Ovarian cysts classification using novel deep reinforcement learning with Harris Hawks Optimization method
The Journal of Supercomputing(2022)
摘要
Ovaries are important parts of the female reproductive system because they produce the egg or ovum needed for fertilization. Cysts frequently impact female follicles, so torsion, infertility, and cancer can result from an enormous ovarian cyst. As a result, this is critical to get a diagnosis as quickly as feasible an ultrasound examination is performed to diagnose an ovarian cyst. So, this work gathered ultrasound images of different women's ovaries and determined whether or not an ovarian cyst was detected. This work proposed a novel technique to detect the ovarian cyst using images of ovarian ultrasound cysts from an ongoing database. Initially pre-processed by removing noise, followed by feature extraction, and finally classifying using new deep reinforcement learning with Harris Hawks Optimization (HHO) classifier. Automatic feature extraction is implemented using the recent popular convolutional neural network (CNN) technique that extracts image features as conditions in the reinforcement learning algorithm. Deep Q-Network (DQN) is generated to train a Q-network and detect the disease, and the swarm-based method of HHO utilizes the optimization method to produce optimal hyperparameters in the DQN model known as HHO-DQN. Extensive experimental evaluations on datasets show that the proposed HHO- DQN approach outperforms existing active learning approaches for ovarian cyst classification.
更多查看译文
关键词
Active learning, Deep reinforcement learning, Deep learning, Image classification, Ultrasound medical image, Ovarian cyst classification and medical imaging
AI 理解论文
溯源树
样例
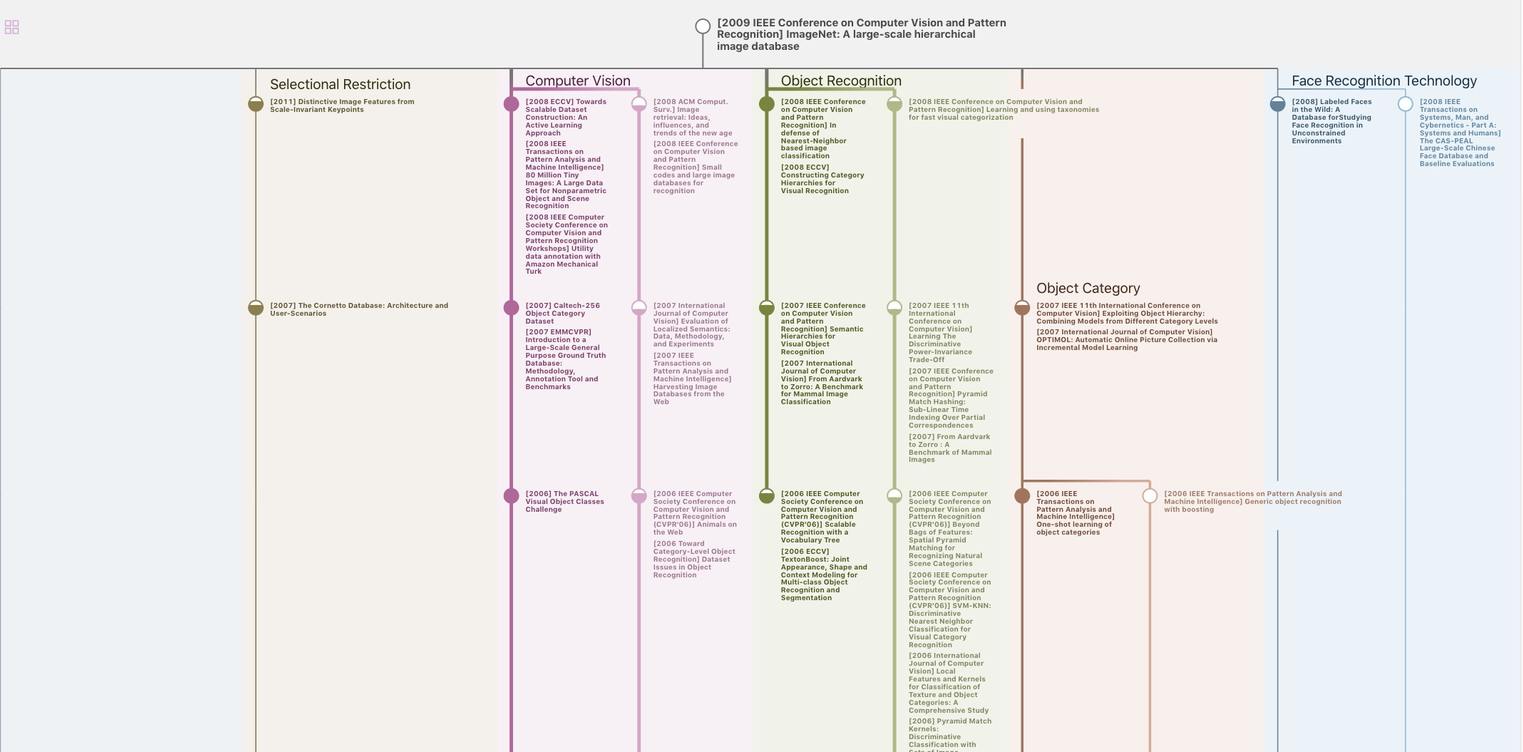
生成溯源树,研究论文发展脉络
Chat Paper
正在生成论文摘要