Accelerated Radiation Test on Quantized Neural Networks trained with Fault Aware Training
2022 IEEE Aerospace Conference (AERO)(2022)
摘要
Quantized neural networks (QNNs) are increasingly considered for adoption on multiple applications, thanks to their high accuracy, but also since they allow for significantly lower compute and memory footprints. While the theory behind QNNs is achieving a high level of maturity, several new challenges have arisen during QNN deployment. Reliable and safe implementations of QNN accelerators becomes pivotal, especially when targeting safety critical applications like automotive, industrial and aerospace, requiring innovative solutions and their careful evaluation. In this work we compare the accuracy of QNNs during accelerated radiation testing when trained with different methodologies and implemented with a dataflow architecture in field programmable gate arrays (FPGA). The initial experiment shows that QNNs trained with a novel methodology, called fault-aware training (FAT), which accounts for soft errors during neural network (NN) training, makes QNNs more resilient to single-event-effects (SEEs) in FPGA.
更多查看译文
关键词
SEEs,single-event-effects,soft errors,dataflow architecture,field programmable gate arrays,neural network training,safety critical applications,QNN accelerators,QNN deployment,memory footprints,fault aware training,quantized neural networks,accelerated radiation test
AI 理解论文
溯源树
样例
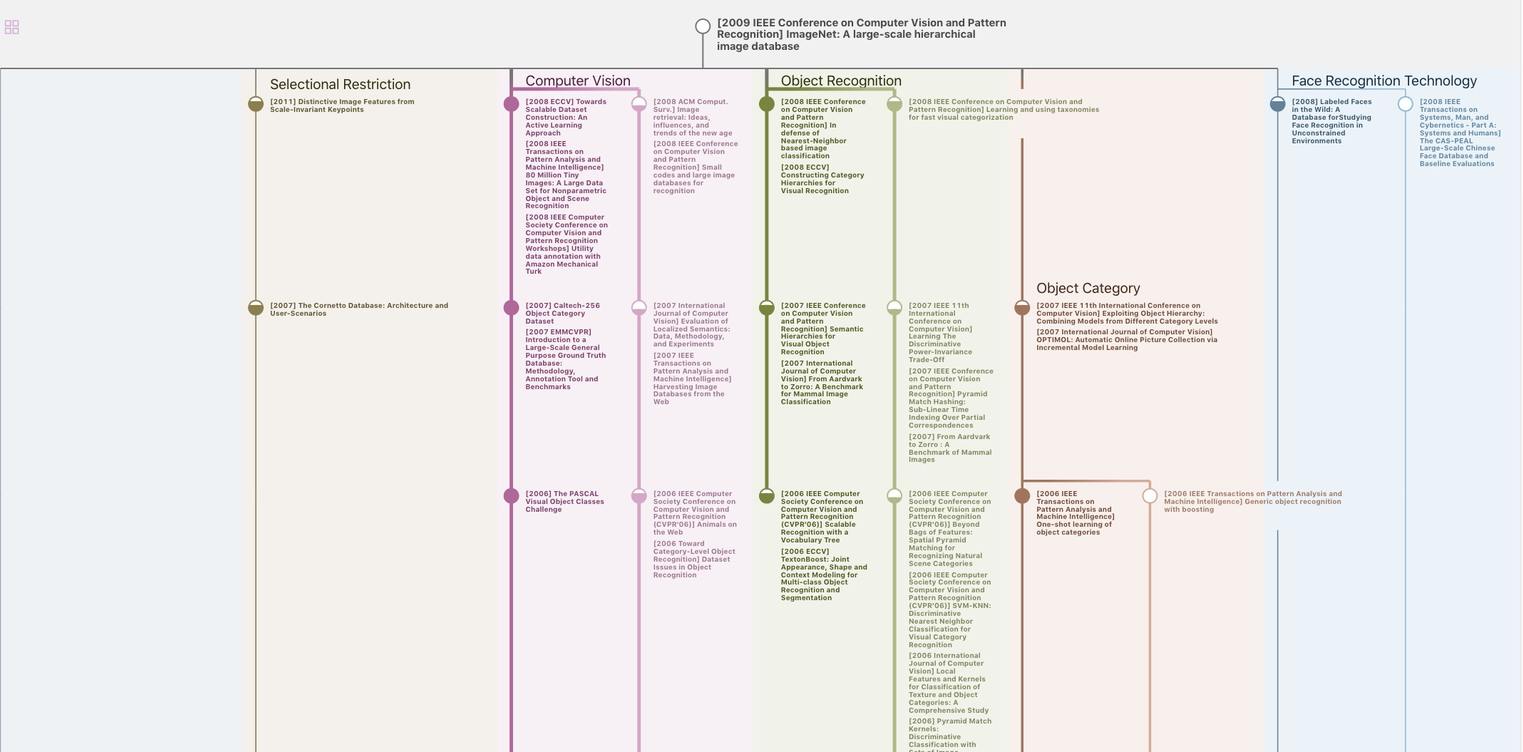
生成溯源树,研究论文发展脉络
Chat Paper
正在生成论文摘要