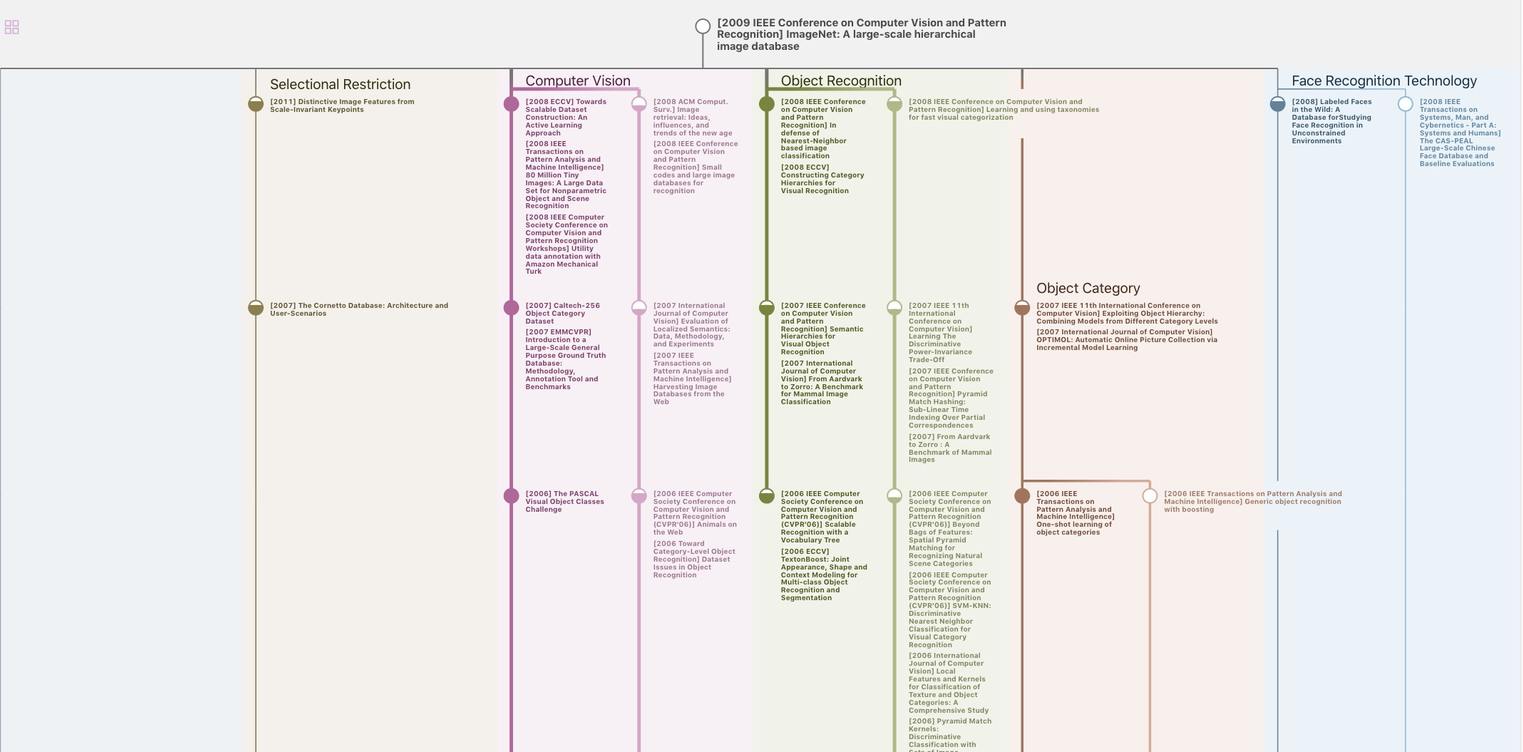
生成溯源树,研究论文发展脉络
Chat Paper
正在生成论文摘要