A novel random subspace method considering complementarity between unsupervised and supervised deep representation features for soft sensors
MEASUREMENT SCIENCE AND TECHNOLOGY(2022)
摘要
Unsupervised and supervised deep learning extract effective and abstract features from different perspectives, which have been successfully applied in soft sensors. However, few studies have fused them and explored the complementary effect between the two kinds of features, which limits the utilization of comprehensive prediction information. To address the problem, a novel random subspace method with stacked auto-encoder (SAE) and bidirectional long short-term memory (Bi-LSTM), named RS-SBL, is proposed for soft sensors. Firstly, unsupervised and supervised deep representation features are extracted by SAE and Bi-LSTM, respectively. Secondly, to leverage the complementarity of the fusion features, an improved random subspcae (RS) method with a structure sparsity learning model is designed to discriminate the relative importance of different features and generate ensemble prediction results. Finally, the experiments on two real-world industrial nonlinear processes demonstrate that the proposed RS-SBL with the feature fusion strategy improves the prediction performance, and outperforms the other comparison soft sensor models.
更多查看译文
关键词
soft sensor, ensemble learning, sparse group lasso, fusion features, deep learning
AI 理解论文
溯源树
样例
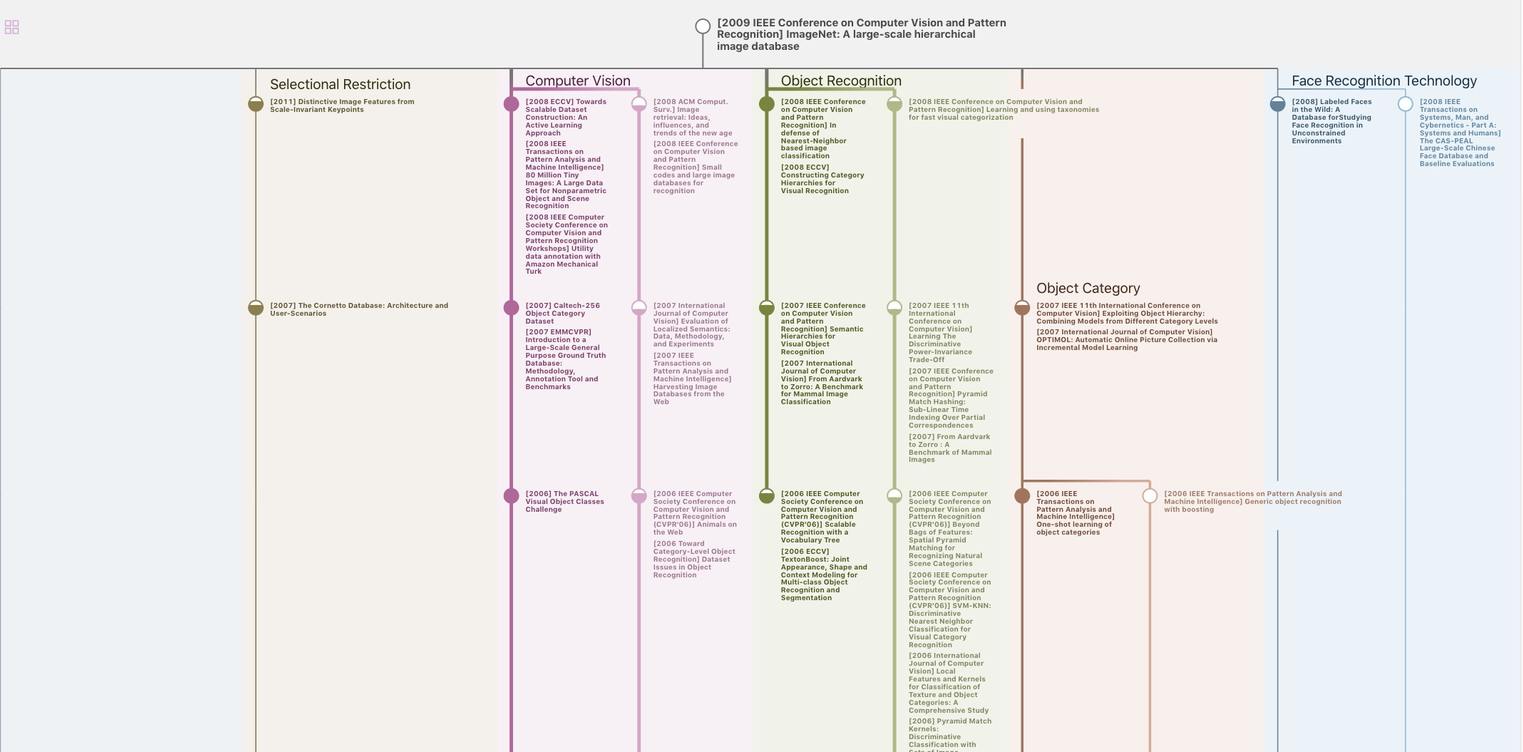
生成溯源树,研究论文发展脉络
Chat Paper
正在生成论文摘要