A hybrid framework based on extreme learning machine, discrete wavelet transform, and autoencoder with feature penalty for stock prediction
Expert Systems with Applications(2022)
摘要
Accurate prediction of the stock market trend can assist efficient portfolio and risk management. In recent years, with the rapid development of deep learning, it can make the classifiers more robust, which can be used for solving nonlinear problems. In our previous research, we proposed a numerical model for predicting the stock market via combination of discrete wavelet transform (DWT) denoising and extreme learning machine (ELM), and promising outcomes are achieved. The current research presents a hybrid framework using DWT, ELM, and autoencoder (AE) with feature penalty. Firstly, the backpropagation of the AE with feature penalty was deduced theoretically. Then, the raw data were denoised by DWT. The denoised data were used to train the AE with feature penalty after feature preprocessing and utilization of the labeling method. Afterward, the encoder part of the well-trained AE was utilized as the feature extraction model to train ELM model, and the hybrid framework named DAELM (DWT-AE-ELM) could be successfully developed. We also carried out experiments on the corresponding dataset of 400 stocks, and the prediction accuracy of the current study was higher than that of our previous research. According to the predicted labels, we presented an investment strategy, and the yield-to-maturity of 400 stocks was significantly higher than that of the buy-and-hold (BAH) strategy. The results confirmed the superiority of the proposed hybrid framework.
更多查看译文
关键词
Stock prediction,Deep learning,Extreme learning machine,Discrete wavelet transform,Autoencoder
AI 理解论文
溯源树
样例
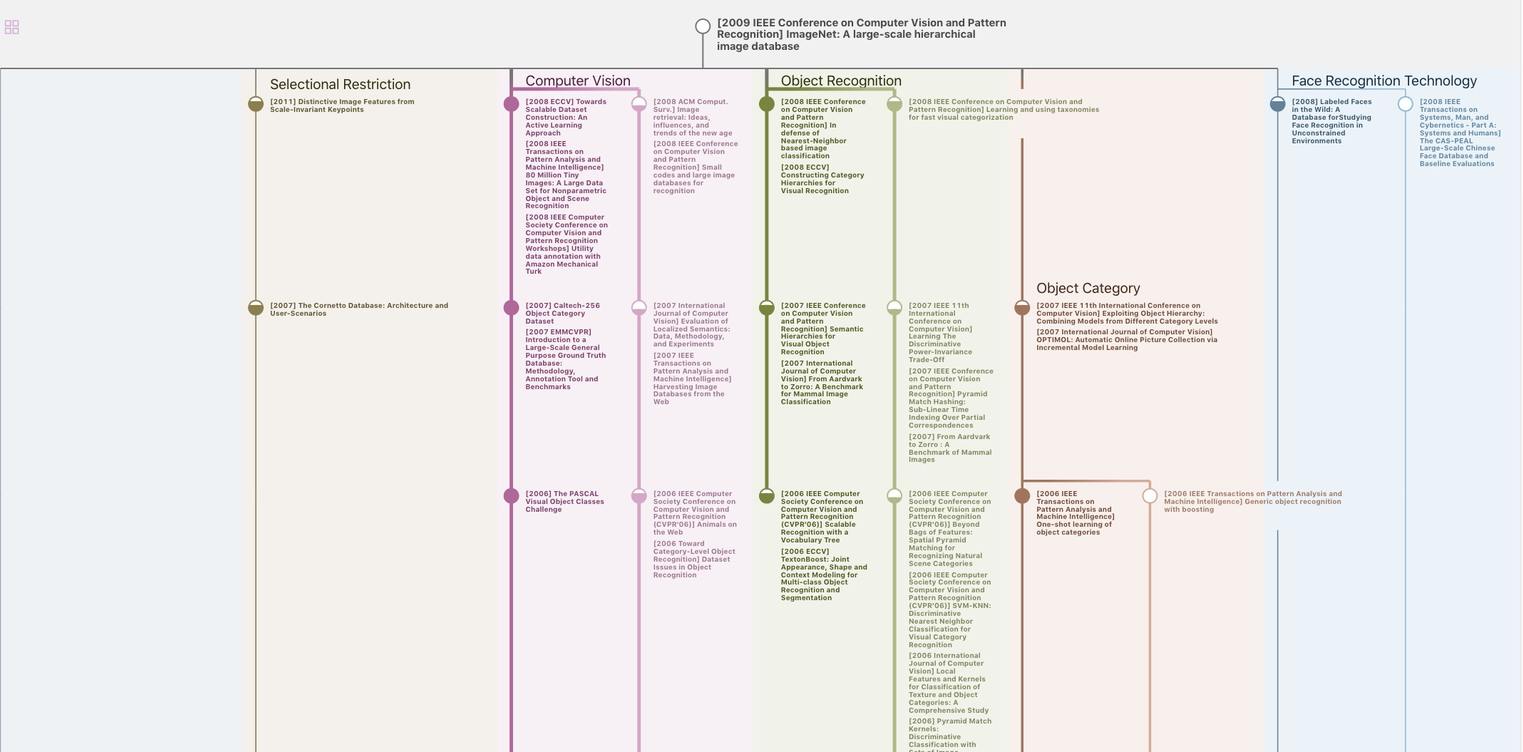
生成溯源树,研究论文发展脉络
Chat Paper
正在生成论文摘要