Local Differentially Private Fuzzy Counting in Stream Data using Probabilistic Data Structure
arxiv(2022)
摘要
Privacy-preserving estimation of counts of items in streaming data finds applications in several real-world scenarios including word auto-correction and traffic management applications. Recent works of RAPPOR and Apple's count-mean sketch (CMS) algorithm propose privacy preserving mechanisms for count estimation in large volumes of data using probabilistic data structures like counting Bloom filter and CMS. However, these existing methods fall short in providing a sound solution for real-time streaming data applications. In this work, we propose a novel (local) Differentially private mechanism that provides high utility for the streaming data count estimation problem with similar or even lower privacy budgets while providing: a) fuzzy counting to report counts of related or similar items (for instance to account for typing errors and data variations), and b) improved querying efficiency to reduce the response time for real-time querying of counts. We provide formal proofs for privacy and utility guarantees and present extensive experimental evaluation of our algorithm using real and synthetic English words datasets for both the exact and fuzzy counting scenarios. Our privacy preserving mechanism substantially outperforms the prior work in terms of lower querying time, significantly higher utility (accuracy of count estimation) under similar or lower privacy guarantees, at the cost of communication overhead.
更多查看译文
关键词
Local differential privacy,fuzzy counting,real-time querying,Cuckoo filter,Bloom filter,data streams
AI 理解论文
溯源树
样例
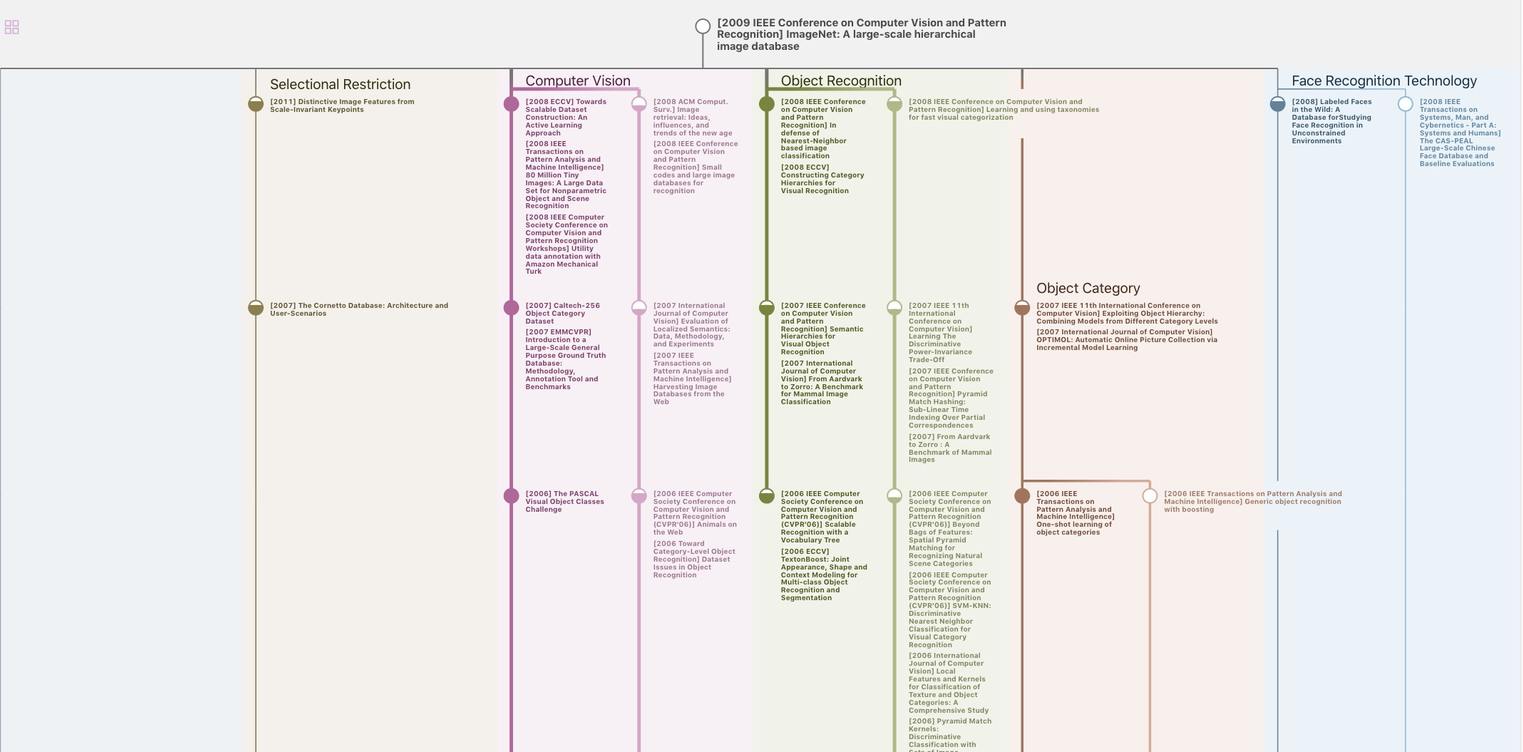
生成溯源树,研究论文发展脉络
Chat Paper
正在生成论文摘要