A computer aided diagnosis framework for detection and classification of interstitial lung diseases using computed tomography (CT) images
Applied Nanoscience(2022)
摘要
In this research work, a computer aided diagnosis (CAD) system is developed and in which the feature extraction system and the classifier are optimized for lung disease prediction. The chest CT slices that have already been diagnosed by an expert are preprocessed and the lung regions are segmented using region growing algorithm. Twenty three histogram features are computed using the Gray Level Histogram (GLH) and three features are computed using the Quincunx Wavelet Frame (QWF) decomposition. Particle Swarm Optimization (PSO) is used to optimize the order and the number of decomposition levels of the QWF. The GLH and QWF features are grouped to form a feature vector. The feature vectors that are extracted are applied to the SVM classifier. The SVM classifier learns from the feature vector space to find the decision boundaries among five classes of lung CT patterns (emphysema, fibrosis, GGO, miliary TB and normal) using a one-versus-all (OAA) approach. The PSO is used to optimize the cost function and the standard deviation of the SVM classifier. This results in an optimized SVM classifier that has been trained to differentiate between five classes of disease patterns. The results show that the use of the PSO-based optimization framework for classifying lung CT patterns resulted in significant improvement.
更多查看译文
关键词
Computer aided diagnosis,Support vector machine,Particle swarm optimization,Lung disease,Emphysema,Fibrosis
AI 理解论文
溯源树
样例
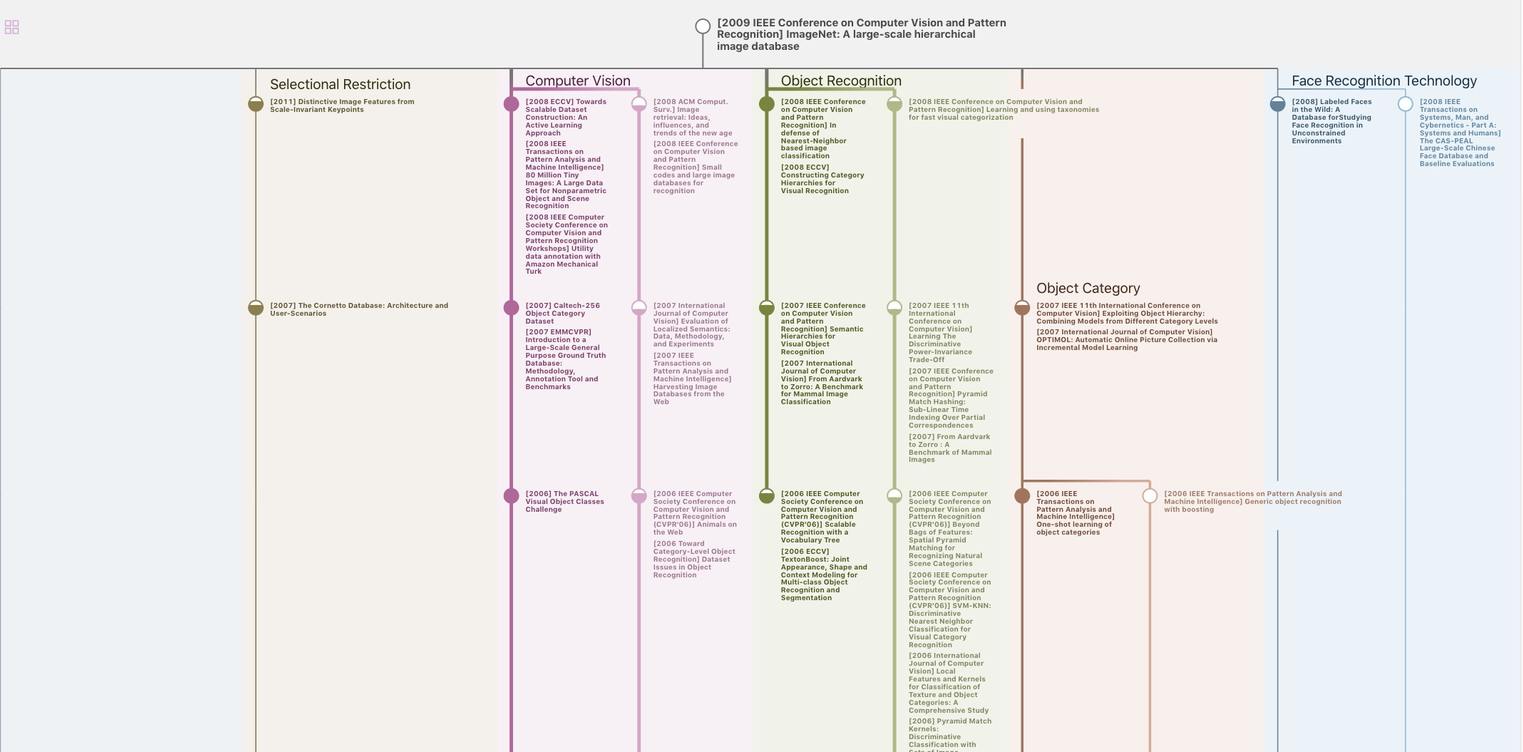
生成溯源树,研究论文发展脉络
Chat Paper
正在生成论文摘要