Double Attention Convolutional Neural Network for Sequential Recommendation.
ACM transactions on the web(2022)
摘要
The explosive growth of e-commerce and online service has led to the development of recommender system. Aiming to provide a list of items to meet a user’s personalized need by analyzing his/her interaction 1 history, recommender system has been widely studied in academic and industrial communities. Different from conventional recommender systems, sequential recommender systems attempt to capture the pattern of users’ sequential behaviors and the evolution of users’ preferences. Most of the existing sequential recommendation models only focus on user interaction sequence, but neglect item interaction sequence. An item interaction sequence also contains rich contextual information for capturing the item’s dynamic characteristic, since an item’s dynamic characteristic can be reflected by the users who interact with it in a period. Furthermore, existing dual sequential models use the same method to handle the user interaction sequence and item interaction sequence, and do not consider their different characteristics. Hence, we propose a novel D ouble A ttention C onvolution N eural N etwork (DACNN) , which incorporates user interaction sequence and item interaction sequence into an integrated neural network framework. DACNN leverages the strength of attention mechanism to capture the temporary suitability and adopts CNN to extract local sequential features. Experimental evaluations on the real datasets show that DACNN outperforms the baseline approaches.
更多查看译文
关键词
Recommender system,sequential prediction,neural network,deep learning
AI 理解论文
溯源树
样例
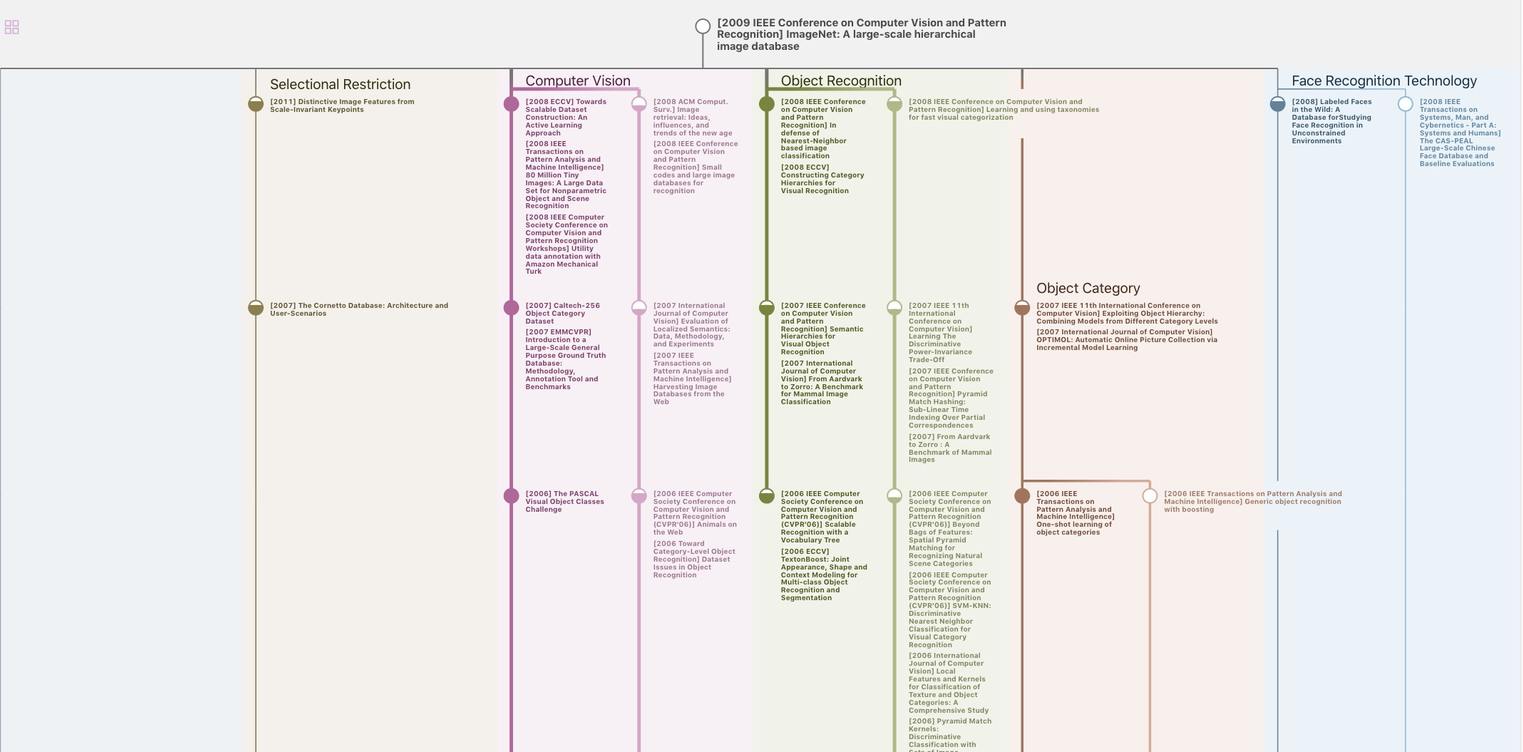
生成溯源树,研究论文发展脉络
Chat Paper
正在生成论文摘要