(Explainable) Artificial Intelligence in Aerospace Safety-Critical Systems
2022 IEEE Aerospace Conference (AERO)(2022)
摘要
AI techniques, encompassing machine learning, have made enormous progress over the last decade with several models already implemented across various aerospace applications, such as, aircraft design, operation, production and maintenance, as well as air traffic control. But the question is: Are there any behaviour validations of such AI models which could help establish assurances that they will continue to perform as specified when deployed in a real-time environment? With Explainable AI (XAI), a sub-field of AI, possibilities of exposing complex AI models to human users/operators in an interpretable and understandable ways are opening. This paper tends to explore valid answers to such questions that perplex the Aerospace AI community in fully capturing the essence of complex AI models (the black boxes) through various known XAI approaches and classes. Accordingly, various techniques, for instance, white-box AI, Black-box AI, model agnostic, fuzzy logic, and knowledge graphs, are investigated to find their efficacy levels in terms of explainability. In addition, the XAI requirements are clearly laid down for safety-critical systems from the perspective of creators, guarantors, and interpreters. Finally, this paper puts forth a comparison of various degrees of explain ability with the standard elements of Intelligence Community Directive (ICD) to set out the capabilities of XAI that would be required to build trust in complex AI models.
更多查看译文
关键词
complex AI models,white-box AI,black-box AI,fuzzy logic,aerospace safety-critical systems,AI techniques,aerospace applications,explainable AI,aerospace AI community,explainable artificial intelligence,XAI,knowledge graphs,intelligence community directive,ICD
AI 理解论文
溯源树
样例
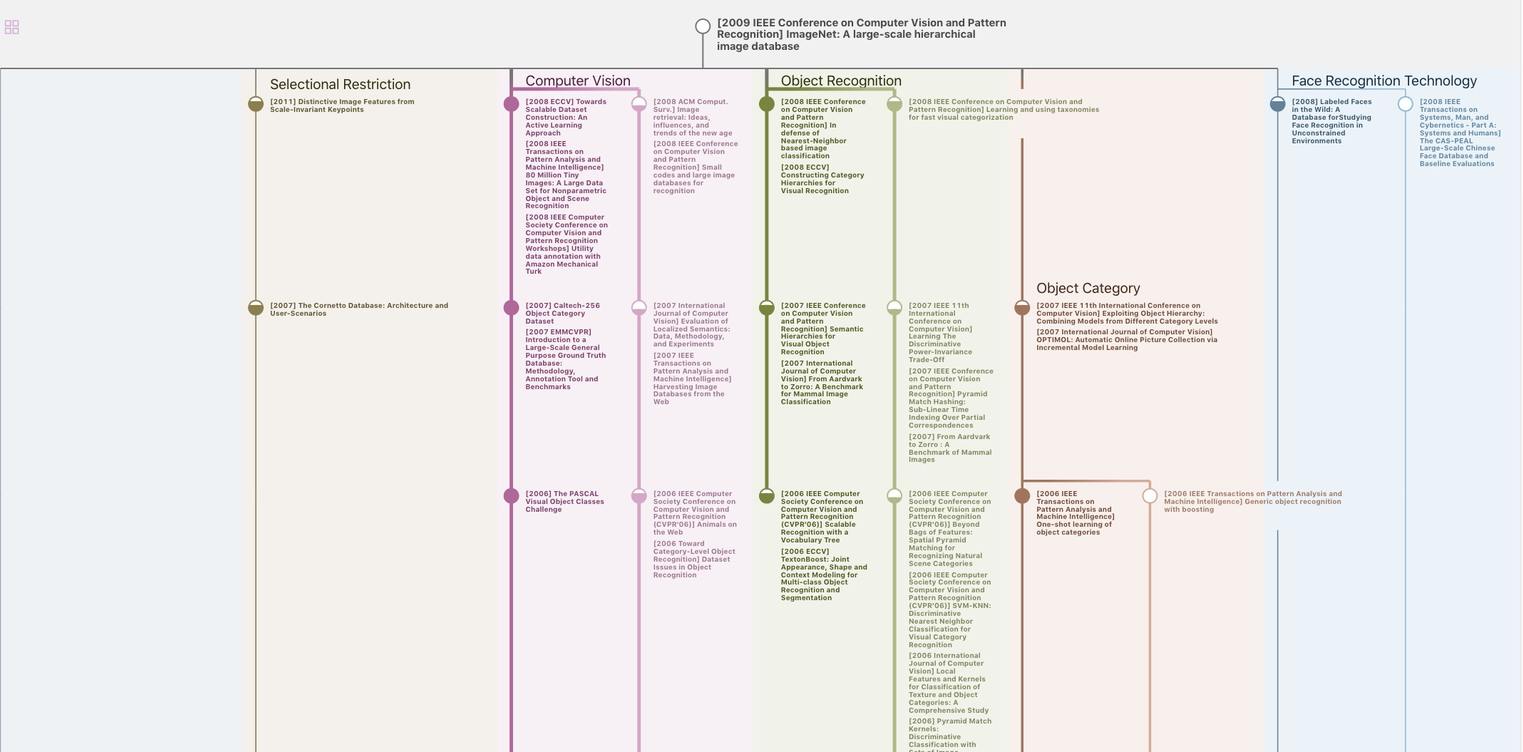
生成溯源树,研究论文发展脉络
Chat Paper
正在生成论文摘要