EcoForecast: An interpretable data-driven approach for short-term macroeconomic forecasting using N-BEATS neural network
Engineering Applications of Artificial Intelligence(2022)
摘要
It will be beneficial to devise an effective approach for short-term macroeconomic forecasting. Existing traditional statistics-based macroeconomic forecasting mainly focuses on exploring feasible methods for improving the accuracy of long-term predictions. However, the performance of short-term predictions was far less impressive under the impact of unexpected incidents. Furthermore, some deep learning-based approaches can achieve fine-grained variable frequency forecasting and preliminarily demonstrate effective results, but the interpretability is still controversial. Therefore, how to consider both the performance and the interpretability has already become a universal concern and an urgent unsolved problem. In this paper, we identified the above issue and proposed an interpretable data-driven approach, named EcoForecast11The code and data are available at https://github.com/navfour/ecoforecast., for short-term macroeconomic forecasting based on the N-BEATS (neural basis expansion analysis for interpretable time series forecasting) neural network. To the best of our knowledge, EcoForecast is the first interpretable purely data-driven unified normative scheme for macroeconomic forecasting that achieves variable forecast frequencies and prediction domains, surpassing traditional statistics-based and deep learning-based approaches in performance or interpretability.
更多查看译文
关键词
Macroeconomic forecasting,Deep learning,N-BEATS,BVAR
AI 理解论文
溯源树
样例
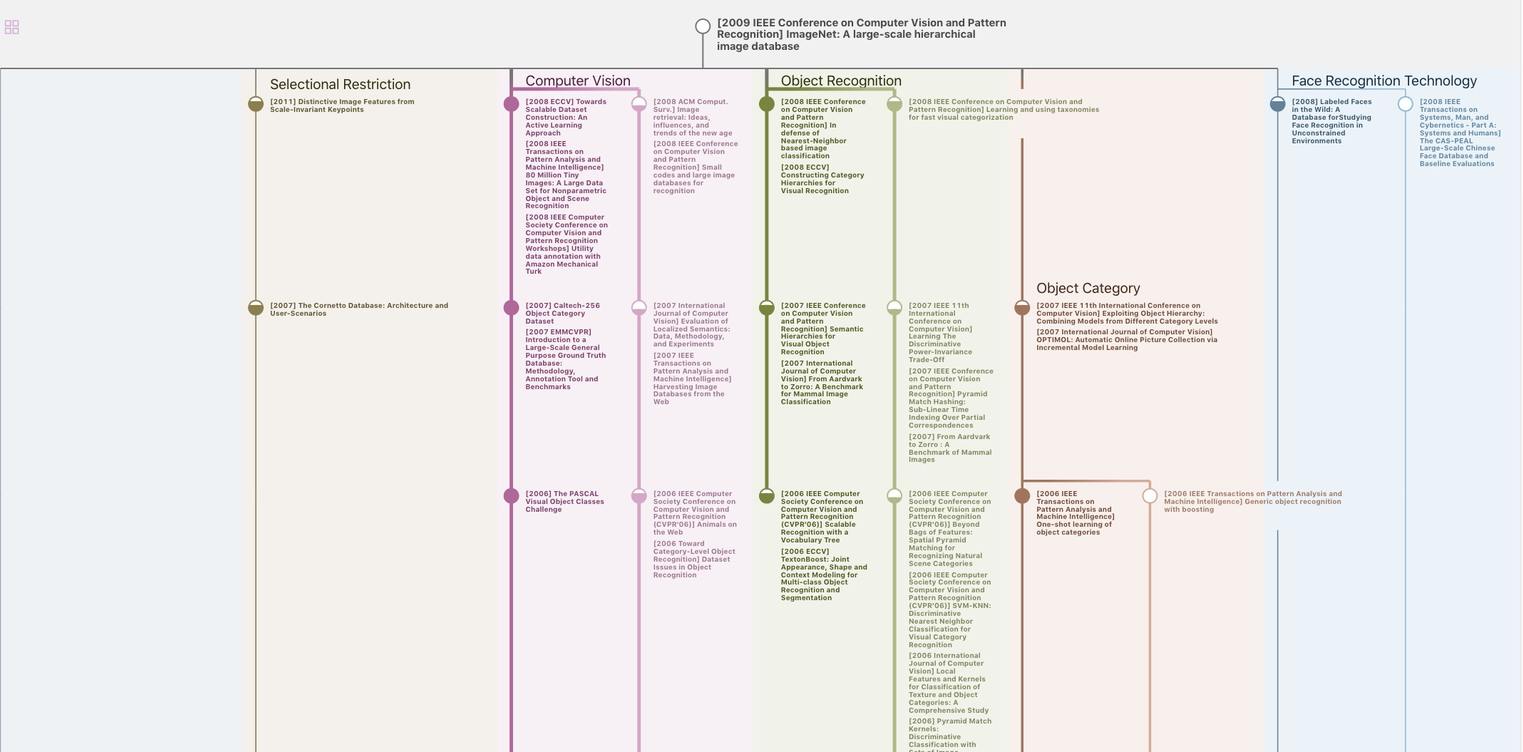
生成溯源树,研究论文发展脉络
Chat Paper
正在生成论文摘要