Developing A Multi-task Edge-attention based Graph Deep Learning Algorithm for Kinase Polypharmacology Profiling.
2022 14th International Conference on Bioinformatics and Biomedical Technology(2022)
摘要
The kinome-wide virtual profiling of molecules with structure data is a challenging task in drug discovery. Here, we present a virtual predicting model against a group of 118 kinases based on large-scale bioactivity data and the multitask graph deep neural network algorithm. The obtained model yields excellent prediction ability with auROC and F1-score of 0.8 on an internal testing dataset. Compared with conventional multi-tasks deep neural network models, the model consistently shows higher auROCs and F1-score on external datasets, despite the apparent deviation of chemical diversity distribution and the uncertainty in different data sources. Visualizing the weights of attention layers in this model can open the black box of this deep learning model and explain its prediction mechanism. Overall, this computational model enables us to create a comprehensive kinome interaction network for designing novel chemical modulators or drug repositioning and is of practical value for exploring new drug molecular structure.
更多查看译文
关键词
kinase polypharmacology profiling,graph deep learning algorithm,deep learning,multi-task,edge-attention
AI 理解论文
溯源树
样例
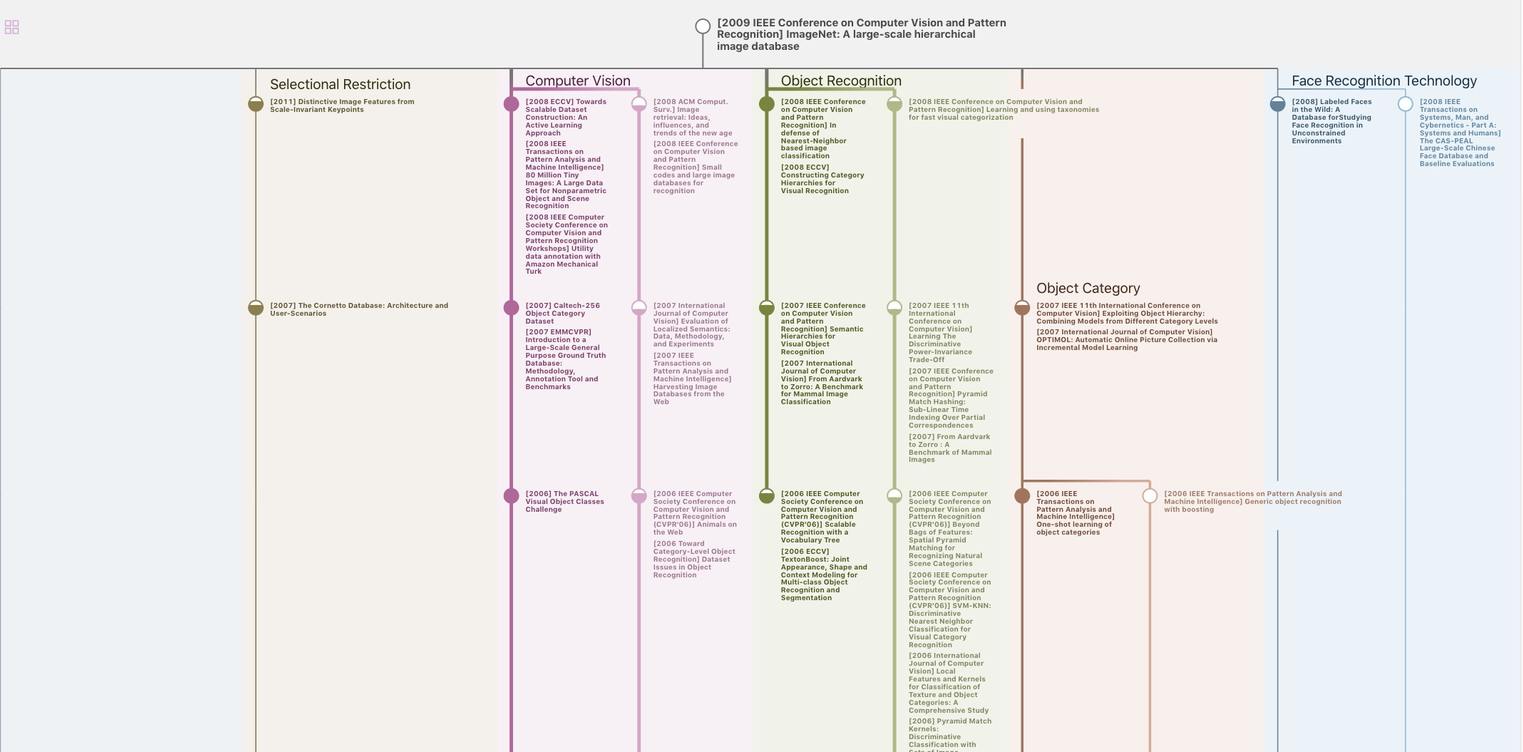
生成溯源树,研究论文发展脉络
Chat Paper
正在生成论文摘要