Human-inspired spatiotemporal feature extraction and fusion network for weather forecasting
Expert Systems with Applications(2022)
摘要
Reliable weather forecasting is quite important for sectors with weather-dependent decision-making. To capture unknown and complex dependencies from multiple spatiotemporal factors, i.e., autocorrelated, meteorological causal, meteorological spatial factors, this paper proposes a spatiotemporal feature extraction and fusion network inspired by humans’ multi-level cognition process, namely, SFEF-Net, including the data pre-processing stage, transfer pre-training stage, spatiotemporal feature extraction stage, and spatiotemporal feature fusion stage. Specifically, inspired by humans’ empirical analysis, the 24 Solar Terms knowledge and Pearson correlation coefficient analysis are utilized to respectively select input timestamps and influencing factors in the first stage. Inspired by humans’ associative learning, a Long Short-Term Memory Network (LSTM) layer with powerful remember capability is trained via weather data from multiple neighbored monitoring stations in the second stage, and further is transferred for better feature extraction. Inspired by humans’ independent analysis, every factor is separately captured and analyzed via the pre-trained LSTM layer in the third stage, which also coincides with the philosophical idea of divide and conquer. Inspired by humans’ comprehensive consideration, extracted features are fused level by level via the attention mechanism with dynamic weights in the fourth stage, which avoids invalid dependencies between multiple features and overfitting. The real-world daily average air temperature forecasting in Shaanxi Province, China is taken to demonstrate the SFEF-Net beyond 12 baseline methods. Additionally, forecasts of drastic change period, ablation analysis, multiple steps, and different stations further present the effectiveness of the SFEF-Net.
更多查看译文
关键词
Spatiotemporal systems,Weather forecast,Multi-level cognition process,Deep learning,Attention mechanism
AI 理解论文
溯源树
样例
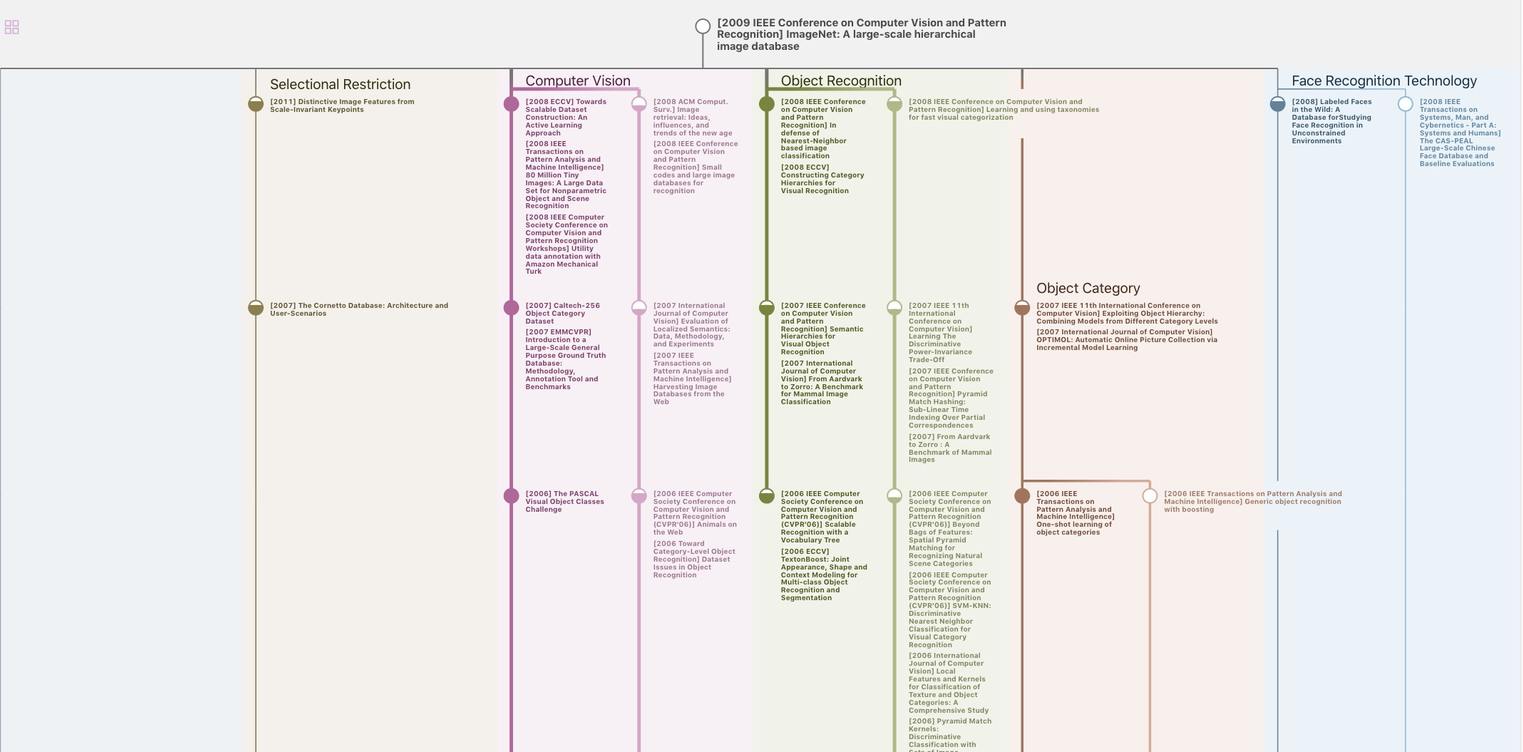
生成溯源树,研究论文发展脉络
Chat Paper
正在生成论文摘要