Template Matching Based Early Exit CNN for Energy-efficient Myocardial Infarction Detection on Low-power Wearable Devices.
Proceedings of the ACM on Interactive, Mobile, Wearable and Ubiquitous Technologies(2022)
摘要
Myocardial Infarction (MI), also known as heart attack, is a life-threatening form of heart disease that is a leading cause of death worldwide. Its recurrent and silent nature emphasizes the need for continuous monitoring through wearable devices. The wearable device solutions should provide adequate performance while being resource-constrained in terms of power and memory. This paper proposes an MI detection methodology using a Convolutional Neural Network (CNN) that outperforms the state-of-the-art works on wearable devices for two datasets - PTB and PTB-XL, while being energy and memory-efficient. Moreover, we also propose a novel Template Matching based Early Exit (TMEX) CNN architecture that further increases the energy efficiency compared to baseline architecture while maintaining similar performance. Our baseline and TMEX architecture achieve 99.33% and 99.24% accuracy on PTB dataset, whereas on PTB-XL dataset they achieve 84.36% and 84.24% accuracy, respectively. Both architectures are suitable for wearable devices requiring only 20 KB of RAM. Evaluation of real hardware shows that our baseline architecture is 0.6x to 53x more energy-efficient than the state-of-the-art works on wearable devices. Moreover, our TMEX architecture further improves the energy efficiency by 8.12% (PTB) and 6.36% (PTB-XL) while maintaining similar performance as the baseline architecture.
更多查看译文
关键词
early exit CNN,energy-efficiency,myocardial infarction,wearable devices
AI 理解论文
溯源树
样例
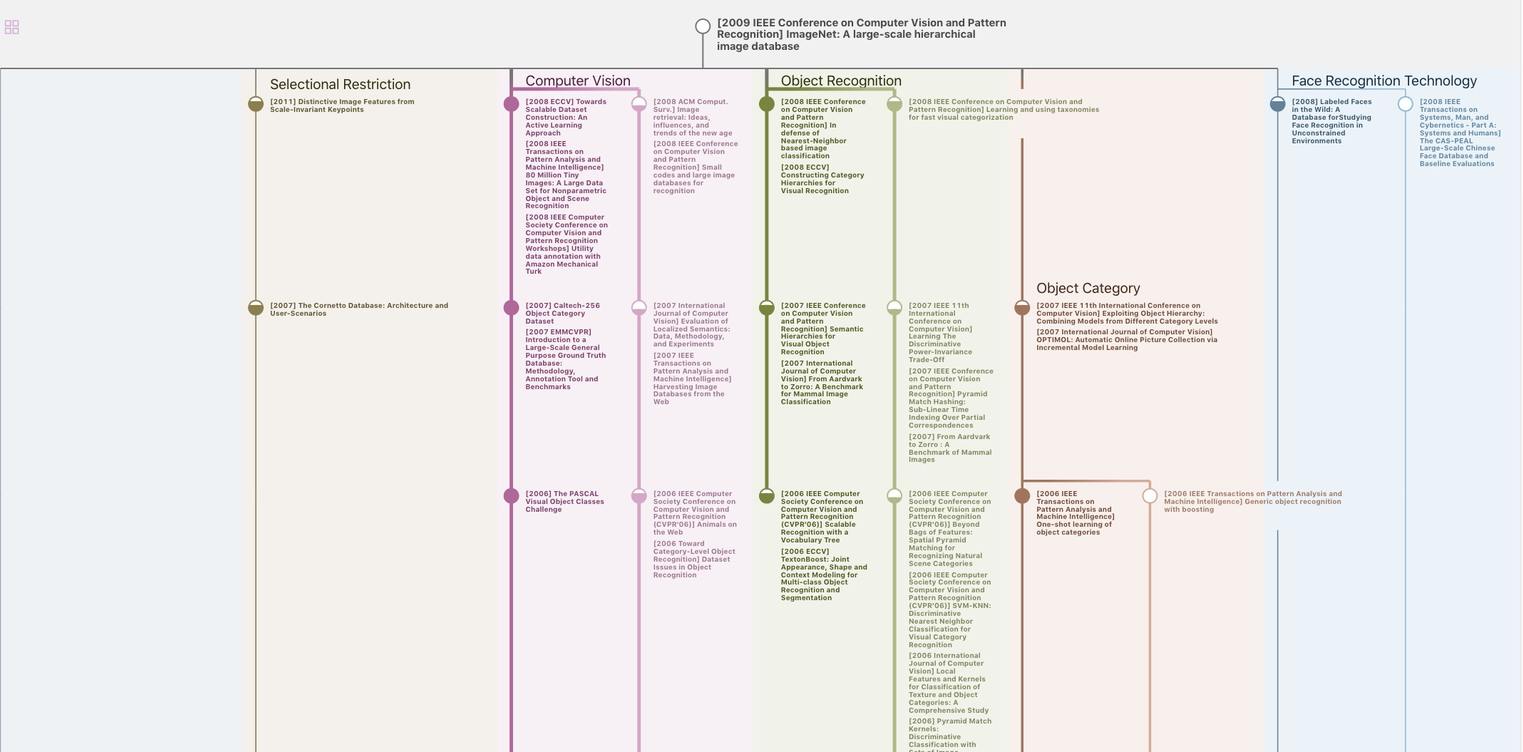
生成溯源树,研究论文发展脉络
Chat Paper
正在生成论文摘要