DGPolarNet: Dynamic Graph Convolution Network for LiDAR Point Cloud Semantic Segmentation on Polar BEV
REMOTE SENSING(2022)
摘要
Semantic segmentation in LiDAR point clouds has become an important research topic for autonomous driving systems. This paper proposes a dynamic graph convolution neural network for LiDAR point cloud semantic segmentation using a polar bird's-eye view, referred to as DGPolarNet. LiDAR point clouds are converted to polar coordinates, which are rasterized into regular grids. The points mapped onto each grid distribute evenly to solve the problem of the sparse distribution and uneven density of LiDAR point clouds. In DGPolarNet, a dynamic feature extraction module is designed to generate edge features of perceptual points of interest sampled by the farthest point sampling and K-nearest neighbor methods. By embedding edge features with the original point cloud, local features are obtained and input into PointNet to quantize the points and predict semantic segmentation results. The system was tested on the Semantic KITTI dataset, and the segmentation accuracy reached 56.5%
更多查看译文
关键词
semantic segmentation,polar BEV,LiDAR point cloud,dynamic graph convolution network
AI 理解论文
溯源树
样例
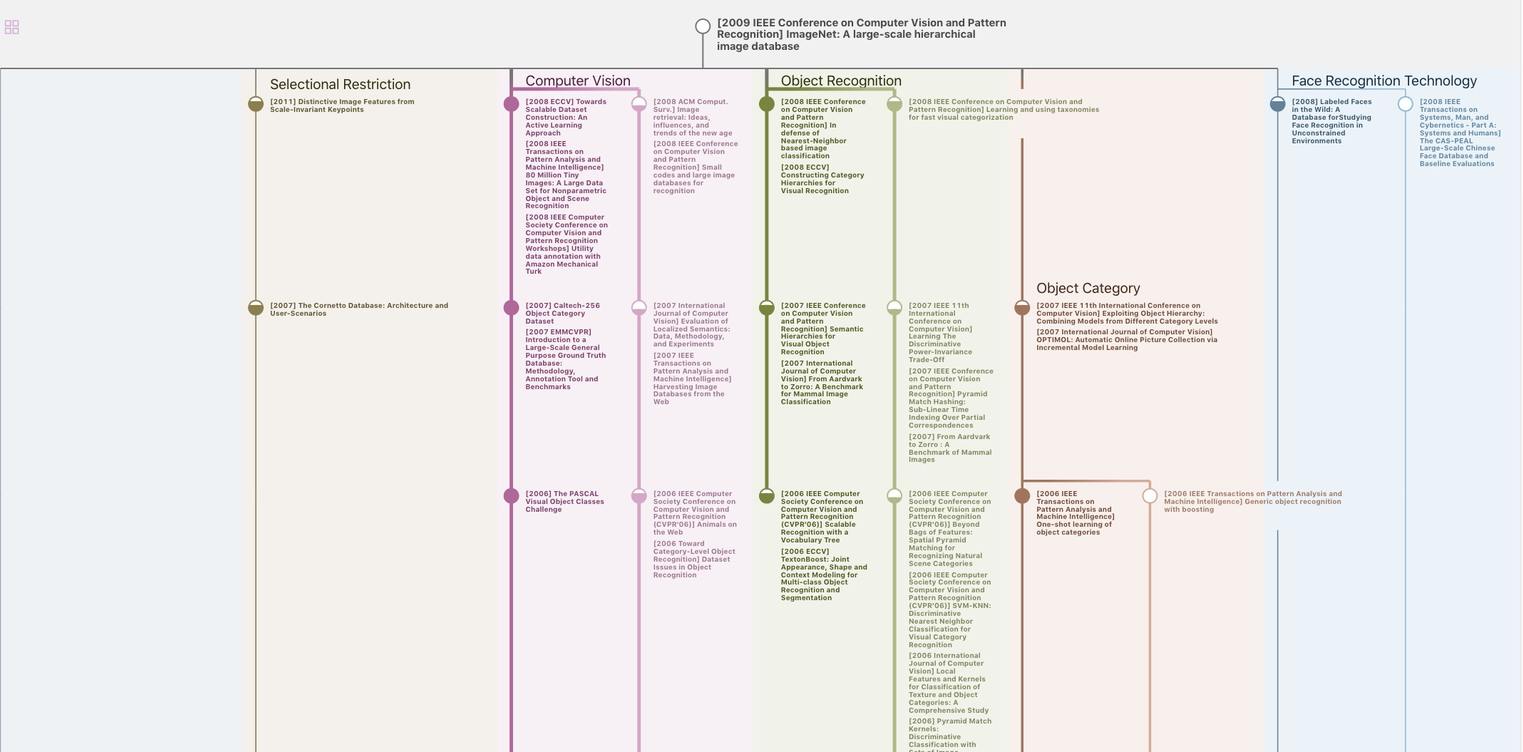
生成溯源树,研究论文发展脉络
Chat Paper
正在生成论文摘要