Constrained Robust Bayesian Optimization of Expensive Noisy Black‐box Functions with Guaranteed Regret Bounds
AIChE journal(2022)
摘要
Many real-world design problems involve optimization of expensive black-box functions. Bayesian optimization (BO) is a promising approach for solving such challenging problems using probabilistic surrogate models to systematically tradeoff between exploitation and exploration of the design space. Although BO is often applied to unconstrained problems, it has recently been extended to the constrained setting. Current constrained BO methods, however, cannot identify solutions that are robust to unavoidable uncertainties. In this article, we propose a robust constrained BO method, constrained adversarially robust Bayesian optimization (CARBO), that addresses this challenge by jointly modeling the effect of the design variables and uncertainties on the unknown functions. Using exact penalty functions, we establish a bound on the number of CARBO iterations required to find a near-global robust solution and provide a rigorous proof of convergence. The advantages of CARBO are demonstrated on two case studies including a non-convex benchmark problem and a realistic bubble column reactor design problem.
更多查看译文
关键词
black-box optimization,derivative-free optimization under uncertainty,exact penalty functions,Gaussian processes,robust Bayesian optimization
AI 理解论文
溯源树
样例
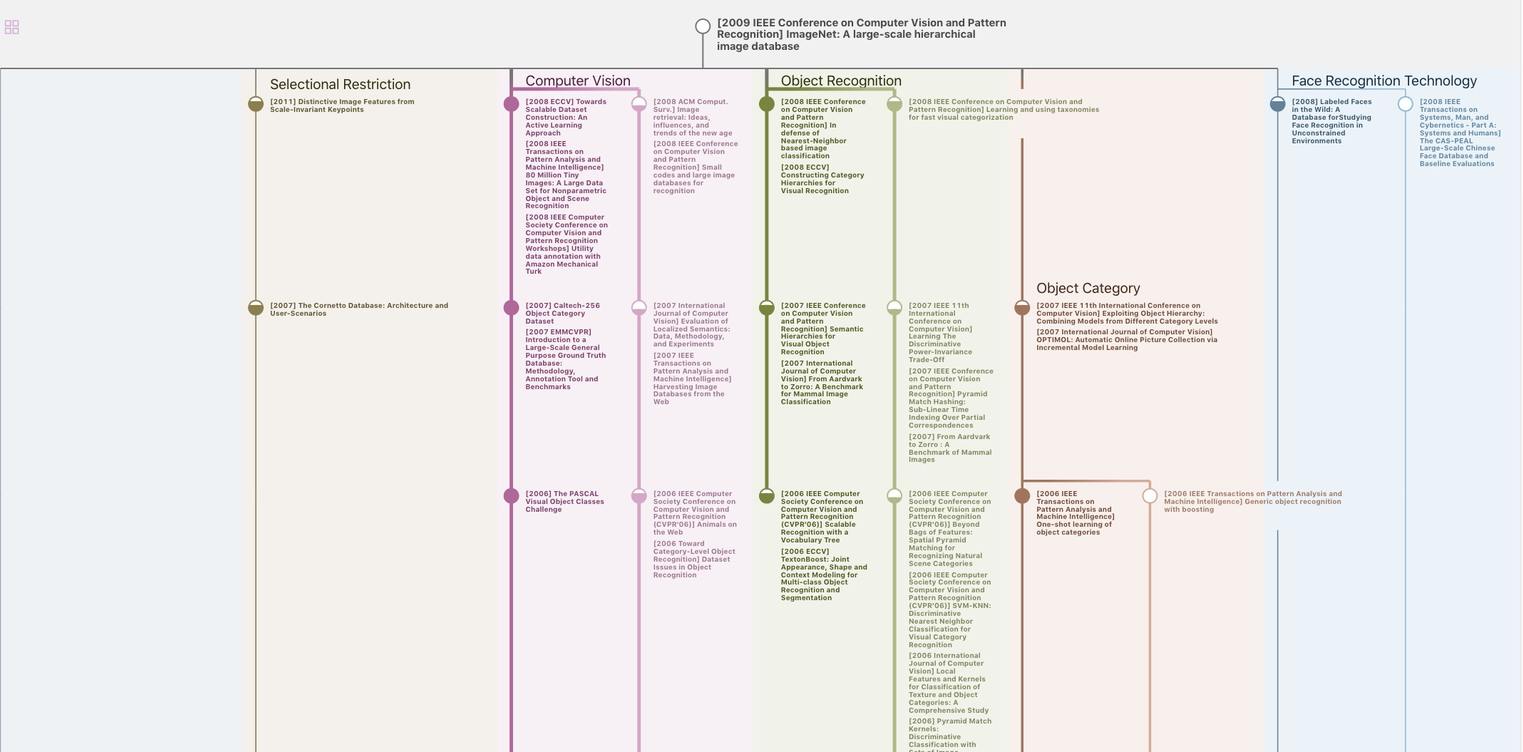
生成溯源树,研究论文发展脉络
Chat Paper
正在生成论文摘要