Scalable spatiotemporal regression model based on Moran's eigenvectors
INTERNATIONAL JOURNAL OF GEOGRAPHICAL INFORMATION SCIENCE(2023)
摘要
We propose a scalable regression model with spatially and temporally varying coefficients based on Moran's eigenvectors and efficient computation algorithms. Regression models that consider spatiotemporal non-stationarity are important because many real-world datasets, such as housing prices, are tied to geographical and temporal locations. Although geographically weighted regression (GWR) and its variants are widely used to model spatially varying coefficients, they cannot handle large datasets. We employ an alternative modelling method of spatially varying coefficients based on Moran's eigenvectors and extend it to handle large spatiotemporal datasets. Additionally, we introduce a scalable learning algorithm that exploits the model structures based on the Kalman filter and the expectation-maximisation algorithm. Our scalable algorithm is efficient even for large datasets that cannot be handled by GWR. To evaluate the performance of the proposed model, we applied it to a housing market dataset collected in Tokyo, Japan. The results show that the predictive performance of the proposed model is comparable to that of GWR while increasing the computational speed. Moreover, larger datasets can accelerate the algorithm convergence.
更多查看译文
关键词
Eigenvector spatial filtering,spatiotemporal modelling,state-space model,expectation-maximisation algorithm,spatially varying coefficients
AI 理解论文
溯源树
样例
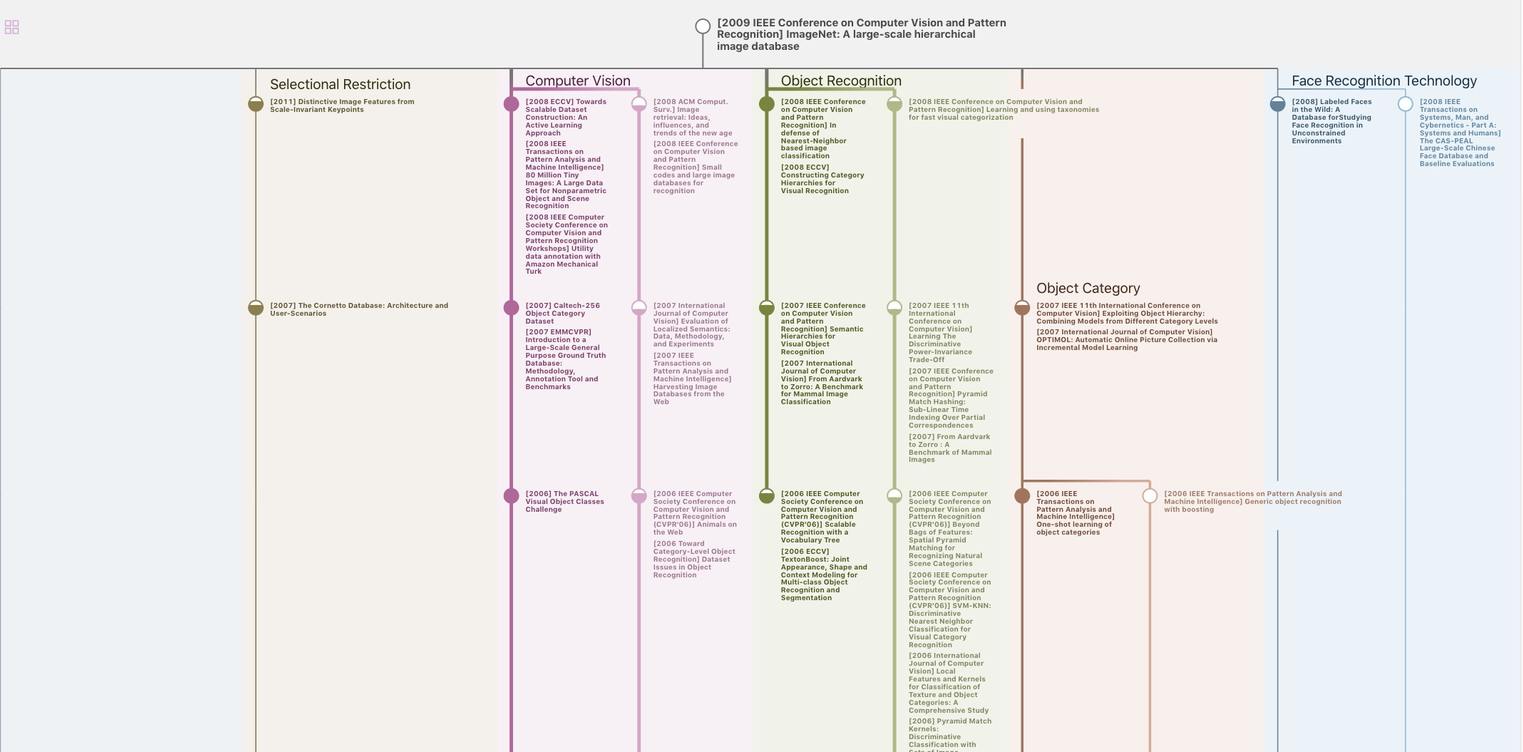
生成溯源树,研究论文发展脉络
Chat Paper
正在生成论文摘要