Locally adaptive Bayesian isotonic regression using half shrinkage priors
SCANDINAVIAN JOURNAL OF STATISTICS(2024)
摘要
Isotonic regression or monotone function estimation is a problem of estimating function values under monotonicity constraints, which appears naturally in many scientific fields. This paper proposes a new Bayesian method with global-local shrinkage priors for estimating monotone function values. Specifically, we introduce half shrinkage priors for positive valued random variables and assign them for the first-order differences of function values. We also develop fast and simple Gibbs sampling algorithms for full posterior analysis. By incorporating advanced shrinkage priors, the proposed method is adaptive to local abrupt changes or jumps in target functions. We show this adaptive property theoretically by proving that the posterior mean estimators are robust to large differences and that asymptotic risk for unchanged points can be improved. Finally, we demonstrate the proposed methods through simulations and applications to a real data set.
更多查看译文
关键词
horseshoe prior,isotonic regression,monotone curve estimation,tail robustness
AI 理解论文
溯源树
样例
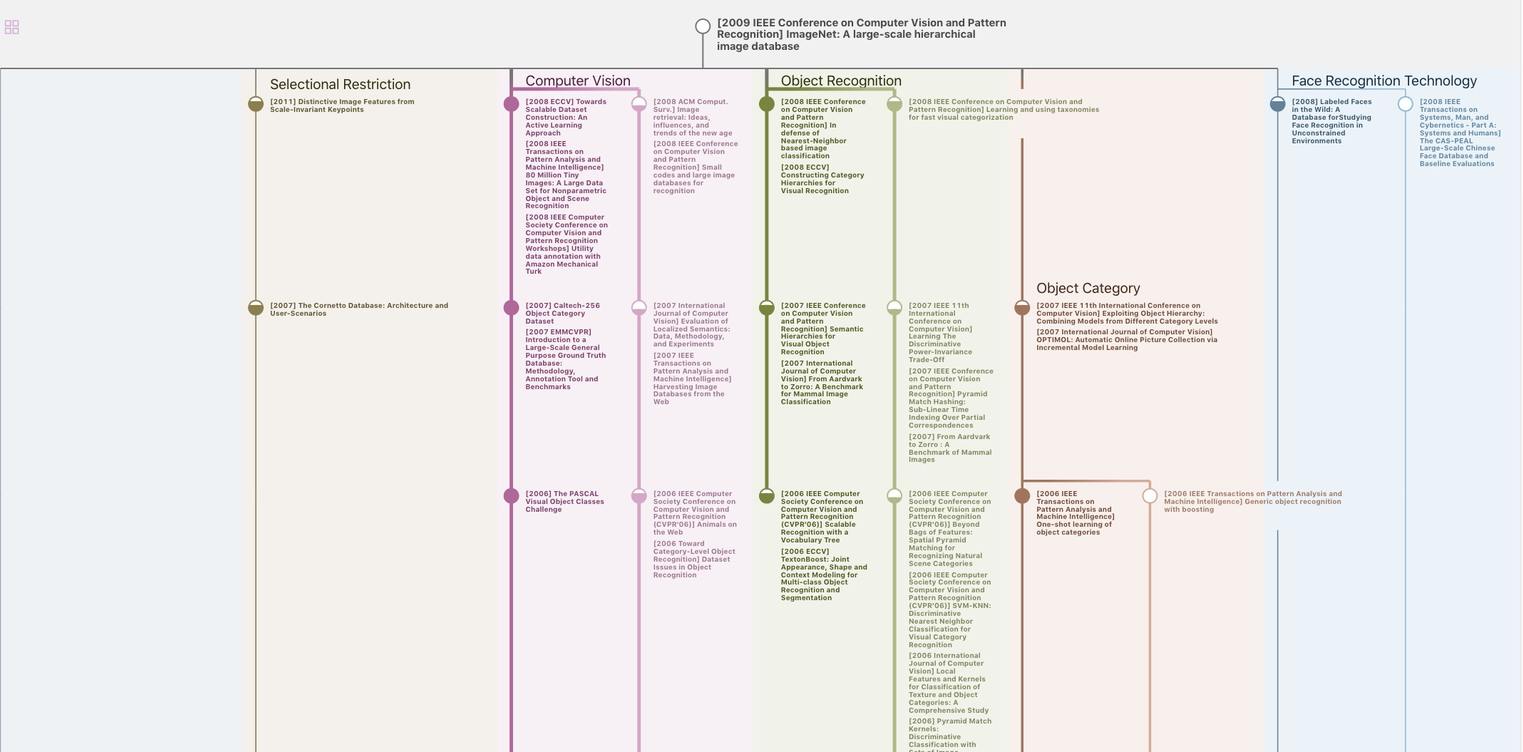
生成溯源树,研究论文发展脉络
Chat Paper
正在生成论文摘要