Balancing the trade-off between accuracy and diversity in recommender systems with personalized explanations based on Linked Open Data
Knowledge-Based Systems(2022)
摘要
Collaborative filtering recommendation algorithms generate suggestions based on similar interactions between users. Although it provides accurate recommendations, the approach has two limitations: the popularity bias, which frequently suggests a small set of the most interacted items, and the systems’ black box functioning, as they are grounded on complex mathematical models. To improve such aspects in collaborative filtering algorithms, this paper introduces a multi-domain item reordering system based on the best explanation for an item, which are the best ranked paths extracted from a Linked Open Data knowledge graph connecting recommended and interacted items. To order paths, the algorithm assigns a value to the node attributes connecting two items by calculating the popularity of the property between interacted items that are rare in the full set of items. Results from two datasets of the movie and music domains comparing the proposed reordering system with six baselines of different collaborative filtering families showed that our easy-to-explain approach improved diversity and/or accuracy metrics.
更多查看译文
关键词
Recommender systems,Collaborative filtering,Linked open data,Explainable AI
AI 理解论文
溯源树
样例
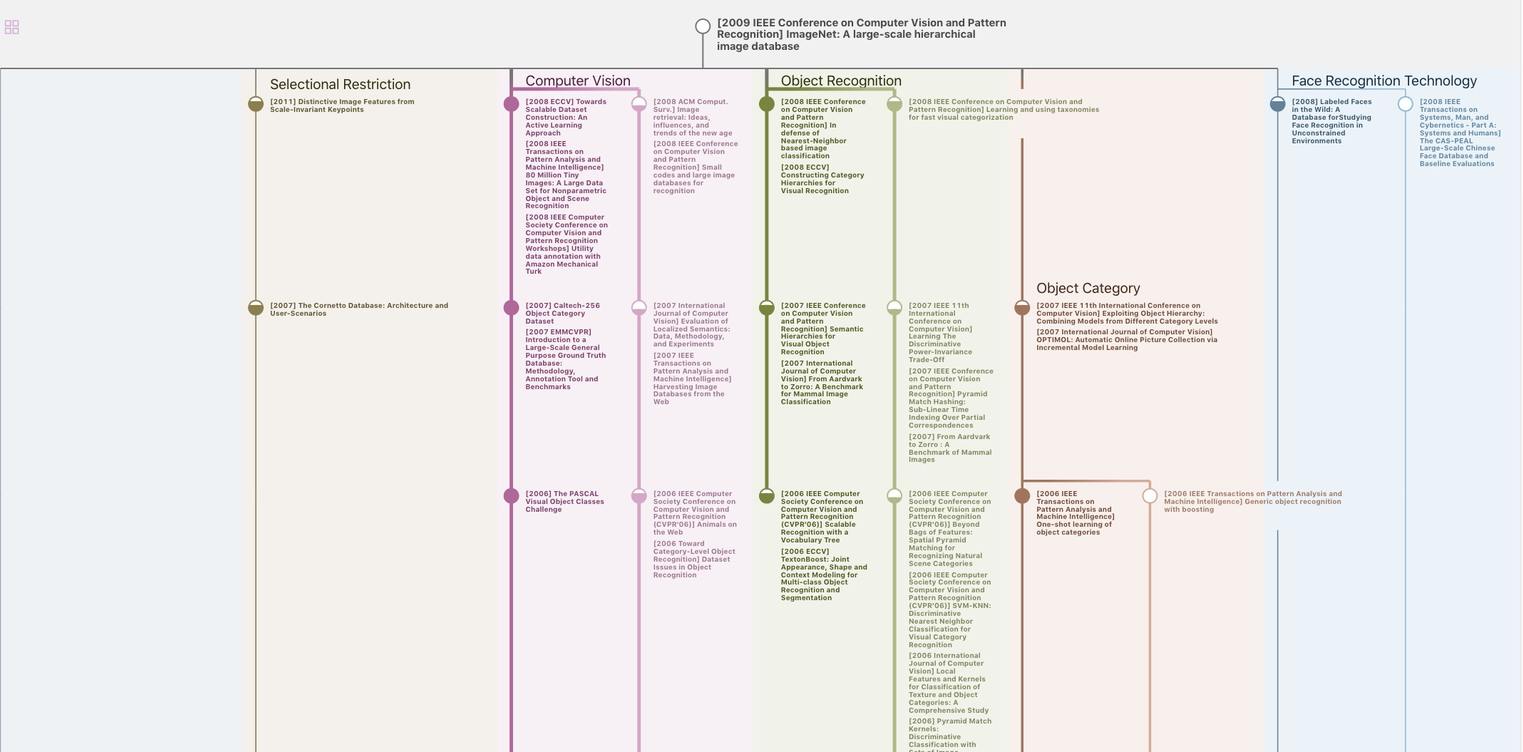
生成溯源树,研究论文发展脉络
Chat Paper
正在生成论文摘要