A medical assistant segmentation method for MRI images of osteosarcoma based on DecoupleSegNet
INTERNATIONAL JOURNAL OF INTELLIGENT SYSTEMS(2022)
摘要
Nowadays, the most common primary bone tumor is osteosarcoma, which mostly occurs in teenagers. A common diagnosis method is currently that doctors manually diagnose osteosarcoma in magnetic resonance imaging (MRI) images because it is nonradioactive and has no biological damage to brain tissue and more obvious performance in soft tissue components such as tumors, blood vessels, and muscles in MRI images. However, this method is labor-intensive and time-consuming work, and cannot guarantee the accuracy of the diagnostic results. Existing osteosarcoma MRI image segmentation methods either aim to model the global context to improve the inner consistency of objects, or multiscale feature fusion to refine the detail of objects along their boundaries, which all ignore the interaction between the body of the object and the object boundary. Therefore, this paper proposes a novel segmentation method for osteosarcoma MRI images based on DecoupleSegNet, which explores the relationship between body feature and edge feature. It can assist doctors in diagnosing osteosarcoma and improve their work efficiency. First, we warp the feature of MRI images through learning a flow field so we can make the object more consistent. We then make further work to optimize the resulting body feature and residual edge feature through explicitly sampling pixels from different parts under decoupled supervision. Through these steps, we finally obtain the final feature map with fine boundaries from the MRI image of osteosarcoma. We take a test by using more than 80,000 osteosarcoma MRI images obtained from three hospitals in China. We find that compared with existing osteosarcoma MRI image segmentation methods, our proposed method achieves 90.51 Intersection of Union % with few parameters on the test, outperforming other models. In the test, we prove that our proposed method has better accuracy and lower resource consumption.
更多查看译文
关键词
DecoupleSegNet, edge supervision, flow field, MRI image segmentation, osteosarcoma
AI 理解论文
溯源树
样例
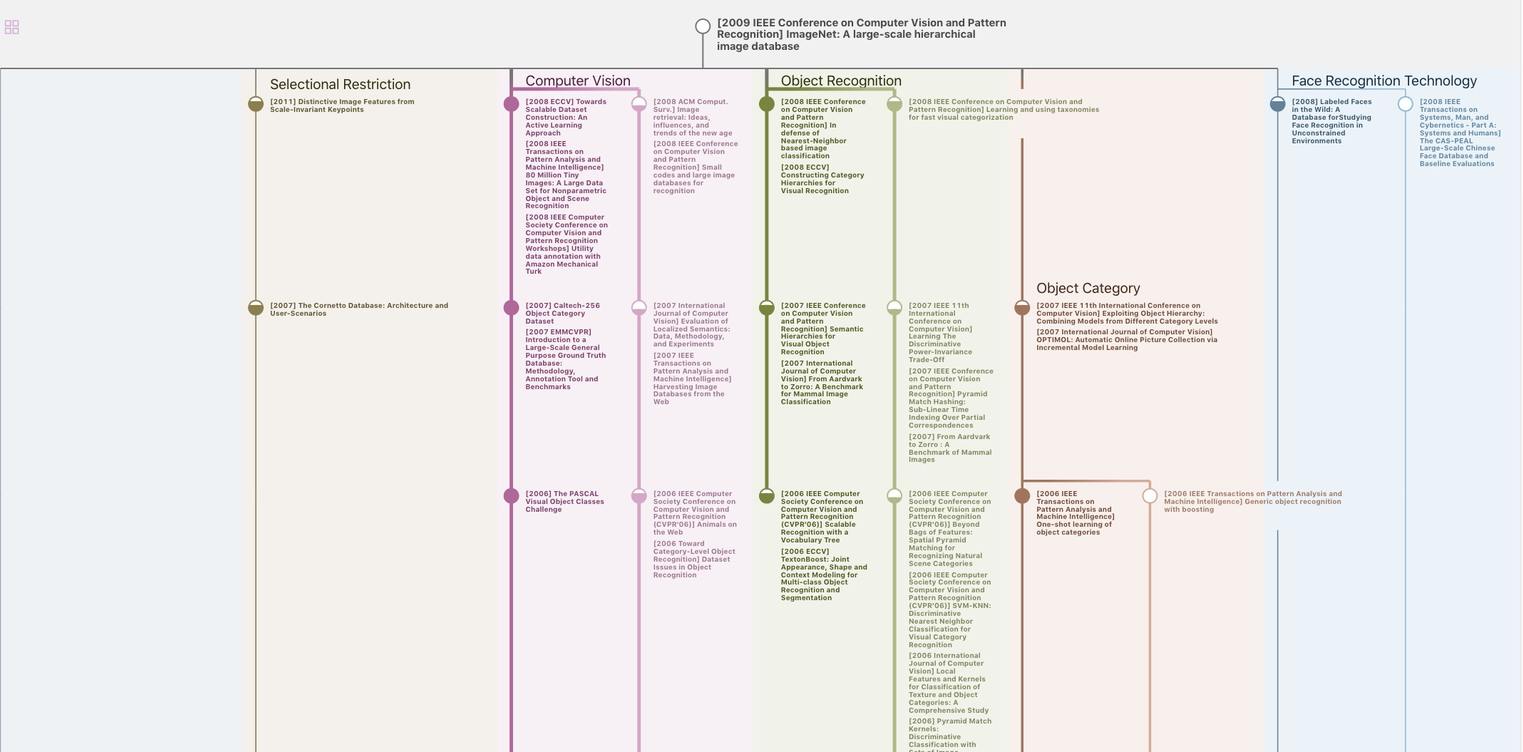
生成溯源树,研究论文发展脉络
Chat Paper
正在生成论文摘要