Learning from Visual Demonstrations via Replayed Task-Contrastive Model-Agnostic Meta-Learning
IEEE Transactions on Circuits and Systems for Video Technology(2022)
摘要
With the increasing application of versatile robotics, the need for end-users to teach robotic tasks via visual/video demonstrations in different environments is increasing fast. One possible method is meta-learning. However, most meta-learning methods are tailored for image classification or just focus on teaching the robot what to do, resulting in a limited ability of the robot to adapt to the real world. Thus, we propose a novel yet efficient model-agnostic meta-learning framework based on task-contrastive learning to teach the robot what to do and what not to do through positive and negative demonstrations. Our approach divides the learning procedure from visual/video demonstrations into three parts. The first part distinguishes between positive and negative demonstrations via task-contrastive learning. The second part emphasizes what the positive demo is doing, and the last part predicts what the robot needs to do. Finally, we demonstrate the effectiveness of our meta-learning approach on 1) two standard public simulated benchmarks and 2) real-world placing experiments using a UR5 robot arm, significantly outperforming current related state-of-the-art methods.
更多查看译文
关键词
Meta-learning,learning from demonstrations,one-shot visual imitation learning,learning to learn
AI 理解论文
溯源树
样例
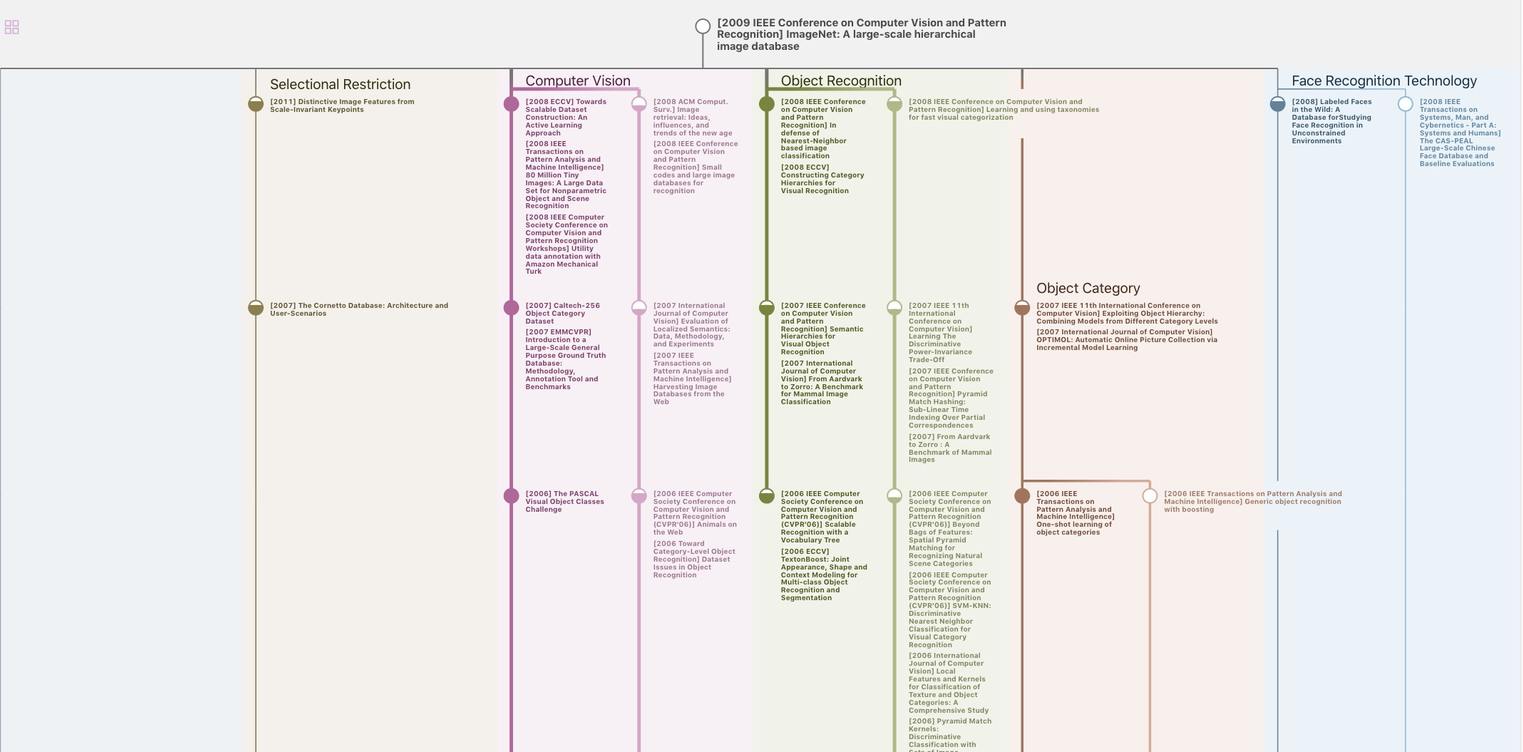
生成溯源树,研究论文发展脉络
Chat Paper
正在生成论文摘要