Temporal knowledge graph representation learning with local and global evolutions
Knowledge-Based Systems(2022)
摘要
Temporal knowledge graph (TKG) representation learning aims to project entities and relations in TKGs to a low-dimensional vector space while preserving the evolutionary nature of TKGs. Most existing methods treat knowledge that happens at different times separately, which fails to explore how temporal knowledge graphs evolve over time. Actually, TKGs should evolve both on the local and global structures. The local structure evolution describes the formation process of the graph structure in a detailed manner, while the global structure evolution refers to the dynamic topology (e.g., community partition) of the graph, which is derived from the continuous formation process. Both are key factors for understanding the evolutionary nature of the TKGs. Unfortunately, little attention has been given to this area of research. In this paper, we propose a new TKG representation learning framework with local and global structure evolutions, named EvoExplore. Specifically, we define the local structure evolution as an establishment process of the relations between the entities, and propose a hierarchical-attention-based temporal point process to capture the formation process of the graph structure in a fine-grained manner. For global structure evolution, we propose a novel soft modularity parameterized by the entity representations to capture the dynamic community partition of the TKGs. Finally, we employ a multi-task loss function to jointly optimize the above two parts, which allows EvoExplore to learn the mutual influences of the local and global structure evolutions. Experimental results on three real-world datasets demonstrate the superiority of EvoExplore compared with the baseline methods. Code is available at https://github.com/zjs123/EvoExplore and https://github.com/zjs123/EvoExplore_MindSpore.
更多查看译文
关键词
Knowledge graph,Temporal evolution,Representation learning
AI 理解论文
溯源树
样例
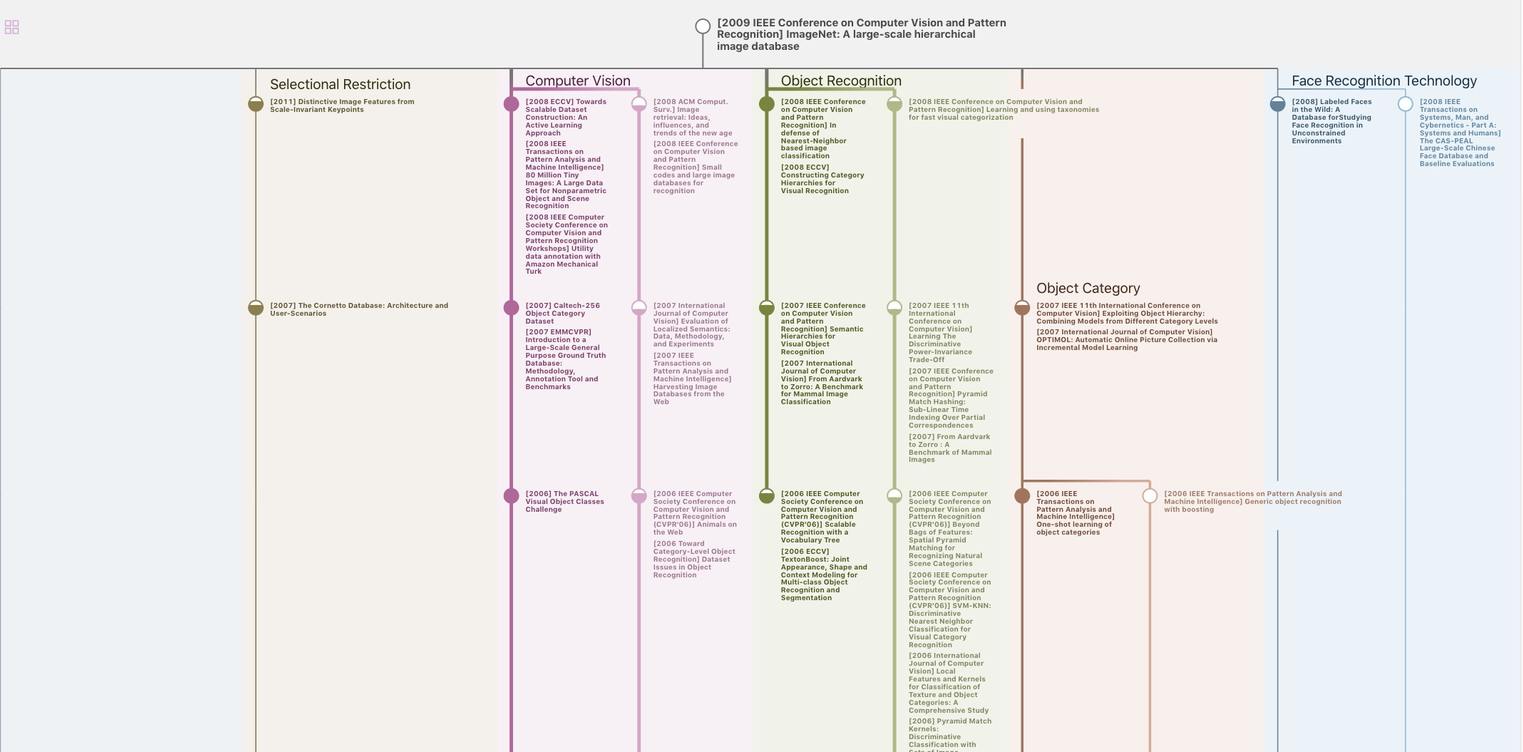
生成溯源树,研究论文发展脉络
Chat Paper
正在生成论文摘要