Comparative Analysis of Centrality Measures for Identifying Critical Nodes in Complex Networks.
Journal of computational science(2022)
摘要
One of the fundamental tasks in complex networks is detecting critical nodes whose removal significantly disrupts network connectivity. Identifying critical nodes can help analyze the topological characteristics of the network, such as vulnerability and robustness. This work considers a well-known critical node detection problem variant, Maximize the Number of Connected Components Problem, which aims to find a set of nodes whose removal maximizes the number of connected components and compares the centrality measures for detecting these nodes. While the existing literature focused only on small datasets, this work analyzes the widely used topology-based centrality measures on several synthetic and real-world networks. Our findings show that degree-like centralities are more relevant measures than path-like centralities for disconnecting networks into several connected components. However, our results also indicate that the traditional centrality measures cannot detect the most vital critical nodes. To overcome this drawback, a new centrality measure, namely Isolating Centrality, that aims to identify the nodes that significantly impact network connectedness is presented. The comprehensive computational study demonstrates that the proposed measure outperforms traditional measures in identifying critical nodes.
更多查看译文
关键词
Complex networks,Robustness,Centrality measures,Critical nodes
AI 理解论文
溯源树
样例
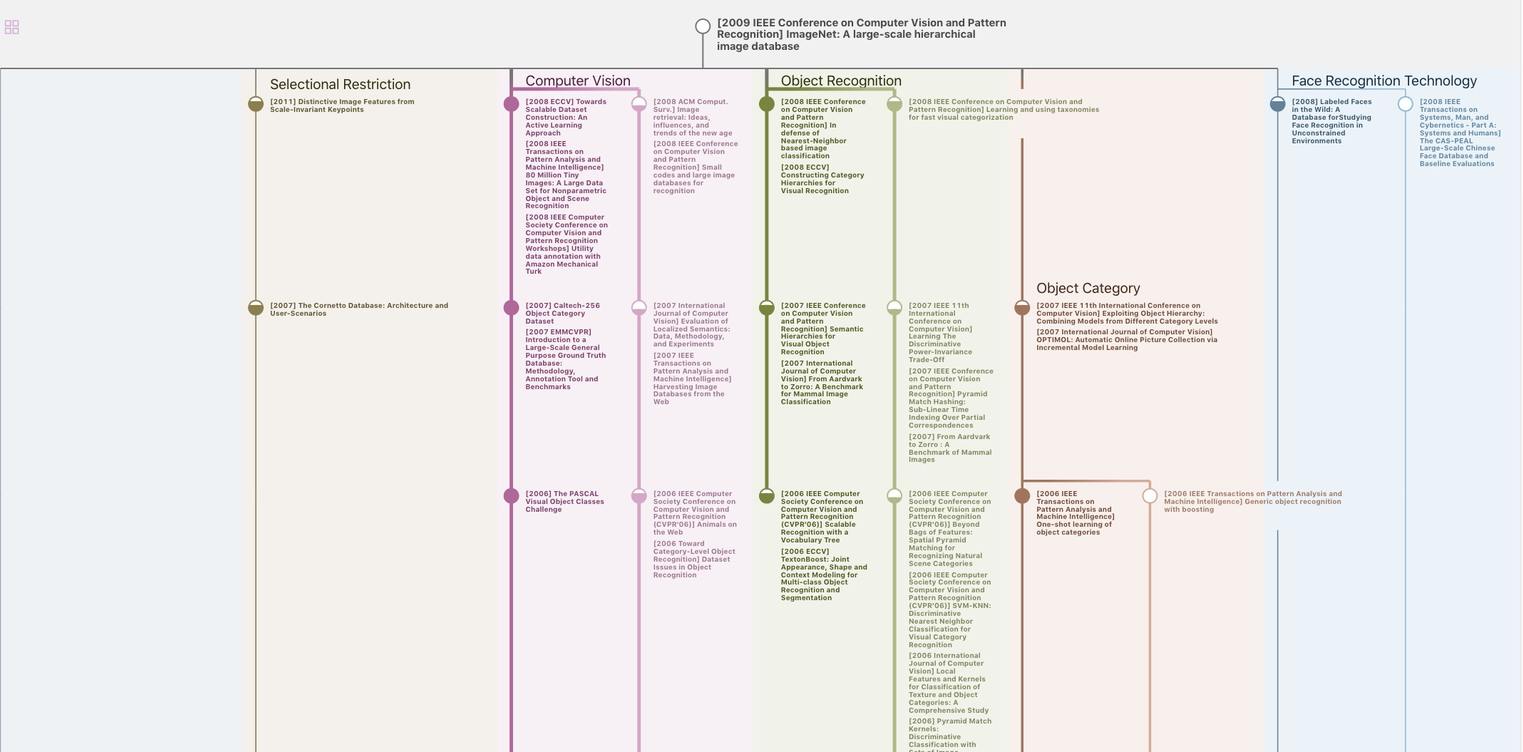
生成溯源树,研究论文发展脉络
Chat Paper
正在生成论文摘要