MetaProfiling: Inferring User Profiles with Few-Shot Data.
IEEE International Conference on High Performance Computing and Communications(2021)
摘要
Inferring user profiles plays a pivotal role in various real-world scenarios. The rapid development of the online social media enables user profiling based on their interacted data. However, some user profiles are diverse and constantly changing. On the one hand, a number of new occupations are emerging, but only with few labeled users. This often leads to the class imbalance problem. On the other hand, there are some similar occupation classes which are difficult to distinguish. These issues bring new challenges to build the user profiles. In this paper, we propose a novel model, named MetaProfiling, to solve the few-shot user profiling problem, i.e., distinguishing occupation classes with few labeled users. Firstly, we extract multi-field features from multi-faceted user information, including user personal attributes and behavior attributes, to characterize users from different aspects. Then, we design a user embedding generator to learn an effective user embedding space by using the feature attention layer and the user embedding fusion layer. Finally, we design a margin-based user profiling classifier to effectively distinguish users from different classes, especially for the similar classes. Specifically, the classifier can enlarge the margin between the similar classes. Experiments on a real-world dataset from a question answering community validate that our approach can consistly outperform many baseline methods under both standard and generalized few-shot learning settings.
更多查看译文
关键词
user profiling,meta-learning,few-shot learning,user attribute inference,class imbalance problem
AI 理解论文
溯源树
样例
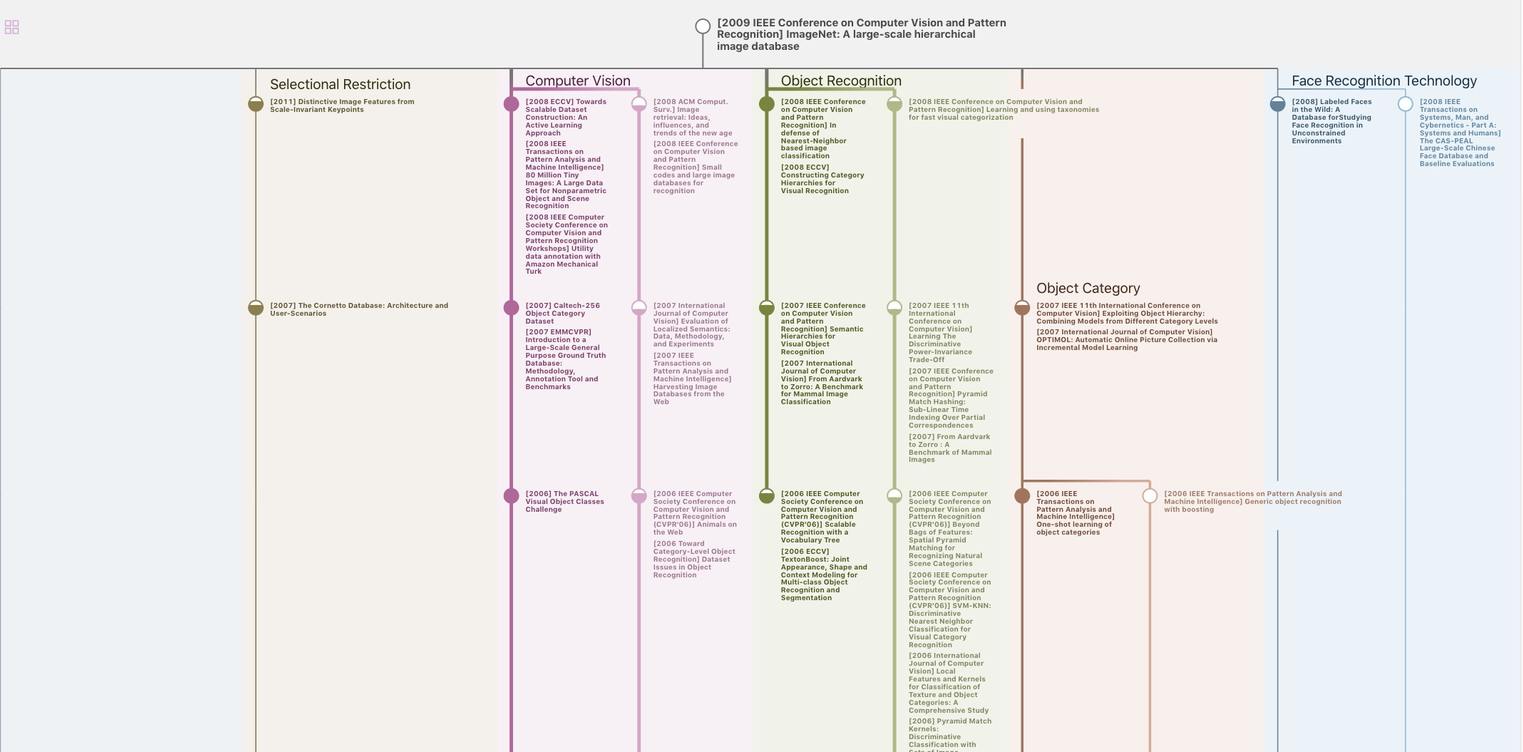
生成溯源树,研究论文发展脉络
Chat Paper
正在生成论文摘要