Airborne Radiometric Surveys and Machine Learning Algorithms for Revealing Soil Texture
REMOTE SENSING(2022)
摘要
Soil texture is key information in agriculture for improving soil knowledge and crop performance, so the accurate mapping of this crucial feature is imperative for rationally planning cultivations and for targeting interventions. We studied the relationship between radioelements and soil texture in the Mezzano Lowland (Italy), a 189 km(2) agricultural plain investigated through a dedicated airborne gamma-ray spectroscopy survey. The K and Th abundances were used to retrieve the clay and sand content by means of a multi-approach method. Linear (simple and multiple) and non-linear (machine learning algorithms with deep neural networks) predictive models were trained and tested adopting a 1:50,000 scale soil texture map. The comparison of these approaches highlighted that the non-linear model introduces significant improvements in the prediction of soil texture fractions. The predicted maps of the clay and of the sand content were compared with the regional soil maps. Although the macro-structures were equally present, the airborne gamma-ray data permits us shedding light on finer features. Map areas with higher clay content were coincident with paleo-channels crossing the Mezzano Lowland in Etruscan and Roman periods, confirmed by the hydrographic setting of historical maps and by the geo-morphological features of the study area.
更多查看译文
关键词
airborne gamma-ray spectroscopy,non-linear machine learning,potassium,clay,thorium,sand,soil texture,paleo-hydrography
AI 理解论文
溯源树
样例
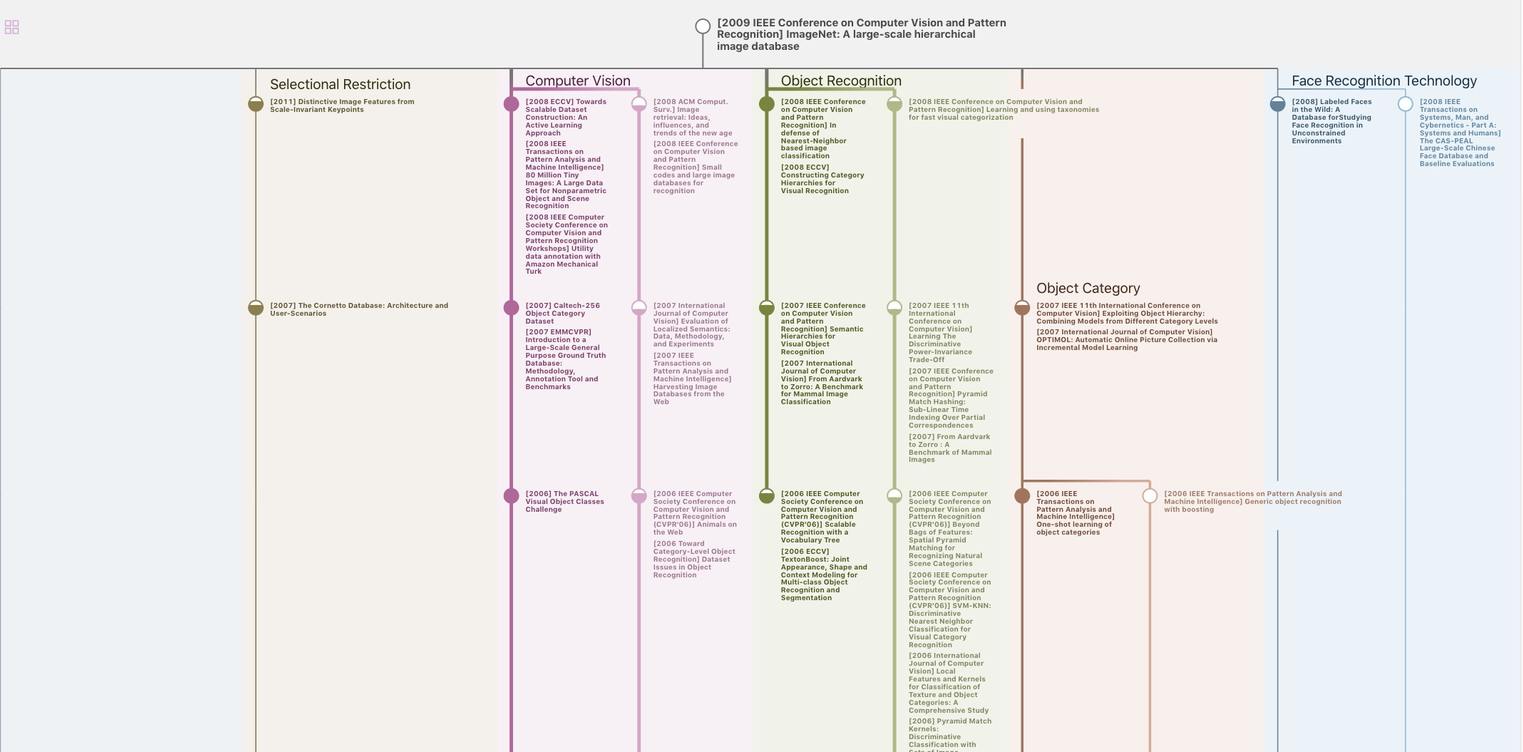
生成溯源树,研究论文发展脉络
Chat Paper
正在生成论文摘要