Early Prediction of Pressure Injury with Long Short-term Memory Networks
SENSORS AND MATERIALS(2022)
摘要
Early diagnosis of pressure injury has always been a challenging problem. Pressure injury can spontaneously heal or develop into decubitus ulcers. Few methods are available to predict the growth trend at the early stage of pressure injury, although this stage is a critical time for preventing and treating pressure injury. To address this issue, artificial intelligence algorithms were used in this work with image processing technology to predict the growth trend of early-stage pressure injury. A long short-term memory (LSTM) network, which is a specialized recurrent neural network, was adopted to predict future events based on images collected from hairless rats that made up the pressure injury models. The images were processed with ImageJ software to extract key features, then used to train the LSTM networks. Two types of LSTM network were used to predict the development trend: single-variate and multivariate. The analysis results demonstrated that multivariate LSTM is more effective than single-variate LSTM and has high potential to be applied in the prediction of early-stage pressure injury.
更多查看译文
关键词
early prediction, pressure injury, feature extraction, LSTM, neural networks
AI 理解论文
溯源树
样例
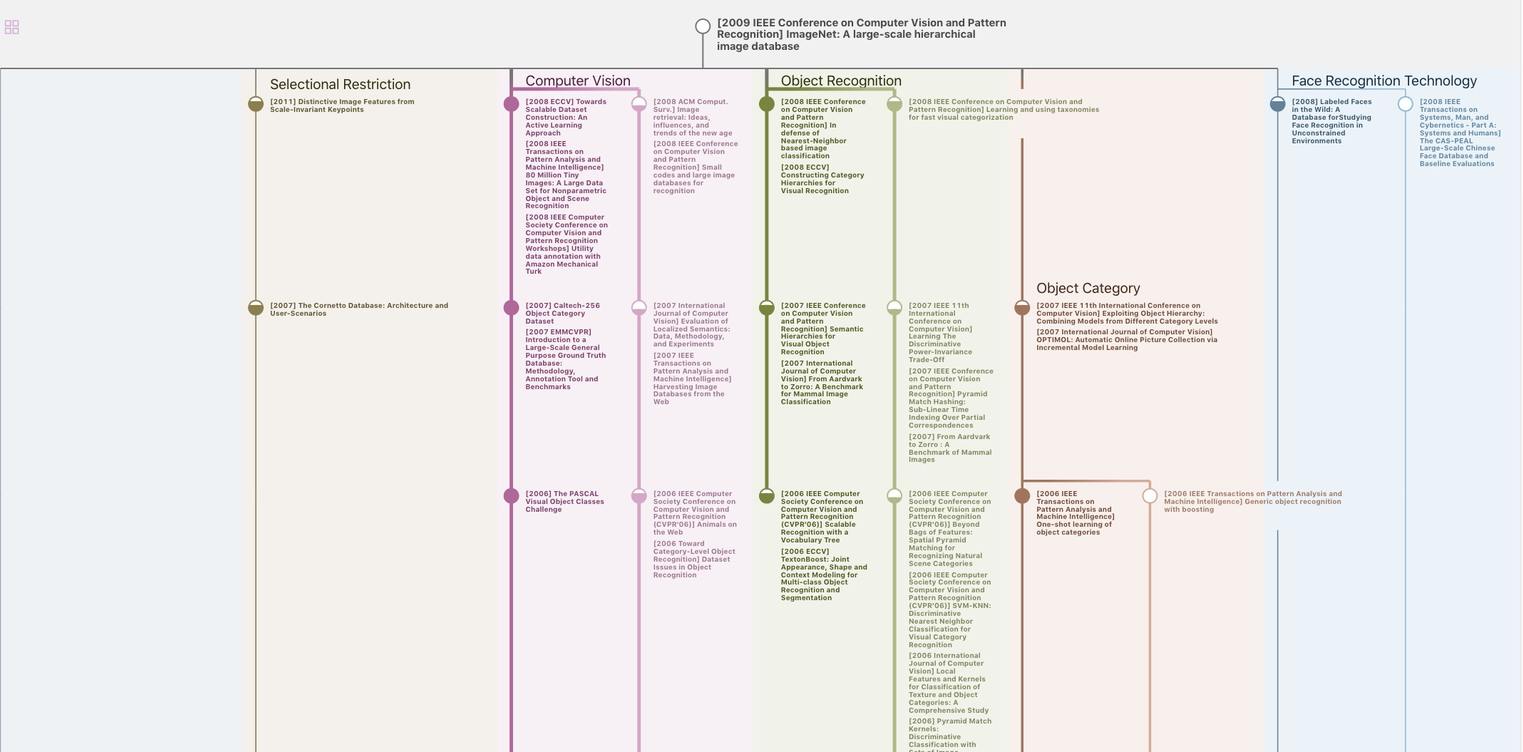
生成溯源树,研究论文发展脉络
Chat Paper
正在生成论文摘要