A Unified Comparison of User Modeling Techniques for Predicting Data Interaction and Detecting Exploration Bias
IEEE Transactions on Visualization and Computer Graphics(2023)
摘要
The visual analytics community has proposed several user modeling algorithms to capture and analyze users' interaction behavior in order to assist users in data exploration and insight generation. For example, some can detect exploration biases while others can predict data points that the user will interact with before that interaction occurs. Researchers believe this collection of algorithms can help create more intelligent visual analytics tools. However, the community lacks a rigorous evaluation and comparison of these existing techniques. As a result, there is limited guidance on which method to use and when. Our paper seeks to fill in this missing gap by comparing and ranking eight user modeling algorithms based on their performance on a diverse set of four user study datasets. We analyze exploration bias detection, data interaction prediction, and algorithmic complexity, among other measures. Based on our findings, we highlight open challenges and new directions for analyzing user interactions and visualization provenance.
更多查看译文
关键词
Visual Analytics,Analytic Provenance,User Interaction Modeling,Machine Learning,Benchmark Study
AI 理解论文
溯源树
样例
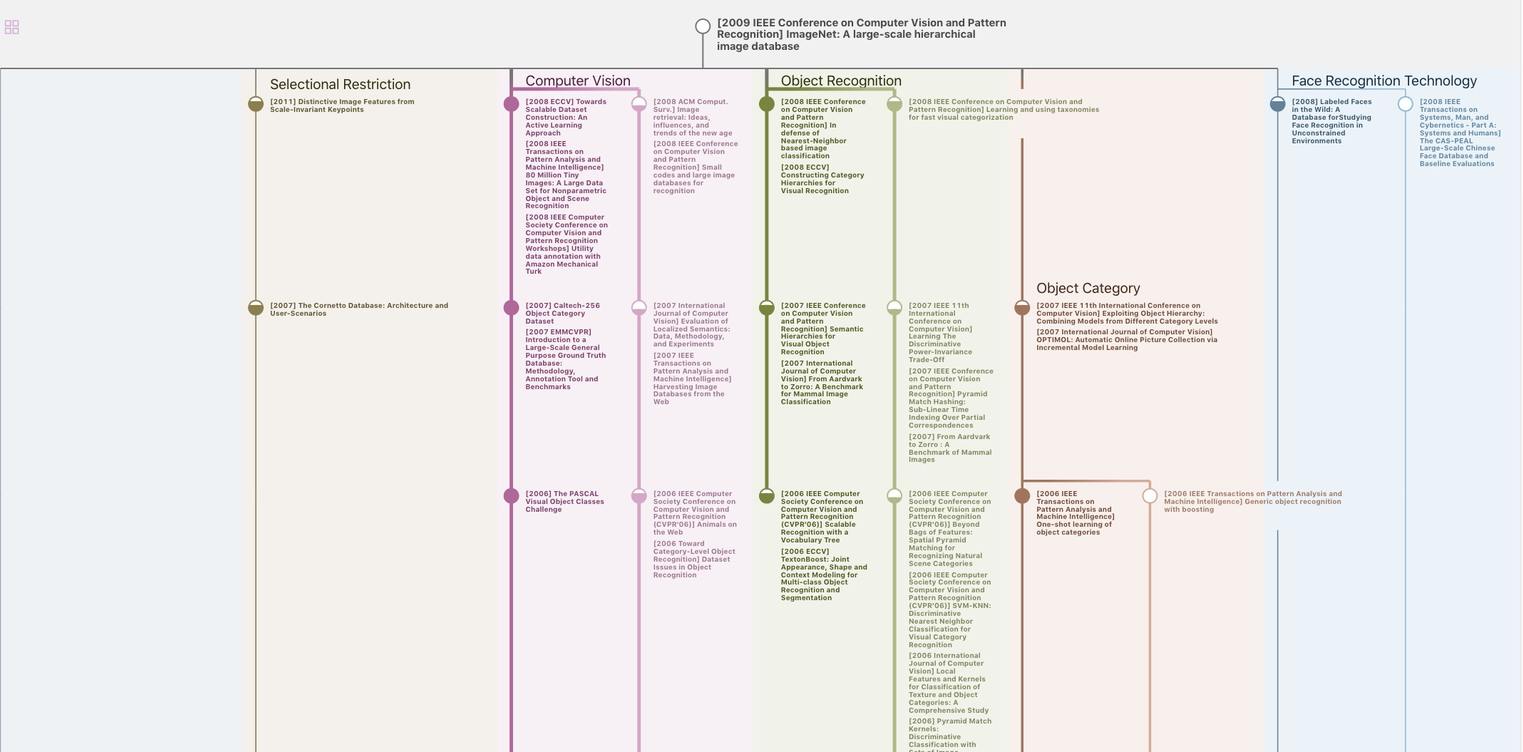
生成溯源树,研究论文发展脉络
Chat Paper
正在生成论文摘要