SMITH: A Self-supervised Downstream-Aware Framework for Missing Testing Data Handling.
user-61447a76e55422cecdaf7d19(2022)
摘要
Missing values in testing data has been a notorious problem in machine learning community since it can heavily deteriorate the performance of downstream model learned from complete data without any precaution. To better perform the prediction task with this kind of downstream model, we must impute the missing value first. Therefore, the imputation quality and how to utilize the knowledge provided by the pre-trained and fixed downstream model are the keys to address this problem. In this paper, we aim to address this problem and focus on models learned from tabular data. We present a novel Self-supervised downstream-aware framework for MIssing Testing data Handling (SMITH), which consists of a transformer-based imputation model and a downstream label estimation algorithm. The former can be replaced by any existing imputation model of interest with additional performance gain acquired in comparison with that of their original design. By advancing two self-supervised tasks and the knowledge from the prediction of the downstream model to guide the learning of our transformer-based imputation model, our SMITH framework performs favorably against state-of-the-art methods under several benchmarking datasets.
更多查看译文
关键词
Missing testing data, Downstream-aware, Transformer, Self-supervised learning, Tabular data
AI 理解论文
溯源树
样例
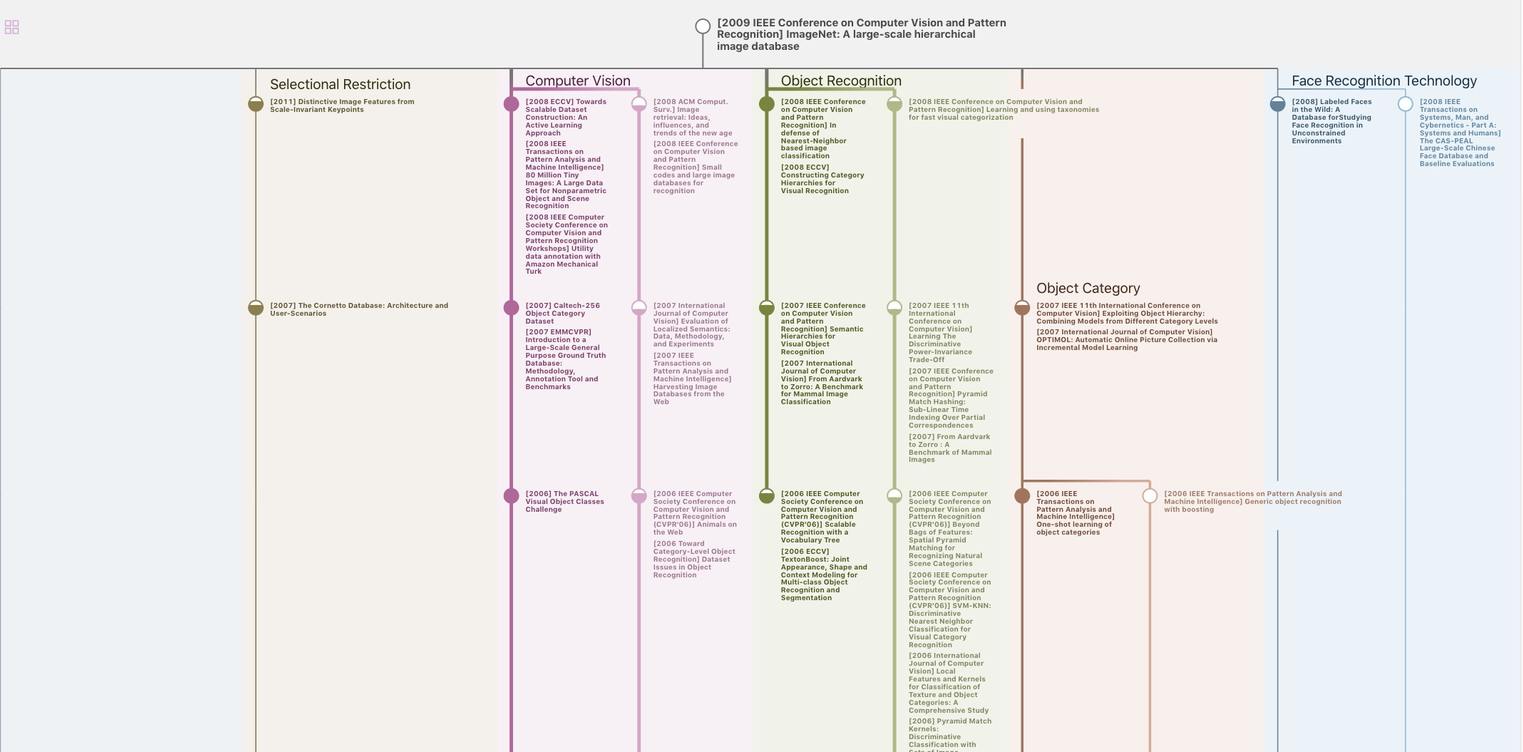
生成溯源树,研究论文发展脉络
Chat Paper
正在生成论文摘要