A novel support vector machine multi-classification strategy for power transformer fault diagnosis
2022 IEEE 5th International Electrical and Energy Conference (CIEEC)(2022)
摘要
Power transformers are considered as the important equipment in power transmission and distribution. Therefore, it is necessary to carry out monitoring and fault diagnosis for transformer. Traditional transformer fault diagnosis methods are usually based on established criteria, which are too stiff and lead to misdiagnosis of samples near the classification boundary. As an excellent pattern recognition tool, the Support Vector Machine (SVM) has been applied to transformer fault diagnosis. However, there is no targeted design for SVM multi-classification strategy at present. The direct application of traditional multi-classification strategy will lead to the difficulty in balancing training efficiency and classification accuracy. As a novel SVM multi-classification strategy for transformer fault diagnosis, the reverse merge strategy is proposed in this paper. The separability index is calculated based on within-class scatter matrix and the between-class scatter matrix. By reverse merging, the structure of classification decision tree is determined. The transformer online monitoring data provided by the State Grid Corporation of China and IEC TC 10 data are adopted for simulation verification. The simulation results demonstrate that the proposed SVM multi-classification strategy can simultaneously improve the training efficiency and classification accuracy of diagnosis model.
更多查看译文
关键词
power transformer fault diagnosis,dissolved gas analysis,support vector machine,multi-classification strategy
AI 理解论文
溯源树
样例
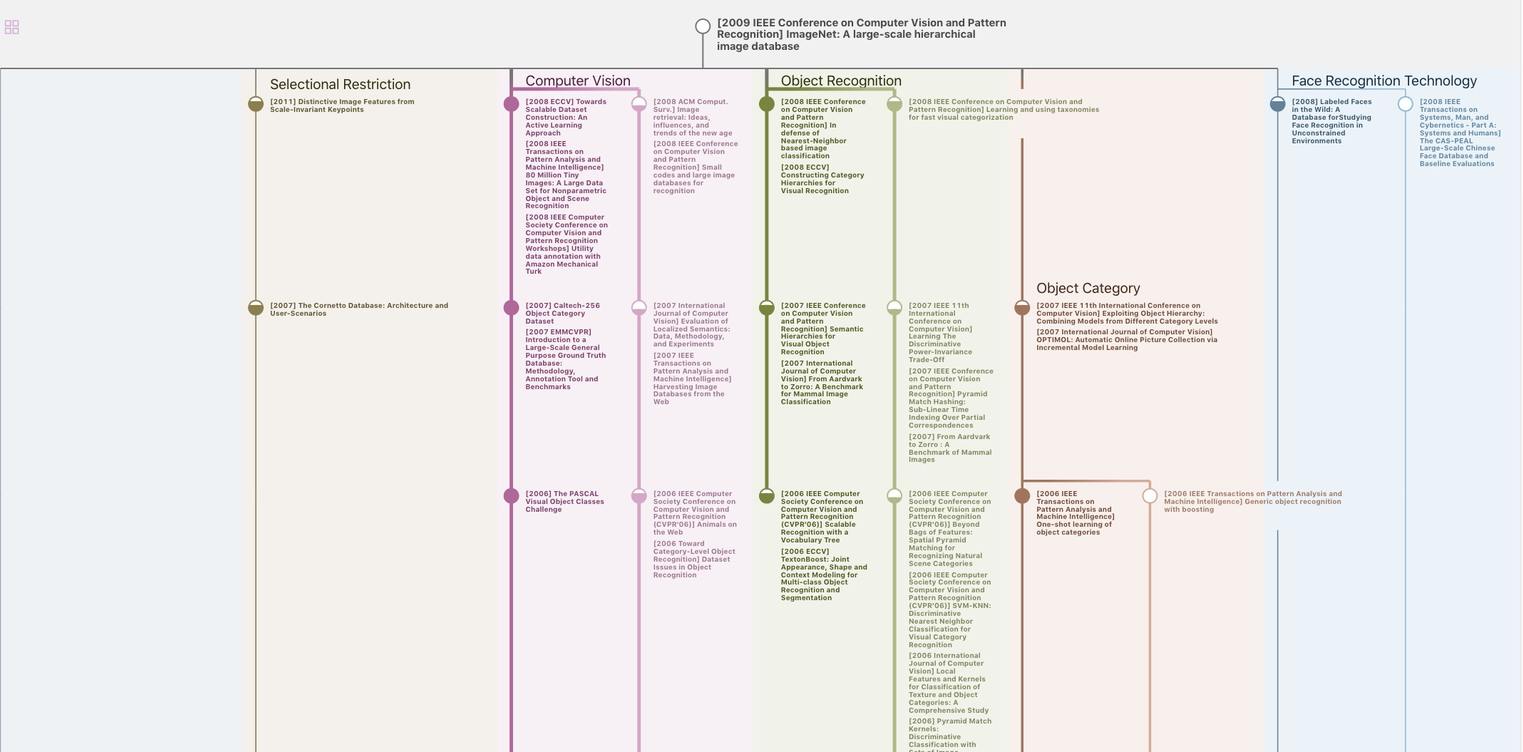
生成溯源树,研究论文发展脉络
Chat Paper
正在生成论文摘要