Triple Sparsification of Graph Convolutional Networks without Sacrificing the Accuracy
arxiv(2022)
摘要
Graph Neural Networks (GNNs) are widely used to perform different machine learning tasks on graphs. As the size of the graphs grows, and the GNNs get deeper, training and inference time become costly in addition to the memory requirement. Thus, without sacrificing accuracy, graph sparsification, or model compression becomes a viable approach for graph learning tasks. A few existing techniques only study the sparsification of graphs and GNN models. In this paper, we develop a SparseGCN pipeline to study all possible sparsification in GNN. We provide a theoretical analysis and empirically show that it can add up to 11.6\% additional sparsity to the embedding matrix without sacrificing the accuracy of the commonly used benchmark graph datasets.
更多查看译文
关键词
graph convolutional networks
AI 理解论文
溯源树
样例
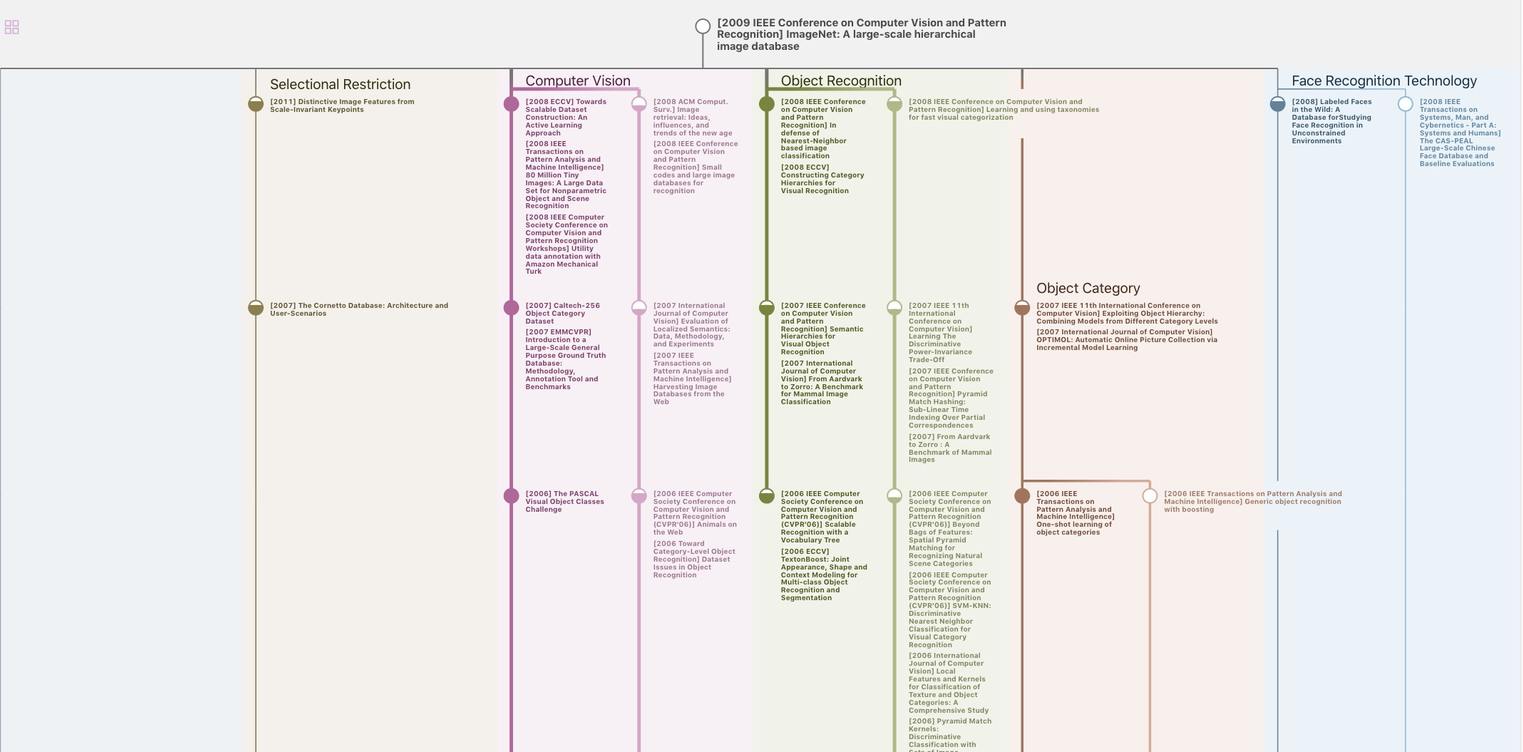
生成溯源树,研究论文发展脉络
Chat Paper
正在生成论文摘要