Classification of Precipitation Types Based on Machine Learning Using Dual-Polarization Radar Measurements and Thermodynamic Fields
REMOTE SENSING(2022)
摘要
An accurate classification of the precipitation type is important for forecasters, particularly in the winter season. We explored the capability of three supervised machine learning (ML) methods (decision tree, random forest, and support vector machine) to determine ground precipitation types (no precipitation, rain, mixed, and snow) for winter precipitation. We provided information on the particle characteristics within a radar sampling volume and the environmental condition to the ML model with the simultaneous use of polarimetric radar variables and thermodynamic variables. The ML algorithms were optimized using predictor selection and hyperparameter tuning in order to maximize the computational efficiency and accuracy. The random forest (RF) had the highest skill scores in all precipitation types and outperformed the operational scheme. The spatial distribution of the precipitation type from the RF model showed a good agreement with the surface observation. As a result, RF is recommended for the real-time precipitation type classification due to its easy implementation, computational efficiency, and satisfactory accuracy. In addition to the validation, this study confirmed the strong dependence of precipitation type on wet-bulb temperature and a 1000-850 hPa layer thickness. The results also suggested that the base heights of the radar echo are useful in discriminating non-precipitating area.
更多查看译文
关键词
precipitation type,machine learning,dual-polarization radar,thermodynamic field
AI 理解论文
溯源树
样例
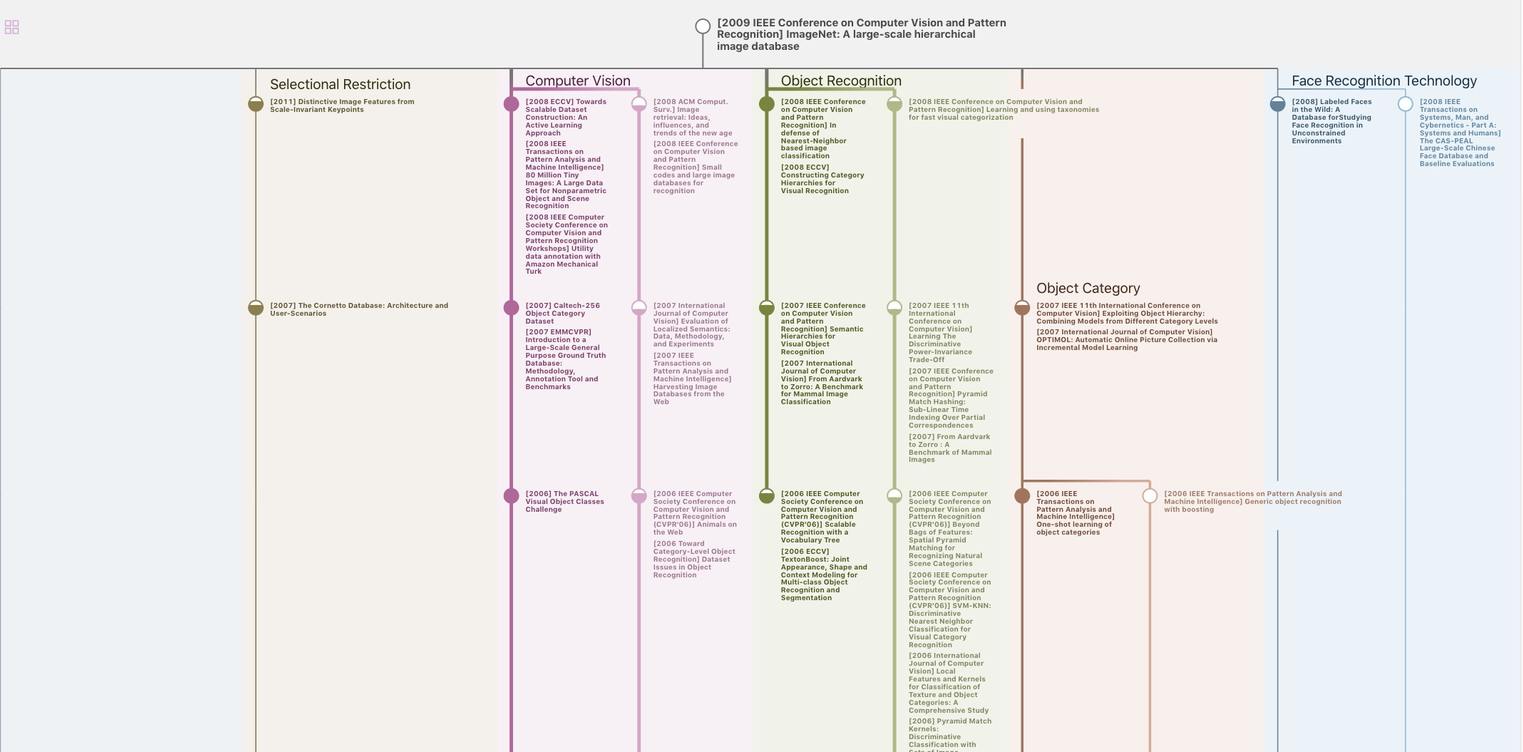
生成溯源树,研究论文发展脉络
Chat Paper
正在生成论文摘要