Moving Multi-Object Detection and Tracking Using MRNN and PS-KM Models
COMPUTER SYSTEMS SCIENCE AND ENGINEERING(2023)
摘要
On grounds of the advent of real-time applications, like autonomous driving, visual surveillance, and sports analysis, there is an augmenting focus of attention towards Multiple-Object Tracking (MOT). The tracking-by-detection paradigm, a commonly utilized approach, connects the existing recognition hypotheses to the formerly assessed object trajectories by comparing the similarities of the appearance or the motion between them. For an efficient detection and tracking of the numerous objects in a complex environment, a Pearson Similarity-centred Kuhn-Munkres (PS-KM) algorithm was proposed in the present study. In this light, the input videos were, initially, gathered from the MOT dataset and converted into frames. The background subtraction occurred which filtered the inappropriate data concerning the frames after the frame conversion stage. Then, the extraction of features from the frames was executed. Afterwards, the higher dimensional features were transformed into lower-dimensional features, and feature reduction process was performed with the aid of Information Gain-centred Singular Value Decomposition (IG-SVD). Next, using the Modified Recurrent Neural Network (MRNN) method, classification was executed which identified the categories of the objects additionally. The PS-KM algorithm identified that the recognized objects were tracked. Finally, the experimental outcomes exhibited that numerous targets were precisely tracked by the proposed system with 97% accuracy with a low false positive rate (FPR) of 2.3%. It was also proved that the present techniques viz. RNN, CNN, and KNN, were effective with regard to the existing models.
更多查看译文
关键词
Multi-object detection, object tracking, feature extraction, morlet wavelet mutation (MWM), ant lion optimization (ALO), background subtraction
AI 理解论文
溯源树
样例
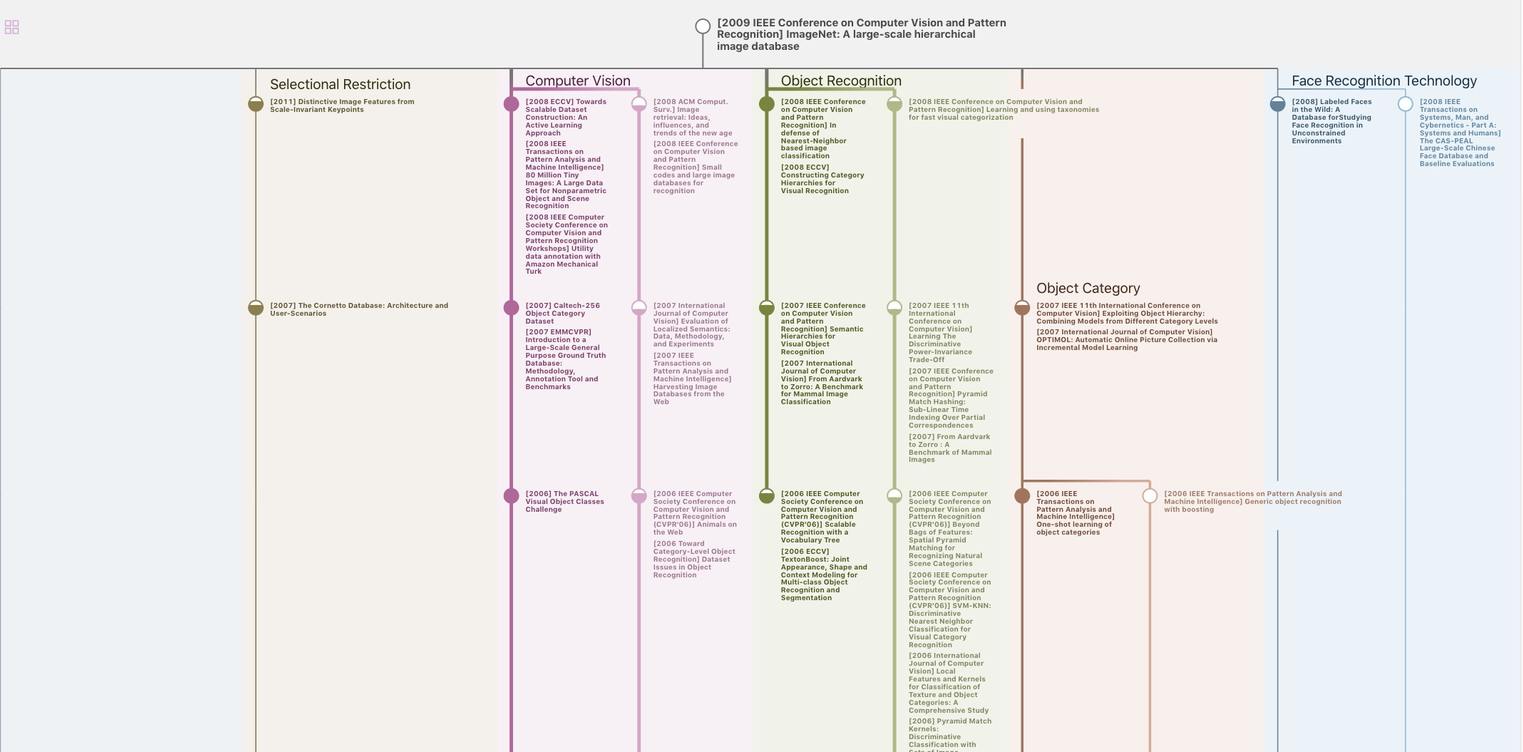
生成溯源树,研究论文发展脉络
Chat Paper
正在生成论文摘要