Return of the normal distribution: Flexible deep continual learning with variational auto-encoders
Neural Networks(2022)
摘要
Learning continually from sequentially arriving data has been a long standing challenge in machine learning. An emergent body of deep learning literature suggests various solutions, through introduction of significant simplifications to the problem statement. As a consequence of a growing focus on particular tasks and their respective benchmark assumptions, these efforts are thus becoming increasingly tailored to specific settings. Whereas approaches that leverage Variational Bayesian techniques seem to provide a more general perspective of key continual learning mechanisms, they however entail their own caveats. Inspired by prior theoretical work on solving the prevalent mismatch between prior and aggregate posterior in deep generative models, we return to a generic variational auto-encoder based formulation and investigate its utility for continual learning. Specifically, we propose to adapt a two-stage training framework towards a context conditioned variant for continual learning, where we then formulate mechanisms to alleviate catastrophic forgetting through choices of generative rehearsal or well-motivated extraction of data exemplar subsets. Although the proposed generic two-stage variational auto-encoder is not tailored towards a particular task and allows for flexible amounts of supervision, we empirically demonstrate it to surpass task-tailored methods in both supervised classification, as well as unsupervised representation learning.
更多查看译文
关键词
Continual learning,Lifelong learning,Variational auto-encoder
AI 理解论文
溯源树
样例
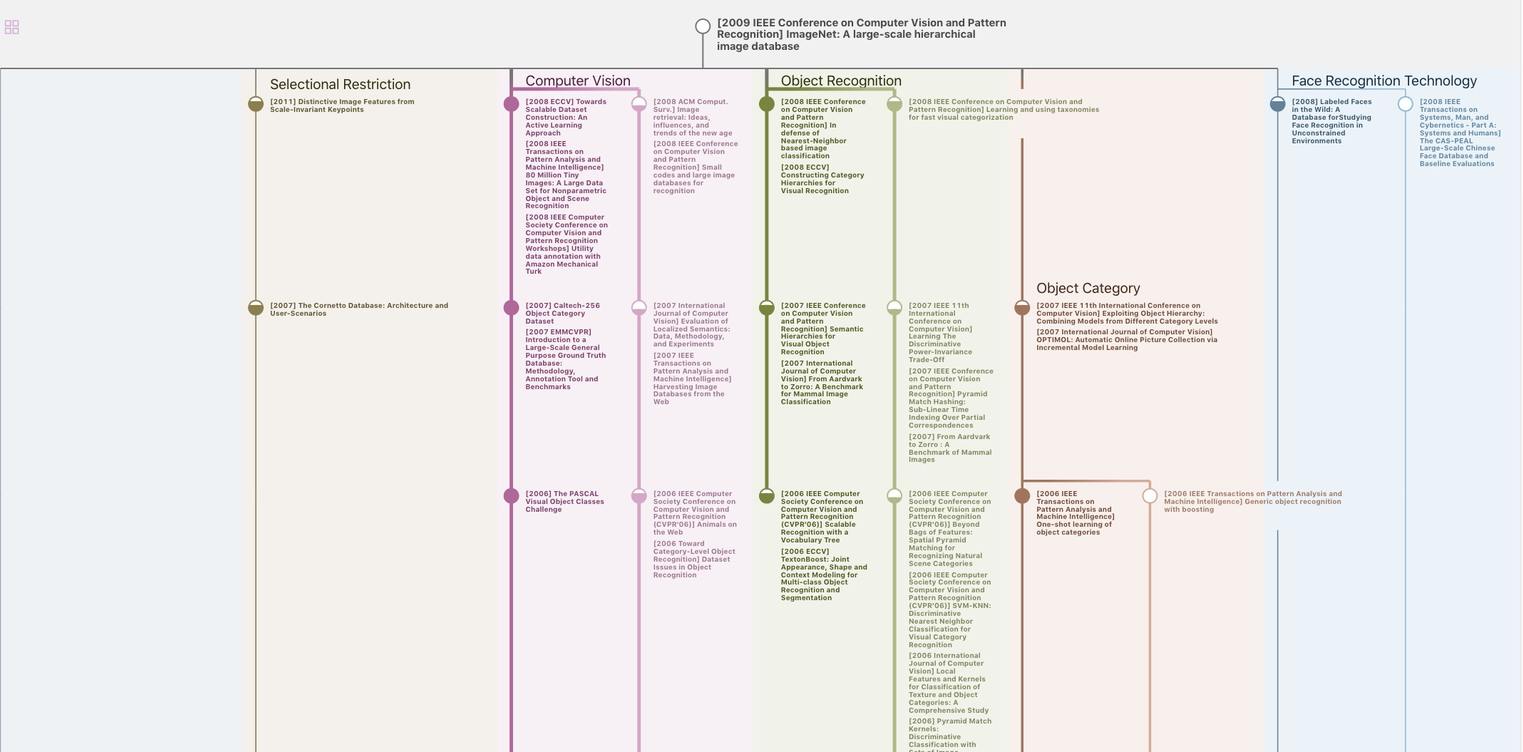
生成溯源树,研究论文发展脉络
Chat Paper
正在生成论文摘要