Incorporating multi-interest into recommendation with graph convolution networks
INTERNATIONAL JOURNAL OF INTELLIGENT SYSTEMS(2022)
摘要
In recent years, the appearance of graph convolutional networks (GCNs) provides a new idea for graph structure data processing. Because of that, they can learn excellent user and item embedding by using cooperative signals of high-order neighbors, and the GCNs technique shows great potential in the recommendation. The common problem with the bulk of GCN-based models is that it appears the situation of performance degradation during the stacking of network layers. The recently proposed IMP-GCN alleviates this problem to some extent. It aims to avoid the influence of downside information from high-order propagation on embedding learning. However, we consider that it ignores the multi-interest factor, in which users may have different interests. In this paper, we present a multi-interest GCN(MI-GCN) model for a recommendation, and it conducts high-order graph convolution operations in three sets of subgraphs. Users with similar interests and the corresponding interaction items belong to the identical subgraph. As for the formation of the subgraph, we adopt two varied clustering methods and the user feature to form a subgraph generation mechanism. This mechanism can generate three groups of differential subgraphs to divide users into multi-interest groups and make subgraph division more reasonable. We carry out massive experiments on three real-world datasets, demonstrating the effectiveness of our model. Experimental results confirm that our presented MI-GCN outperforms the state-of-the-art GCN-based recommendation models.
更多查看译文
关键词
cluster, graph convolution networks, multi-interest, recommendation, subgraph
AI 理解论文
溯源树
样例
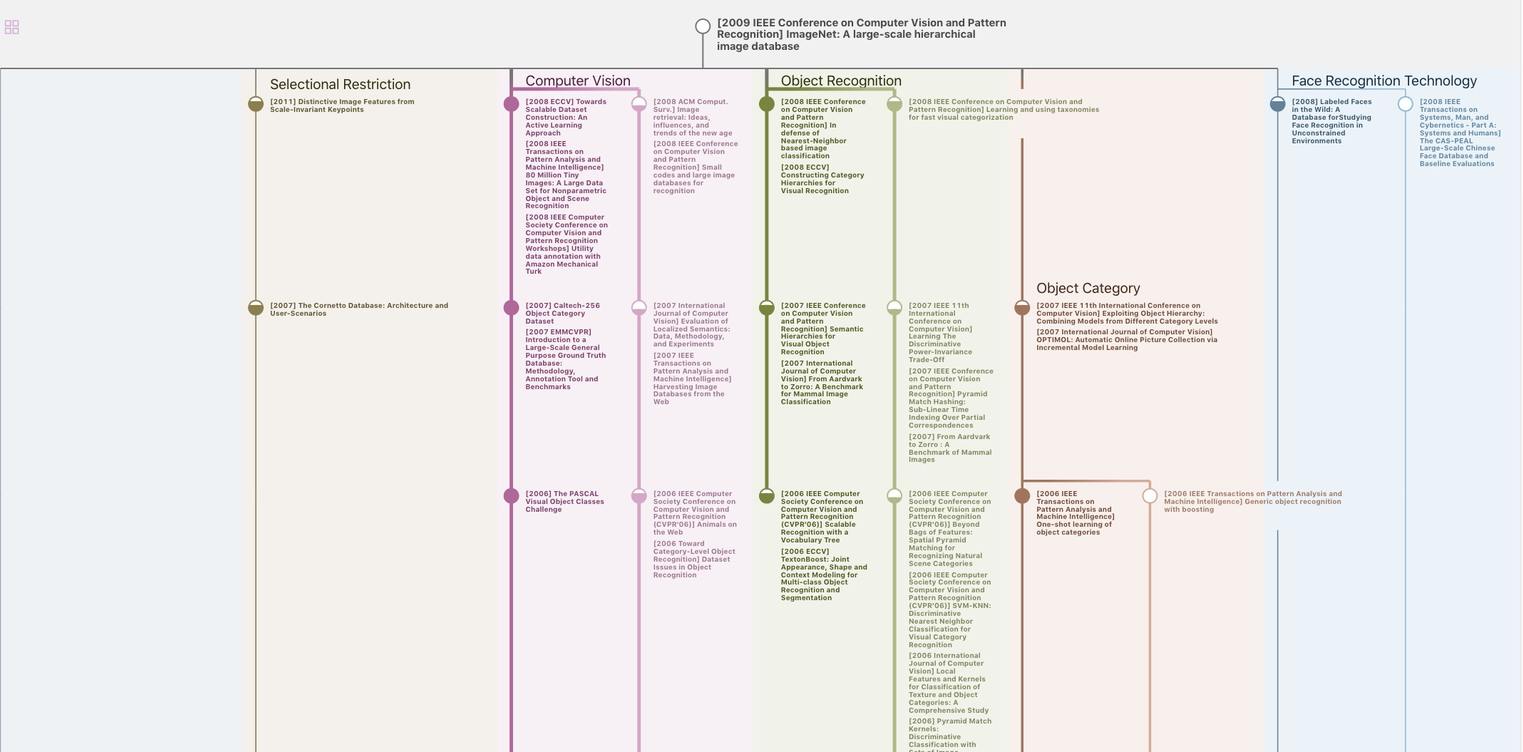
生成溯源树,研究论文发展脉络
Chat Paper
正在生成论文摘要