A Distributed Pricing Strategy for Edge Computation Offloading Optimization in Autonomous Driving
IEEE NETWORK(2023)
摘要
The increase of on-vehicle applications has brought explosive computation demands to autonomous vehicles and overwhelmed their limited onboard resources. Edge computing can offload application load and effectively alleviate this problem. However, the introduction of edge computing faces significant challenges, including the considerable amount of resource contention due to the scarcity of edge resources and the competition among edge computing resource providers to earn users' services requests. We notice that the problem is not purely technical as solutions for these two problems can become conflicting to each other. In this paper, we propose a distributed pricing strategy to achieve full use of computing resources at the edge and maximize the revenue of service operators, both with guaranteed quality-of-service of on-vehicle applications. More specifically, we first use the multi-leader multi-follower Stackelberg game theory to model the pricing of on-vehicle task offloading under edge computing. Next, we propose a distributed pricing strategy to enable edge servers to adjust their local price distributions so that edge servers can bargain with offloading requesters independently. Experimental results confirm that the proposed distributed pricing strategy can provide more optimized server computing resource utilization while guaranteeing the performance of in-vehicle applications.
更多查看译文
关键词
Servers,Task analysis,Pricing,Game theory,Edge computing,Autonomous vehicles,Computational modeling,Distributed processing,Resource management,Intelligent vehicles,Vehicular ad hoc networks,Performance evaluation
AI 理解论文
溯源树
样例
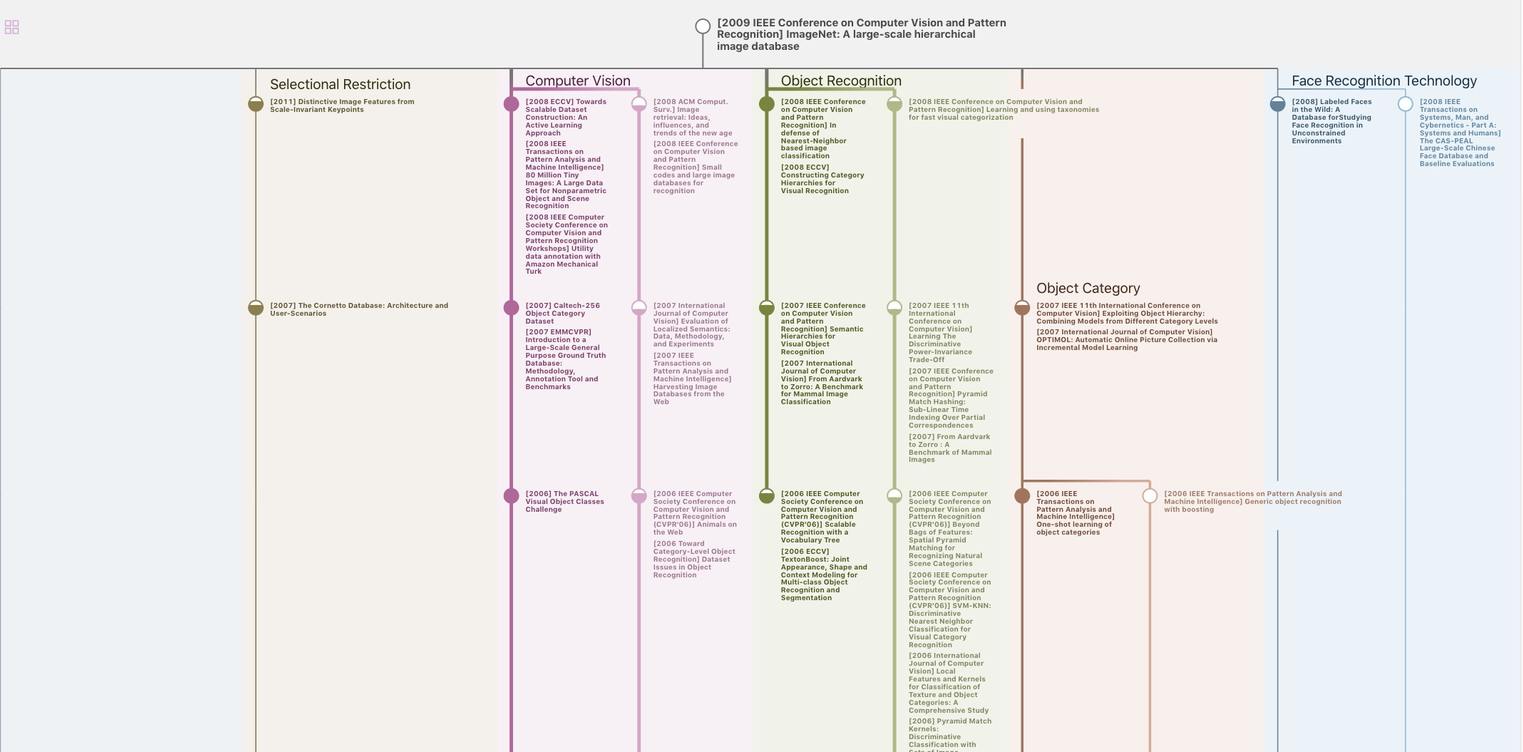
生成溯源树,研究论文发展脉络
Chat Paper
正在生成论文摘要