A Universal Decoupled Training Framework for Human Parsing
Sensors (Basel, Switzerland)(2022)
摘要
Human parsing is an important technology in human-robot interaction systems. At present, the distribution of multi-category human parsing datasets is unbalanced, and the samples present a long-tailed distribution, which directly affects the performance of human parsing. Meanwhile, the similarity between different categories leads the model to predict false parsing results. To solve the above problems, a general decoupled training framework called Decoupled Training framework based on Pixel Resampling (DTPR) was proposed to solve the long-tailed distribution, and a new sampling method named Pixel Resampling based on Accuracy distribution (PRA) for semantic segmentation was also proposed and applied to this decoupled training framework. The framework divides the training process into two phases, the first phase is to improve the model feature extraction ability, and the second phase is to improve the performance of the model on tail categories. The training framework was evaluated in MHPv2.0 and LIP datasets, and tested in both high-precision and real-time SOTA models. The MPA metric of model trained by DTPR in above two datasets increased by more than 6%, and the mIoU metric increased by more than 1% without changing the model structure.
更多查看译文
关键词
pixel resampling, long-tailed distribution, human parsing, semantic segmentation
AI 理解论文
溯源树
样例
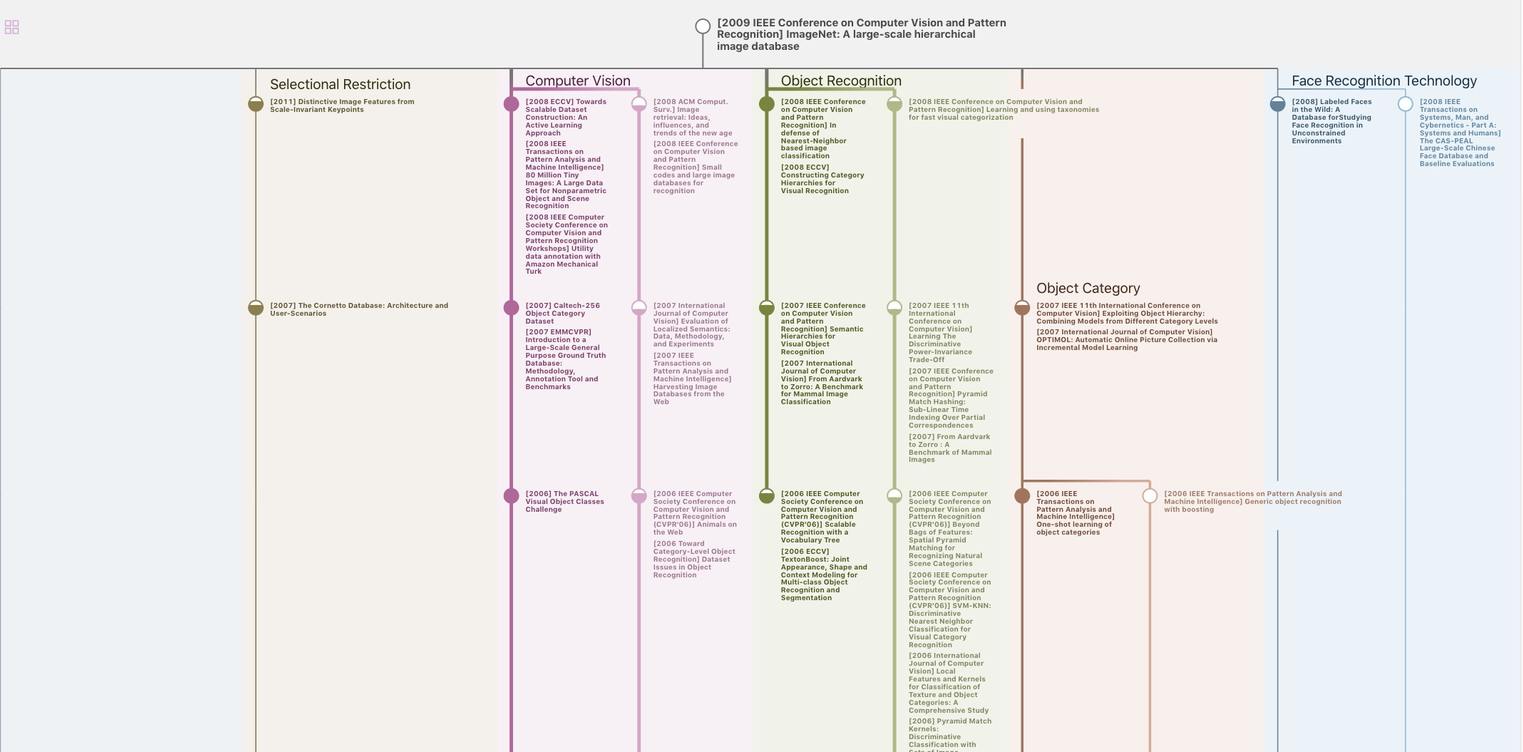
生成溯源树,研究论文发展脉络
Chat Paper
正在生成论文摘要