Temporal Self-Supervised Learning for RSSI-based Indoor Localization
ICC 2022 - IEEE International Conference on Communications(2022)
摘要
The feasibility of integrating the temporal nature of the Bluetooth Low Energy (BLE) Received Signal Strength Indicator (RSSI) into a self-supervised machine learning model for room-level and sub-room-level localization in a realistic residential setting is investigated. The signal is transmitted by a wearable wrist watch and received by multiple access points acting as receivers communicating via the BLE standard. It is found that while the baseline room-level accuracy is sufficiently high for practical applications in rooms separated by a wall, confusion can occur between non-adjacent rooms and thus lower localization performance. Two approaches are explored that exploit the time dimension of the data to mitigate this problem: maximum likelihood estimation in a conditional random field model, and self-supervised contrastive learning based on temporal proximity. Using a real world dataset collected in residential homes, we develop the approaches on the data collected in one residence before evaluating them on data collected in another. On the evaluation residence, we find that conditional random fields do not improve upon the baseline in terms of the weighted F1 score, while contrastive learning leads to an improvement in localization performance.
更多查看译文
关键词
localization,RSSI,fingerprinting,contrastive learning,e-health
AI 理解论文
溯源树
样例
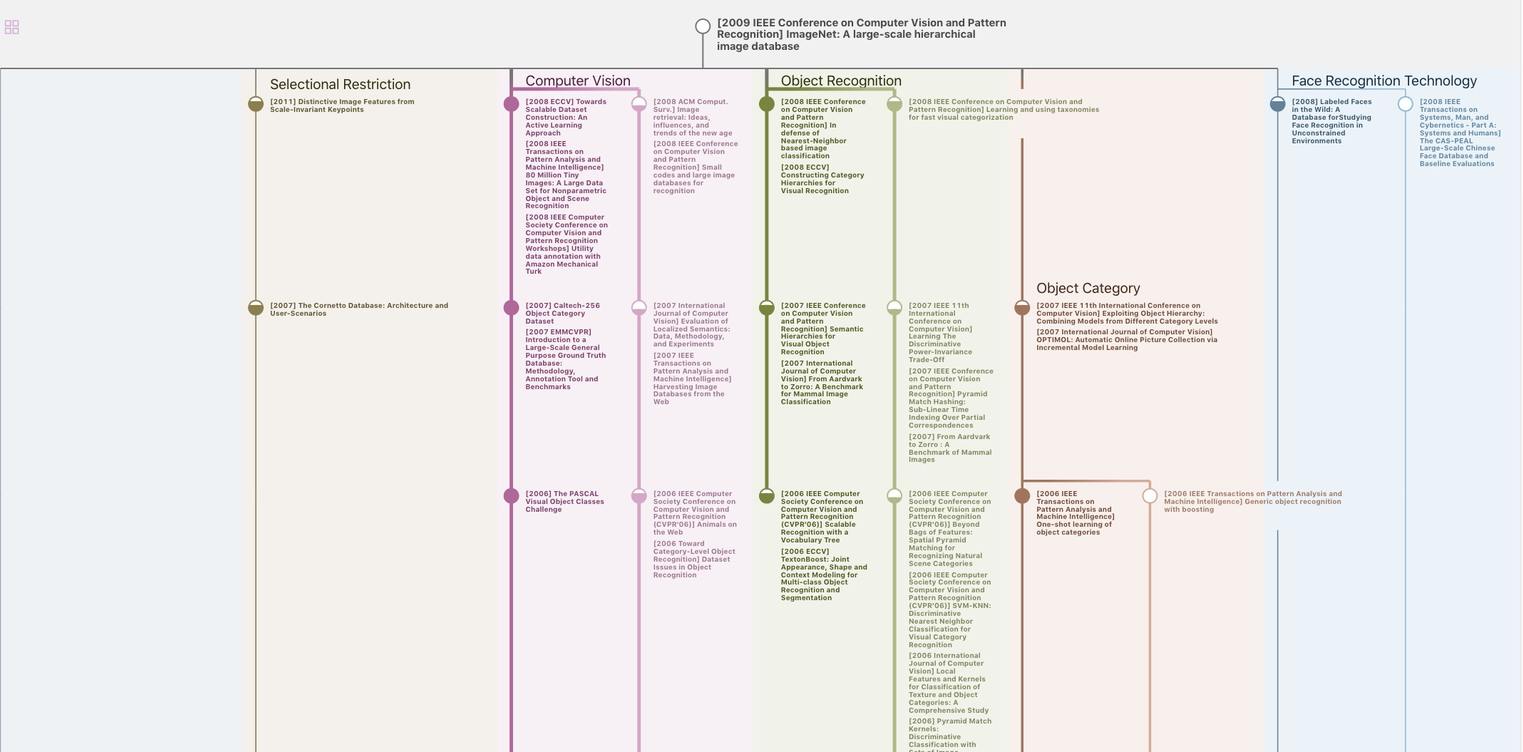
生成溯源树,研究论文发展脉络
Chat Paper
正在生成论文摘要