High-Dimensional Multi-Task Learning using Multivariate Regression and Generalized Fiducial Inference
JOURNAL OF COMPUTATIONAL AND GRAPHICAL STATISTICS(2023)
摘要
Over the past decades, the Multi-Task Learning (MTL) problem has attracted much attention in the artificial intelligence and machine learning communities. However, most published work in this area focuses on point estimation; that is, estimating model parameters and/or making predictions. This article studies another important aspect of the MTL problem: uncertainty quantification for model choices and predictions. To be more specific, this article approaches the MTL problem with multivariate regression and develops a novel method for deriving a probability density function on the space of all potential regression models. With this density function, point estimates, as well as confidence and prediction ellipsoids, can be obtained for quantities of interest, such as future observations. The proposed method, termed GMTask, is based on the generalized fiducial inference (GFI) framework and is shown to enjoy desirable theoretical properties. Its promising empirical properties are illustrated via a sequence of numerical experiments and applications to two real datasets. for this article are available online.
更多查看译文
关键词
Confidence ellipsoids, GMTask, Large p small n, Prediction ellipsoids, Uncertainty quantification
AI 理解论文
溯源树
样例
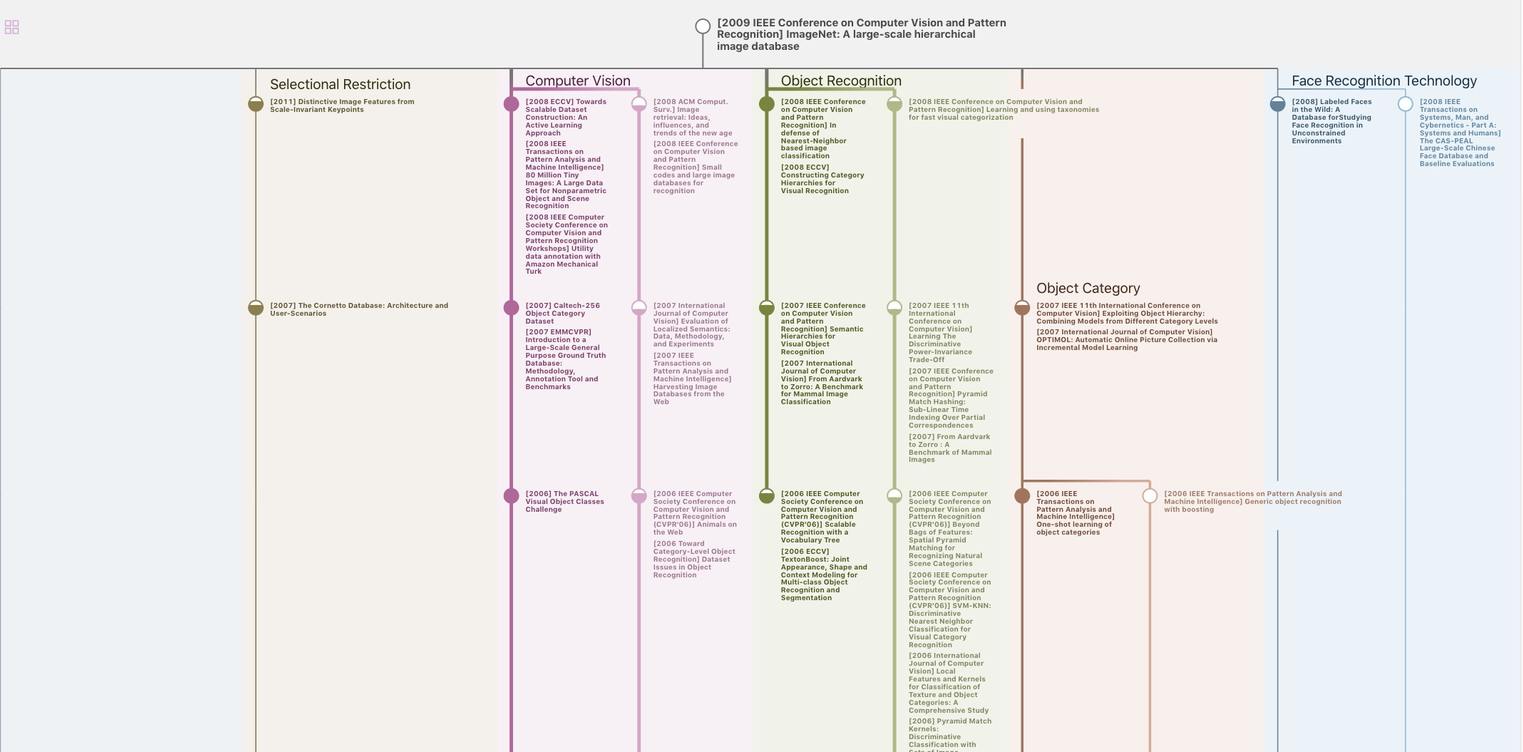
生成溯源树,研究论文发展脉络
Chat Paper
正在生成论文摘要