DSIM: dynamic and static interest mining for sequential recommendation
Knowledge and Information Systems(2022)
摘要
Sequential recommendation aims to predict the next interaction by mining users’ evolving interest from their historical behaviors. Through comprehensive study, we argue that the evolving interest consists of dynamic interest and static interest, both of which are important for recommendation tasks. However, it is still a challenging task to model the dynamic interest accurately since it may change rapidly. Besides, existing approaches cannot fully exploit users’ static interest. In this paper, we propose a novel dynamic and static interest mining approach called DSIM for sequential recommendation. Specifically, DSIM takes advantage of the time information and learn the dynamic interest precisely with a time-aware neural Hawkes process. Furthermore, a side information-aware self-attention mechanism is designed to leverage side information in a non-invasive way to learn static interest. Finally, DSIM fuses the learned dynamic and static interest adaptively with the gating mechanism and generates the hybrid interest for sequential recommendation. Extensive experiments on three real-world datasets demonstrate that DSIM can effectively mine and model users’ interest, and it outperforms the state-of-the-art baselines in sequential recommendation tasks.
更多查看译文
关键词
Sequential recommendation, Interest mining, Time-aware features, Self-attention mechanism
AI 理解论文
溯源树
样例
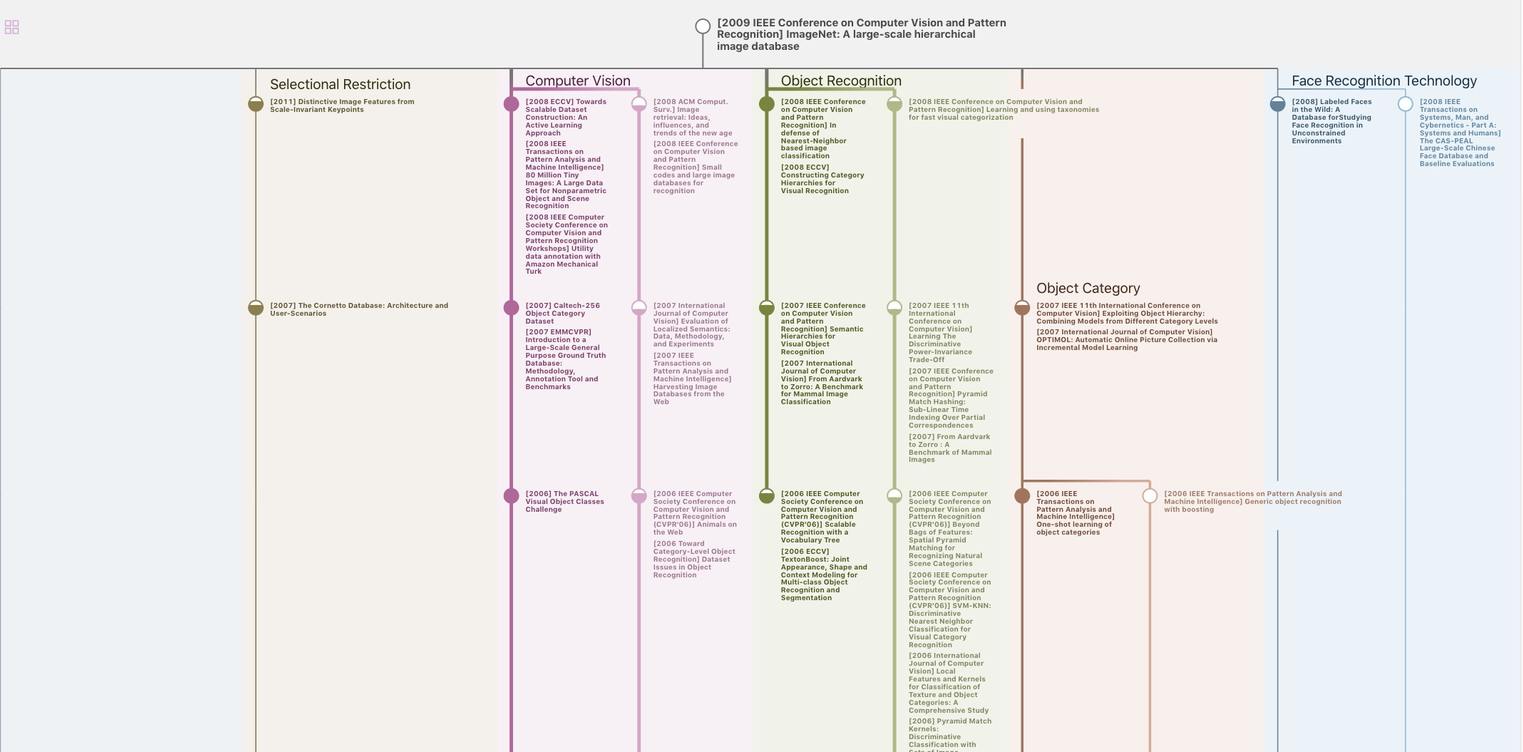
生成溯源树,研究论文发展脉络
Chat Paper
正在生成论文摘要