Spatial-Temporal Deep Learning for Hosting Capacity Analysis in Distribution Grids
IEEE Transactions on Smart Grid(2023)
摘要
The widespread use of distributed energy sources (DERs) raises significant challenges for power system design, planning, and operation, leading to wide adaptation of tools on hosting capacity analysis (HCA). Traditional HCA methods conduct extensive power flow analysis. Due to the computation burden, these time-consuming methods fail to provide online hosting capacity (HC) in large distribution systems. To solve the problem, we first propose a deep learning-based problem formulation for HCA, which conducts offline training and determines HC in real time. The used learning model, long short-term memory (LSTM), implements historical time-series data to capture periodical patterns in distribution systems. However, directly applying LSTMs suffers from low accuracy due to the lack of consideration on spatial information, where location information like feeder topology is critical in nodal HCA. Therefore, we modify the forget gate function to dual forget gates, to capture the spatial correlation within the grid. Such a design turns the LSTM into the Spatial-Temporal LSTM (ST-LSTM). Moreover, as voltage violations are the most vital constraints in HCA, we design a voltage sensitivity gate to increase accuracy further. The results of LSTMs and ST-LSTMs on feeders, such as IEEE 34-, 123-bus feeders, and utility feeders, validate our designs.
更多查看译文
关键词
Hosting capacity,deep learning,data-driven method,long short-term memory (LSTM),spatial-temporal correlation,distributed energy resource
AI 理解论文
溯源树
样例
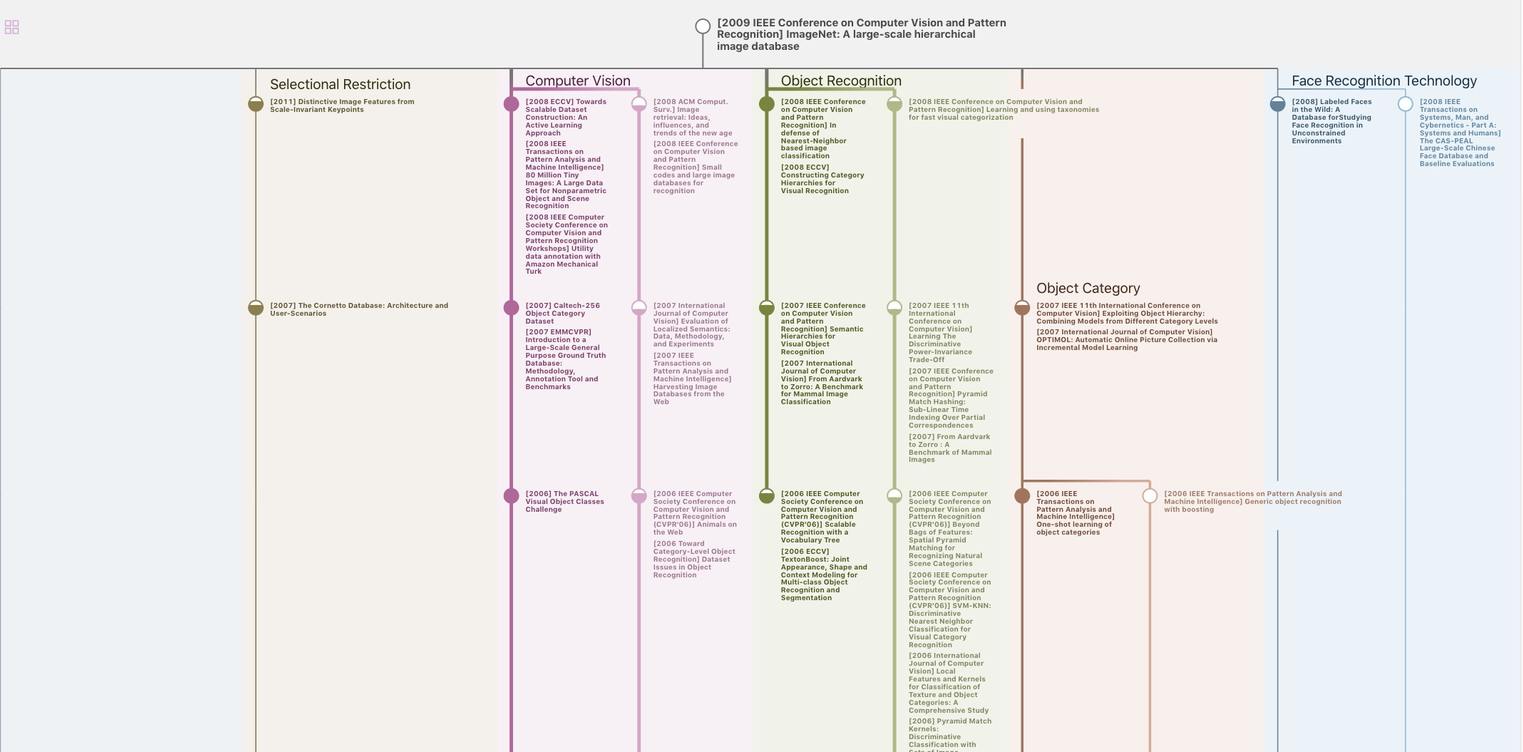
生成溯源树,研究论文发展脉络
Chat Paper
正在生成论文摘要